Inference on the Change Point for High Dimensional Dynamic Graphical Models
arXiv (Cornell University)(2020)
摘要
We develop an estimator for the change point parameter for a dynamically evolving graphical model, and also obtain its asymptotic distribution under high dimensional scaling. To procure the latter result, we establish that the proposed estimator exhibits an $O_p(\psi^{-2})$ rate of convergence, wherein $\psi$ represents the jump size between the graphical model parameters before and after the change point. Further, it retains sufficient adaptivity against plug-in estimates of the graphical model parameters. We characterize the forms of the asymptotic distribution under the both a vanishing and a non-vanishing regime of the magnitude of the jump size. Specifically, in the former case it corresponds to the argmax of a negative drift asymmetric two sided Brownian motion, while in the latter case to the argmax of a negative drift asymmetric two sided random walk, whose increments depend on the distribution of the graphical model. Easy to implement algorithms are provided for estimating the change point and their performance assessed on synthetic data. The proposed methodology is further illustrated on RNA-sequenced microbiome data and their changes between young and older individuals.
更多查看译文
关键词
change point,inference
AI 理解论文
溯源树
样例
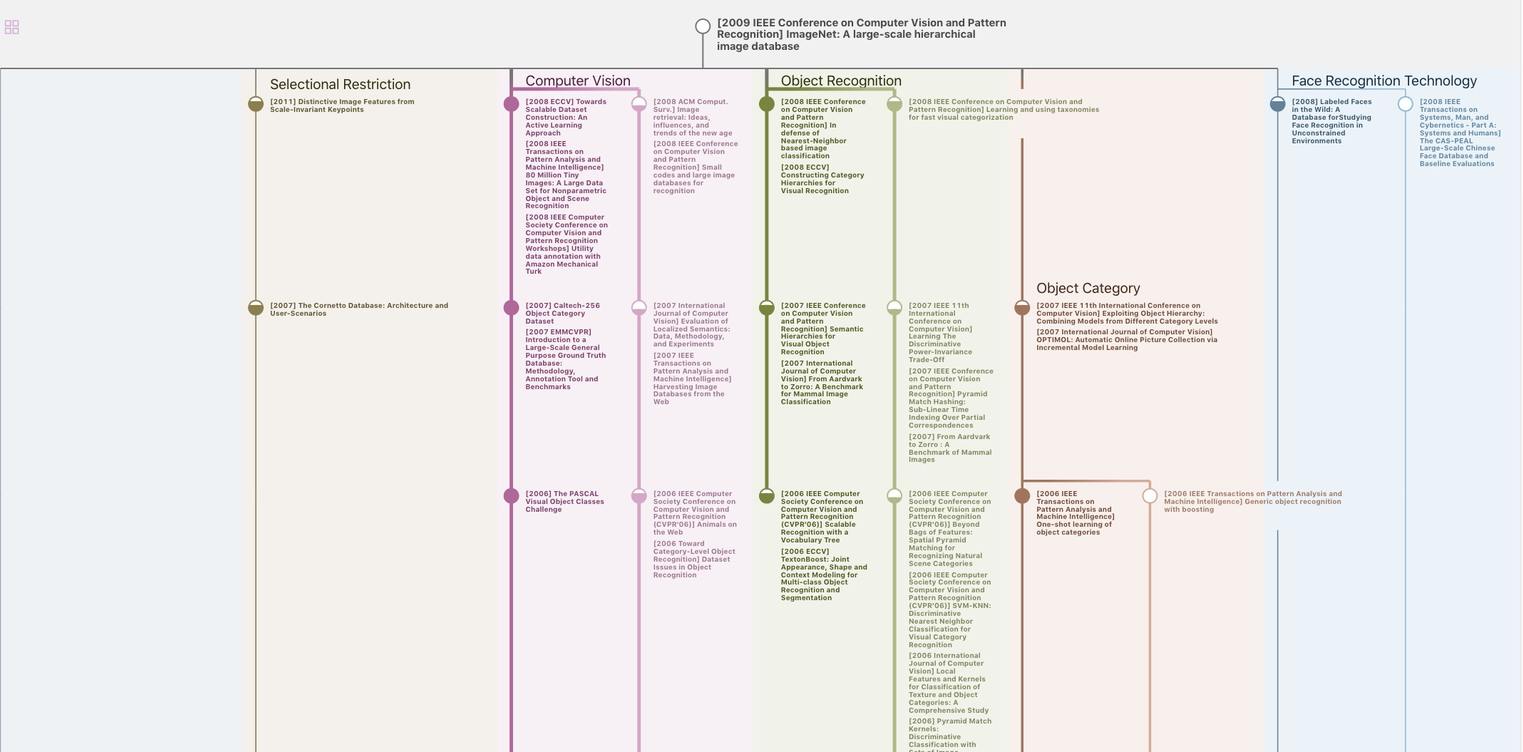
生成溯源树,研究论文发展脉络
Chat Paper
正在生成论文摘要