Calibrating and Improving Graph Contrastive Learning
arXiv (Cornell University)(2021)
摘要
Graph contrastive learning algorithms have demonstrated remarkable success in various applications such as node classification, link prediction, and graph clustering. However, in unsupervised graph contrastive learning, some contrastive pairs may contradict the truths in downstream tasks and thus the decrease of losses on these pairs undesirably harms the performance in the downstream tasks. To assess the discrepancy between the prediction and the ground-truth in the downstream tasks for these contrastive pairs, we adapt the expected calibration error (ECE) to graph contrastive learning. The analysis of ECE motivates us to propose a novel regularization method, Contrast-Reg, to ensure that decreasing the contrastive loss leads to better performance in the downstream tasks. As a plug-in regularizer, Contrast-Reg effectively improves the performance of existing graph contrastive learning algorithms. We provide both theoretical and empirical results to demonstrate the effectiveness of Contrast-Reg in enhancing the generalizability of the Graph Neural Network(GNN) model and improving the performance of graph contrastive algorithms with different similarity definitions and encoder backbones across various downstream tasks.
更多查看译文
关键词
graph contrastive learning,calibrating
AI 理解论文
溯源树
样例
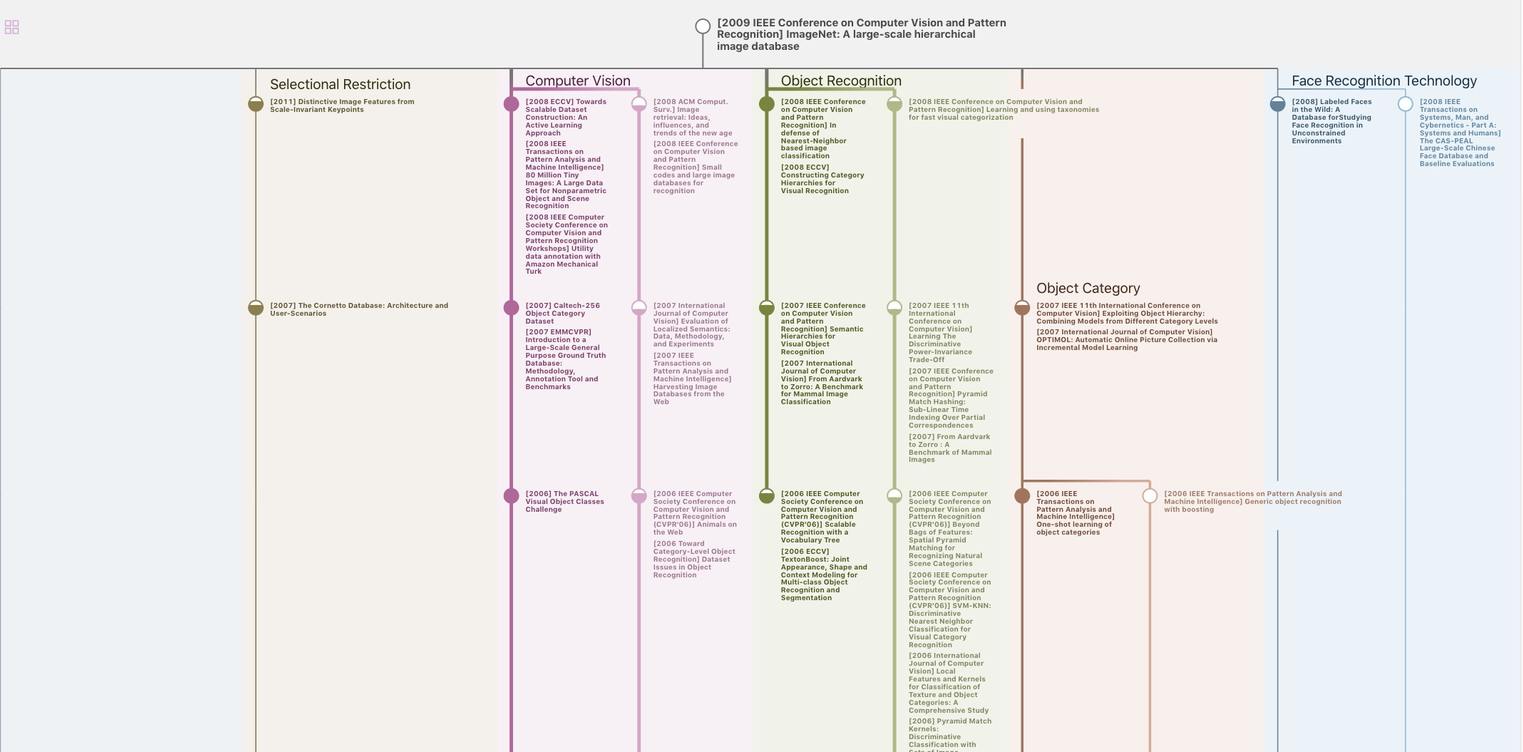
生成溯源树,研究论文发展脉络
Chat Paper
正在生成论文摘要