Auto4D: Learning to Label 4D Objects from Sequential Point Clouds
arXiv (Cornell University)(2021)
摘要
In the past few years we have seen great advances in object perception (particularly in 4D space-time dimensions) thanks to deep learning methods. However, they typically rely on large amounts of high-quality labels to achieve good performance, which often require time-consuming and expensive work by human annotators. To address this we propose an automatic annotation pipeline that generates accurate object trajectories in 3D space (i.e., 4D labels) from LiDAR point clouds. The key idea is to decompose the 4D object label into two parts: the object size in 3D that's fixed through time for rigid objects, and the motion path describing the evolution of the object's pose through time. Instead of generating a series of labels in one shot, we adopt an iterative refinement process where online generated object detections are tracked through time as the initialization. Given the cheap but noisy input, our model produces higher quality 4D labels by re-estimating the object size and smoothing the motion path, where the improvement is achieved by exploiting aggregated observations and motion cues over the entire trajectory. We validate the proposed method on a large-scale driving dataset and show a 25% reduction of human annotation efforts. We also showcase the benefits of our approach in the annotator-in-the-loop setting.
更多查看译文
关键词
label auto4d objects,sequential point clouds,learning
AI 理解论文
溯源树
样例
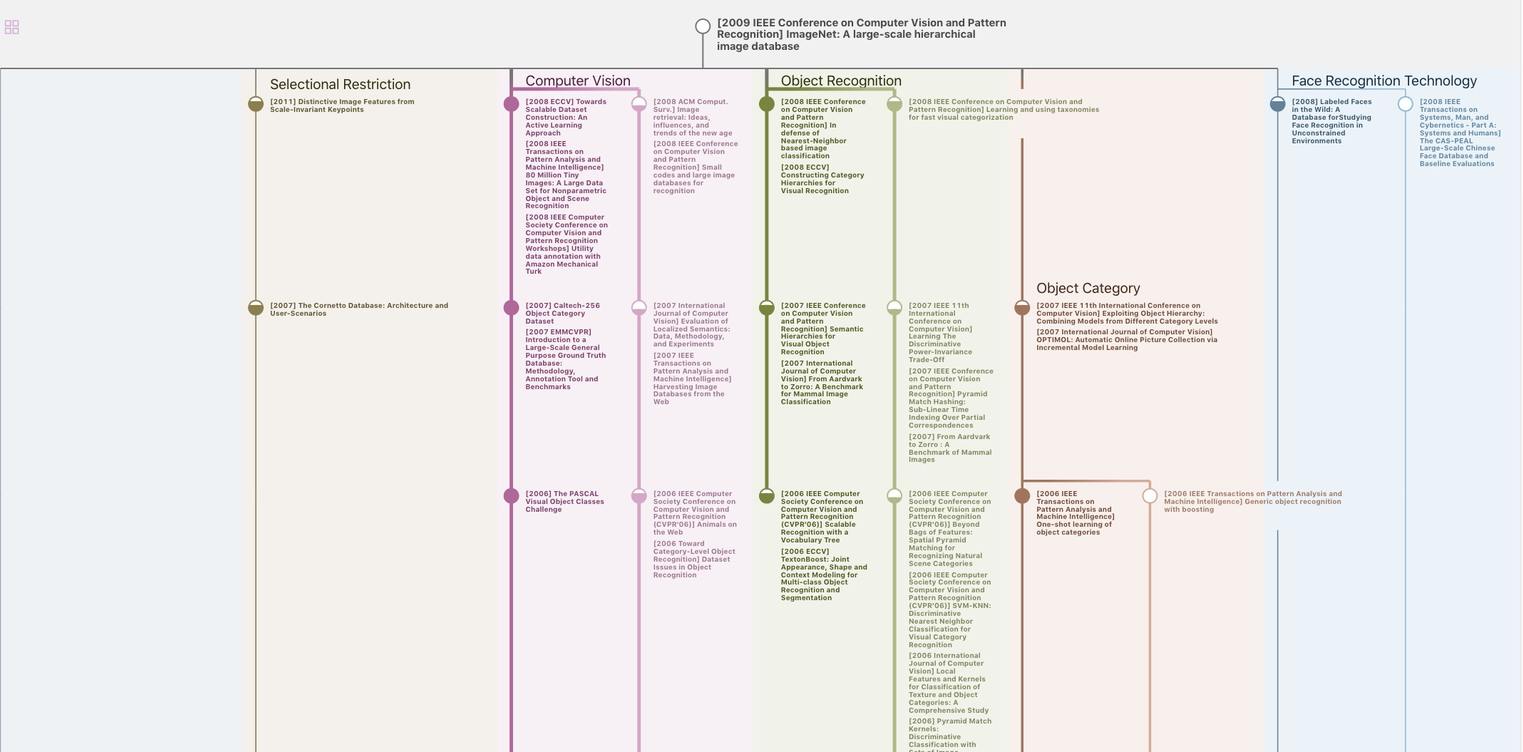
生成溯源树,研究论文发展脉络
Chat Paper
正在生成论文摘要