Development and External Evaluation of Predictions Models for Mortality of COVID-19 Patients using Machine Learning Method
Research Square (Research Square)(2020)
摘要
Abstract Background To develop and evaluate the prognostic machine-learning model for mortality in patients with coronavirus disease 2019 (COVID-19).Methods Clinical data of confirmed COVID-19 were retrospectively collected from Wuhan between 18th January and 29th March 2020. Gradient Boosting Decision Tree (GBDT), logistic regression (LR) model, and simplified LR with selected 5 features (LR-5) model were built to predict the mortality of COVID-19. 5-fold area under curve (AUC), accuracy, positive predictive value (PPV), and negative predictive value (NPV) were calculated and compared between models.Results A total of 2,924 patients were included in the final analysis, 257(8.8%) of whom died during hospitalization and 2,667 (91.2%) survived. There were 21(0.7%) mild cases, 2,051(70.1%) moderate case, 779(26.6%) severe cases, and 73(2.5%) critically severe cases of COVID-19 on admission. The overall 5-fold AUC was observed highest in GBDT model (0.941), followed by LR (0.928) and LR-5 (0.913). The diagnostic accuracy were 0.889 in GBDT, 0.868 in LR and 0.887 in LR-5. GBDT model also showed the highest sensitivity (0.899) and speciality (0.889). The NPV of all three models exceeded 97%, while the PPV were relatively low in all models, 0.381 for LR, 0.402 for LR-5 and 0.432 for GBDT. In subgroups analysis with severe cases only, GBDT model also performed the best with a accuracy of 0.799 and 5-fold AUC (0.918).Conclusion The finding revealed that mortality prediction performance of the GBDT was superior to the LR models in confirmed cases of COVID-19, regardless of disease severity.
更多查看译文
关键词
mortality,predictions models,machine learning
AI 理解论文
溯源树
样例
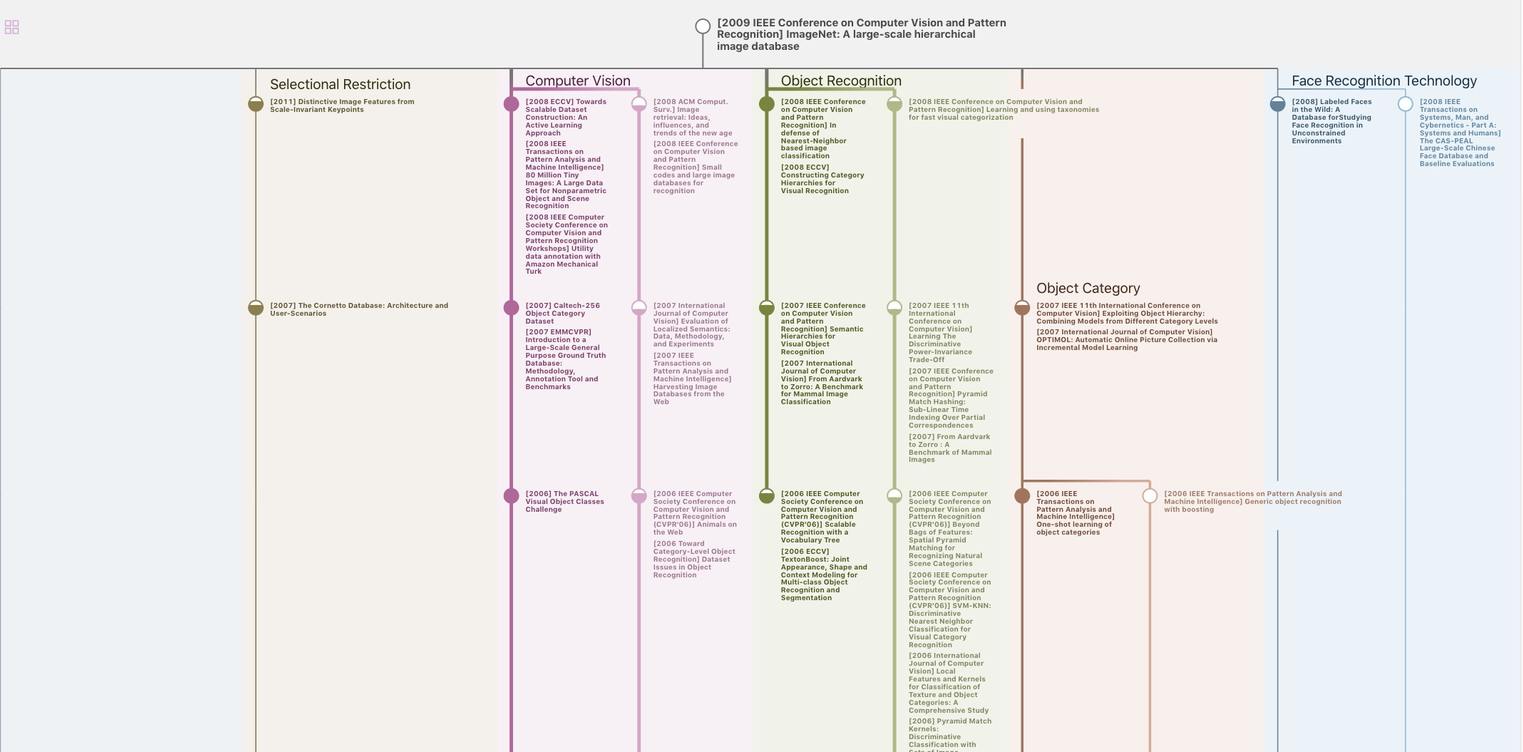
生成溯源树,研究论文发展脉络
Chat Paper
正在生成论文摘要