Learning Diverse Options via InfoMax Termination Critic
arXiv (Cornell University)(2020)
摘要
We consider the problem of autonomously learning reusable temporally extended actions, or options, in reinforcement learning. While options can speed up transfer learning by serving as reusable building blocks, learning reusable options for unknown task distribution remains challenging. Motivated by the recent success of mutual information (MI) based skill learning, we hypothesize that more diverse options are more reusable. To this end, we propose a method for learning termination conditions of options by maximizing MI between options and corresponding state transitions. We derive a scalable approximation of this MI maximization via gradient ascent, yielding the InfoMax Termination Critic (IMTC) algorithm. Our experiments demonstrate that IMTC significantly improves the diversity of learned options without extrinsic rewards combined with an intrinsic option learning method. Moreover, we test the reusability of learned options by transferring options into various tasks, confirming that IMTC helps quick adaptation, especially in complex domains where an agent needs to manipulate objects.
更多查看译文
关键词
infomax termination critic,diverse options,learning
AI 理解论文
溯源树
样例
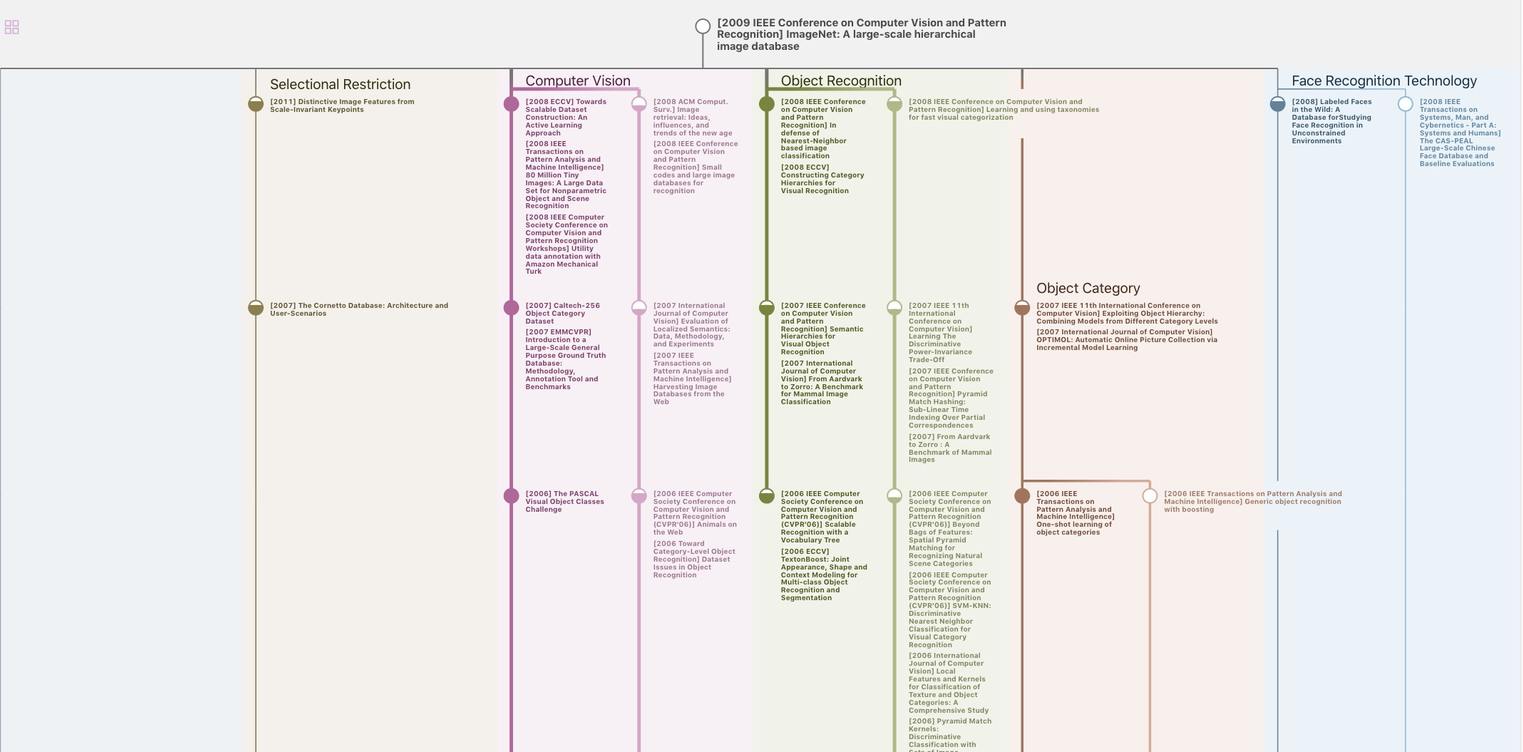
生成溯源树,研究论文发展脉络
Chat Paper
正在生成论文摘要