Efficiently Enhancing Zero-Shot Performance of Instruction Following Model via Retrieval of Soft Prompt.
EMNLP 2023(2023)
摘要
Enhancing the zero-shot performance of instruction-following models requires heavy computation, either by scaling the total number of training datasets or the model size. In this work, we explore how retrieval of soft prompts obtained through prompt tuning can efficiently assist hard prompts in zero-shot task generalization. Specifically, we train soft prompt embeddings for each prompt through prompt tuning, store the samples of the training instances mapped with the prompt embeddings, and retrieve the corresponding prompt embedding of the training instance closest to the query instance during inference. While only adding 0.007% additional parameters, retrieval of soft prompt enhances the performance of T0 on unseen tasks by outperforming it on 10 out of 11 datasets as well as improving the mean accuracy of T0 on BIG-bench benchmark by 2.39% points. Also, we report an interesting finding that retrieving source embeddings trained on similar answer choice formats is more important than those on similar task types.
更多查看译文
关键词
instruction following
AI 理解论文
溯源树
样例
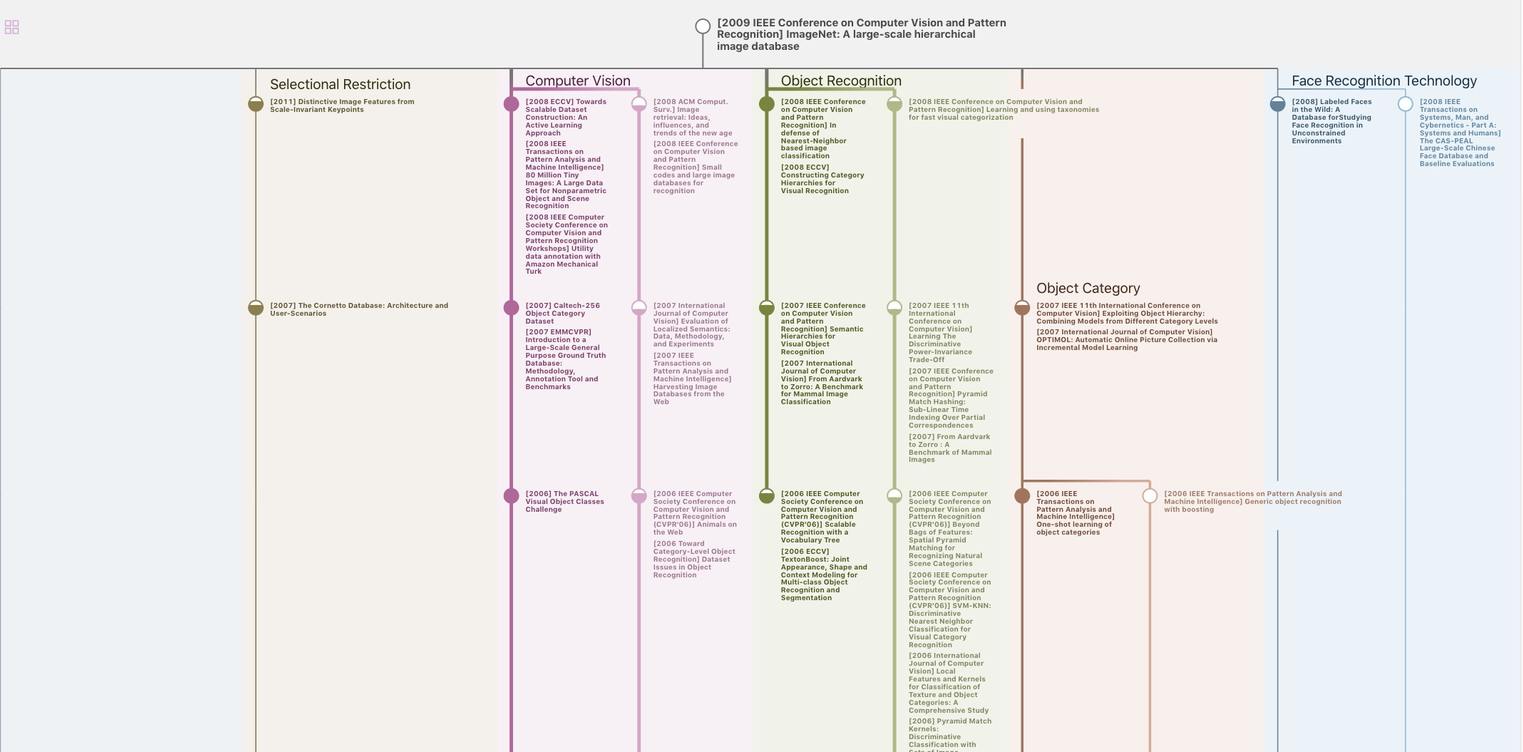
生成溯源树,研究论文发展脉络
Chat Paper
正在生成论文摘要