Additive interaction modelling using I-priors
arXiv (Cornell University)(2020)
摘要
Additive regression models with interactions are widely studied in the literature, using methods such as splines or Gaussian process regression. However, these methods can pose challenges for estimation and model selection, due to the presence of many smoothing parameters and the lack of suitable criteria. We propose to address these challenges by extending the I-prior methodology (Bergsma, 2020) to multiple covariates, which may be multidimensional. The I-prior methodology has some advantages over other methods, such as Gaussian process regression and Tikhonov regularization, both theoretically and practically. In particular, the I-prior is a proper prior, is based on minimal assumptions, yields an admissible posterior mean, and estimation of the scale (or smoothing) parameters can be done using an EM algorithm with simple E and M steps. Moreover, we introduce a parsimonious specification of models with interactions, which has two benefits: (i) it reduces the number of scale parameters and thus facilitates the estimation of models with interactions, and (ii) it enables straightforward model selection (among models with different interactions) based on the marginal likelihood.
更多查看译文
关键词
additive interaction modelling,i-priors
AI 理解论文
溯源树
样例
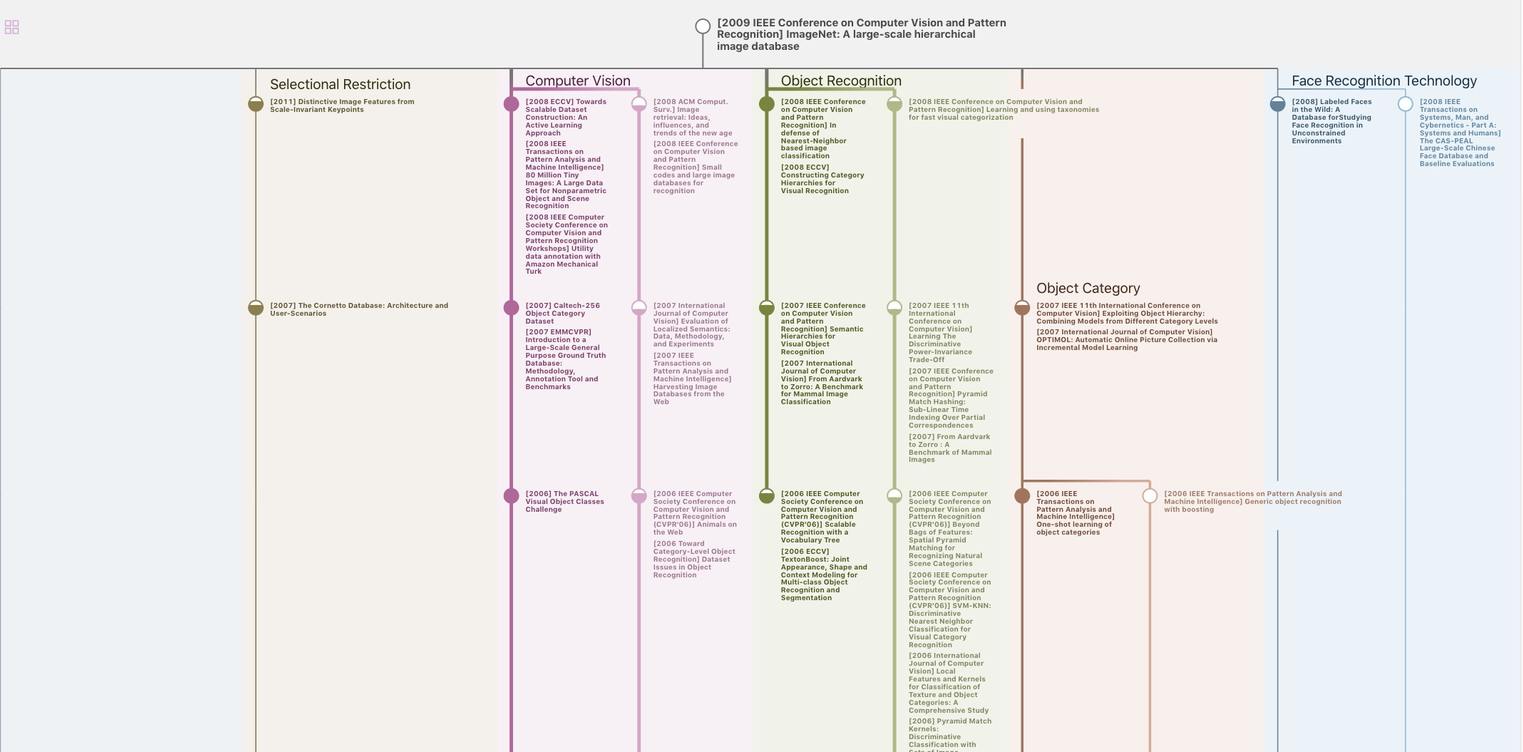
生成溯源树,研究论文发展脉络
Chat Paper
正在生成论文摘要