How can we quantify and reduce the uncertainty of a watershed-scale pesticide transfer model? A comparison of several approaches
HAL (Le Centre pour la Communication Scientifique Directe)(2021)
摘要
Intensive use of pesticides in agricultural catchments leads to a widespread contamination of rivers and groundwater. Pesticide applied on fields are transfered at surface and in subsurface to waterbodies. Such transfers are highly influenced by landscape elements that can accelerate or slow down and dissipate water and contaminant flows. The PESHMELBA model has been developed to simulate pesticide fate on small agricultural catchments and to represent the landscape elements in an explicit way. It is characterized by a process-oriented approach and an original spatial discretization that make it particularly suitable to simulate complex agricultural catchments. In the long run, we aim at setting up and comparing different landscape organization scenarios for decision-making support. However, before considering such operationnal use of PESHMELBA, it must be strongly evaluated and uncertainties must be quantified and reduced. We firstly set a small virtual hillslope composed of plots, vegetative filter zones and river reaches. Even basic, this configuration led to a large set of parameters as the model is fully distributed and physically-based. Whats more, the modular structure of PESHMELBA, composed of independant building blocks coupled within a framework resulted in a highly non linear model. An adapted strategy had to be settled to address such challenges. First, a sensitivity analysis was applied using several fast methods (Morris, Hilbert-Schmidt Independence Criterion HSIC, Polynomial Chaos Extension PCE), to identify sensitive parameters , allowing to, secondly, a Sobol procedure on less input factors. Preliminary results improved the understanding we have about the model functioning. Some guidelines about critical representation of processes or potential simplifications also arose. Above all, this study is a preliminary step to choose variables to be potentially assimilated and a suitable data assimilation method to reduce the models uncertainty.
更多查看译文
关键词
watershed-scale watershed-scale
AI 理解论文
溯源树
样例
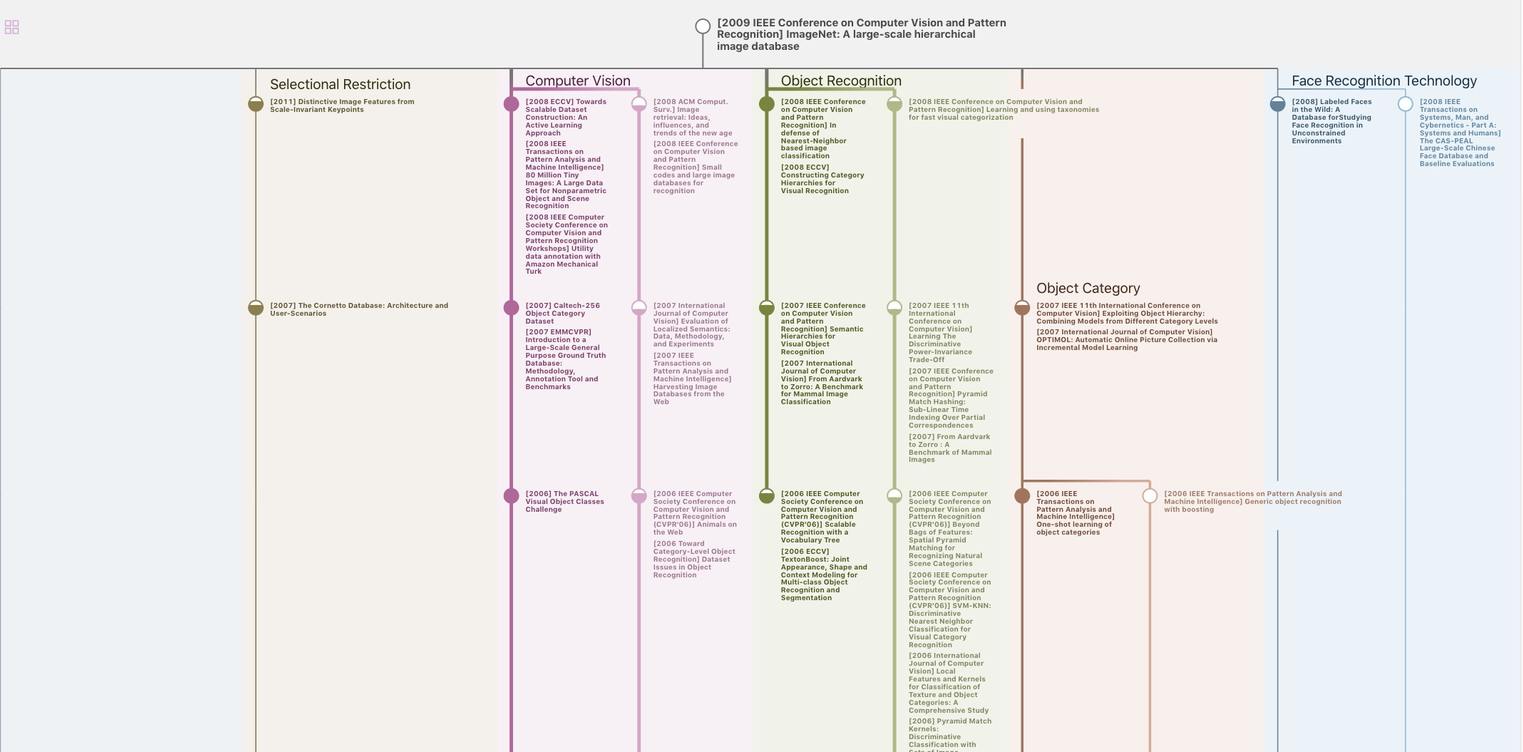
生成溯源树,研究论文发展脉络
Chat Paper
正在生成论文摘要