Distinct predictive patterns of antidepressant response to sertraline, placebo and bupropion using pre-treatment reward processing as examined through functional MRI and deep learning: Key results from the EMBARC study
bioRxiv (Cold Spring Harbor Laboratory)(2020)
摘要
ABSTRACT Uncertainty in selecting the appropriate antidepressant for each patient is a major challenge in treatment of major depressive disorder (MDD). No biologically driven markers are currently available to improve precision in treatment selection, thus leading to a trial-and-error process and prolonged morbidity for most patients. This study developed deep learning models that accurately predict treatment outcomes for sertraline, bupropion and placebo. Models were trained on data from the EMBARC study, in which 223 un-medicated subjects with MDD underwent pre-treatment reward task fMRI and received 8 weeks of treatment with sertraline, bupropion, or placebo. These models integrate fMRI and clinical measures and they explain up to 37% of the variance in ΔHAMD, classify remitters with NNT of 2.3-4.3, and classify responders with NNT of 3.2-4.9. Findings reveal new regions predictive of treatment outcome such as the hippocampus and paracentral lobule, while additional regions implicated in existing research are corroborated. Distinct models were identified for each treatment and provide substantial evidence of their potential to improve precision in treatment selection for MDD.
更多查看译文
关键词
antidepressant response,sertraline,functional mri,embarc study,placebo,pre-treatment
AI 理解论文
溯源树
样例
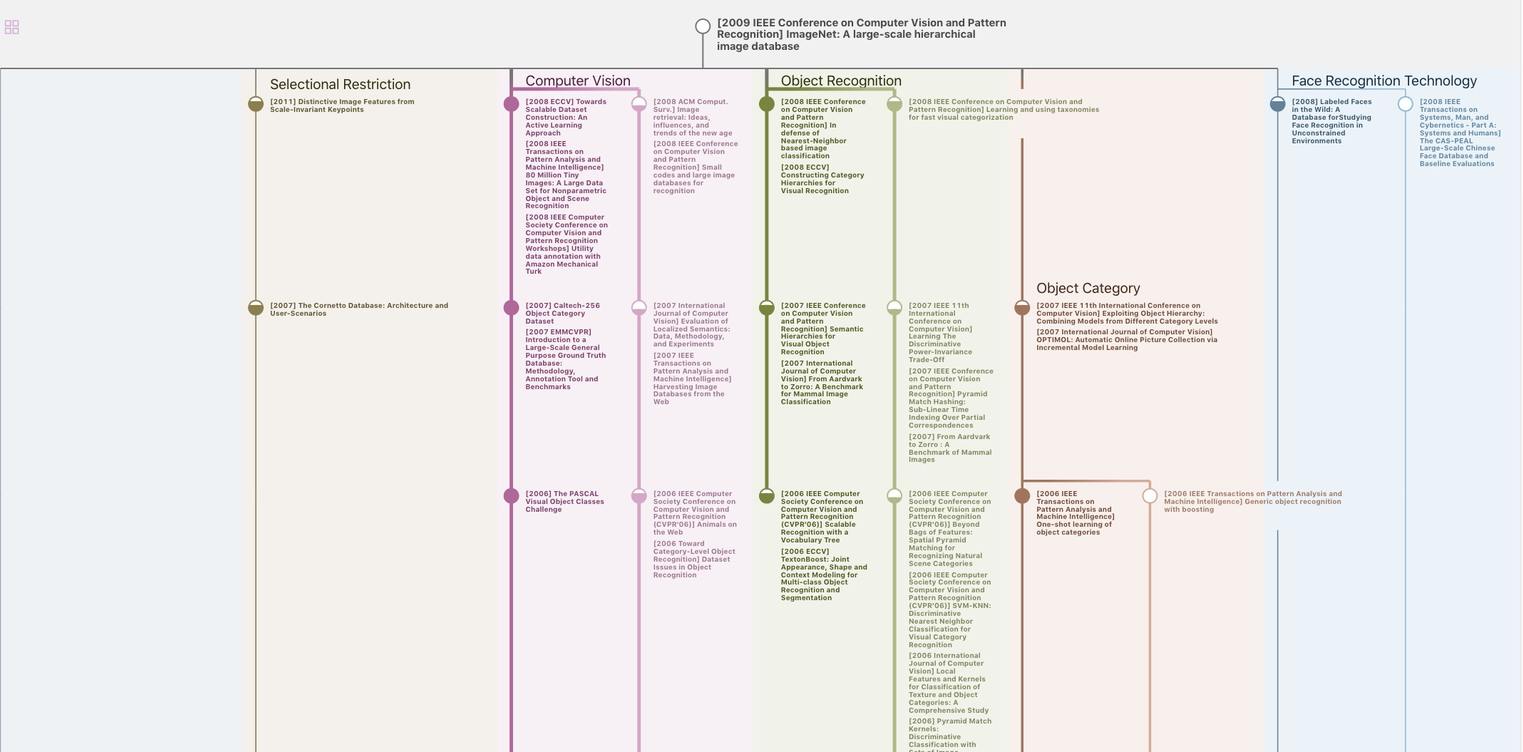
生成溯源树,研究论文发展脉络
Chat Paper
正在生成论文摘要