Analysis on Effects of Fault Elements in Memristive Neuromorphic Systems
CoRR(2023)
摘要
Nowadays, neuromorphic systems based on Spiking Neural Networks (SNNs)
attract attentions of many researchers. There are many studies to improve
performances of neuromorphic systems. These studies have been showing
satisfactory results. To magnify performances of neuromorphic systems,
developing actual neuromorphic systems is essential. For developing them,
memristors play key role due to their useful characteristics. Although
memristors are essential for actual neuromorphic systems, they are vulnerable
to faults. However, there are few studies analyzing effects of fault elements
in neuromorphic systems using memristors. To solve this problem, we analyze
performance of a memristive neuromorphic system with fault elements changing
fault ratios, types, and positions. We choose neurons and synapses to inject
faults. We inject two types of faults to synapses: SA0 and SA1 faults. The
fault synapses appear in random and important positions. Through our analysis,
we discover the following four interesting points. First, memristive
characteristics increase vulnerability of neuromorphic systems to fault
elements. Second, fault neuron ratios reducing performance sharply exist.
Third, performance degradation by fault synapses depends on fault types.
Finally, SA1 fault synapses improve performance when they appear in important
positions.
更多查看译文
AI 理解论文
溯源树
样例
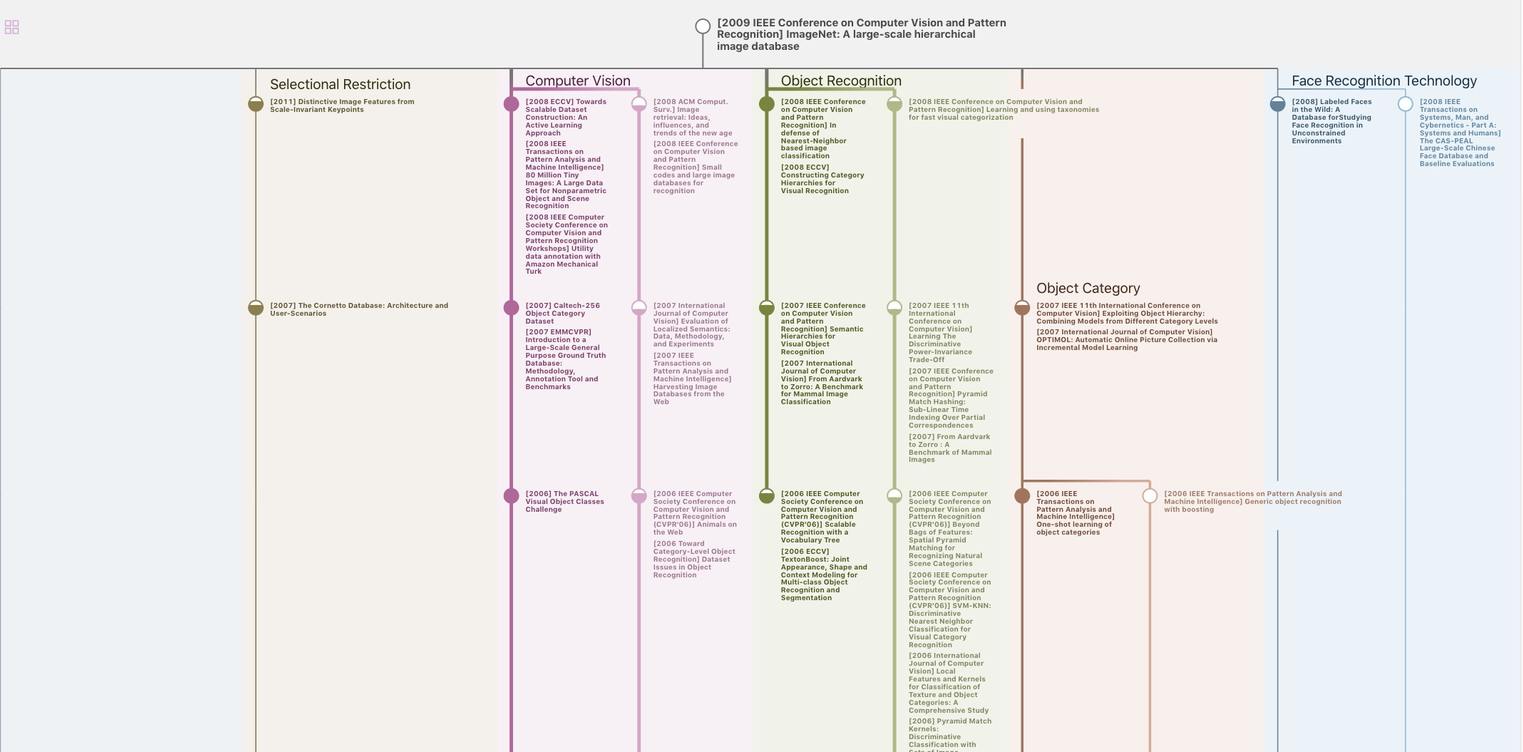
生成溯源树,研究论文发展脉络
Chat Paper
正在生成论文摘要