Energy Extrapolation in Quantum Optimization Algorithms
arXiv (Cornell University)(2021)
摘要
Quantum optimization algorithms are promising to find the ground state of a target Hamiltonian on near-term quantum devices. However, it is necessary to limit the evolution time or the circuit depth as much as possible since otherwise decoherence will degrade the computation. Even when this is done, there always exists a non-negligible estimation error in the ground state energy. Here we show a scalable extrapolation approach to mitigate this error. With an appropriate regression, we can significantly improve the estimation accuracy for quantum annealing, variational quantum eigensolver and quantum imaginary time evolution for fixed evolution time or circuit depth. The inference is achieved by extrapolating the annealing time to infinity or extrapolating the variance to zero. For Hamiltonians that only involve few-body interactions, the additional overhead is an increase in the number of measurements by a constant factor. We verified the validity of our method via numerical simulation and experiments on an IBM quantum computer. The method is robust to noise, and the techniques are applicable to other physics problems. Analytic derivations for the quadratic convergence feature of the residual energy in quantum annealing and the linear convergence feature of energy variance are given. Our work paves a promising new way for enhancing near-term quantum computing with classical post-processing.
更多查看译文
关键词
quantum,optimization,algorithms,energy
AI 理解论文
溯源树
样例
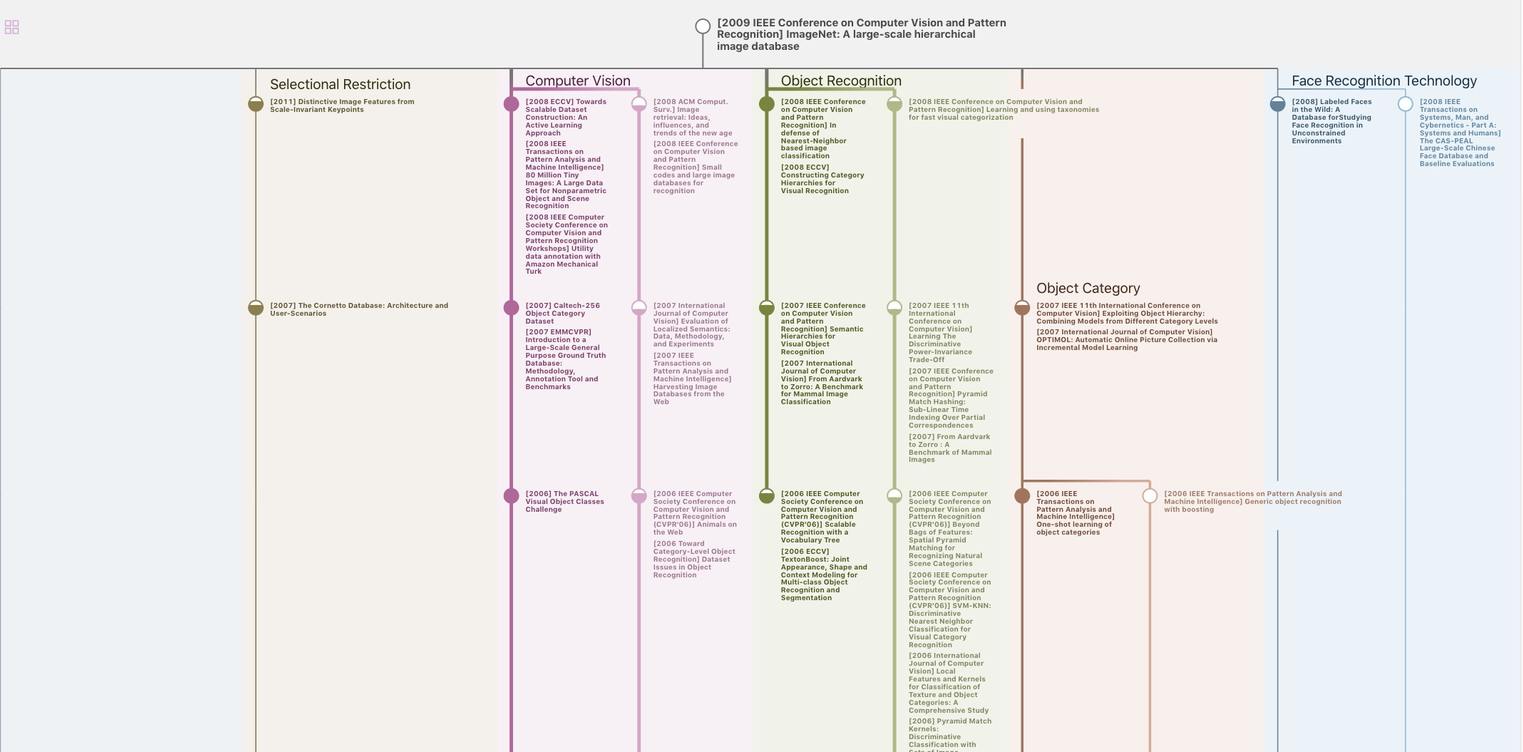
生成溯源树,研究论文发展脉络
Chat Paper
正在生成论文摘要