A deep learning-based high-temperature overtime working alert system for smart cities with multi-sensor data
NONDESTRUCTIVE TESTING AND EVALUATION(2024)
摘要
Prolonged heat exposure may cause various physiological responses to outdoor workers. This will result in economic and productivity losses for a company and also may affect the sustainable development speed of cities. To avoid the above adverse effects, an alerting system is designed for outdoor workers to prevent them from overtime working in high-temperature scenarios. In the system, multiple sensors embedded micro-electromechanical system (MEMS) wearable device is used for real-time working status data collection, and a hybrid deep learning model is adopted to recognise the working status of outdoor workers. This hybrid model, called C-LSTM, combines the advantages of convolutional neural networks (CNN) and long short-term memory networks (LSTM) to extract useful spatial and temporal features of working status efficiently. Experimental results show that the performance on the inference time and accuracy of the C-LSTM model is better than that of conventional ones. The working status recognition accuracy of the C-LSTM model reaches 97.91%, and the inference time of the model reduces to less than 51 ms. In addition, the C-LSTM model has the best stability. The designed system can not only be used in outdoor high-temperature environment but also applied to medical and industrial scenarios, which further extends the application fields.
更多查看译文
关键词
Working status,sensor,deep learning,sustainable smart city
AI 理解论文
溯源树
样例
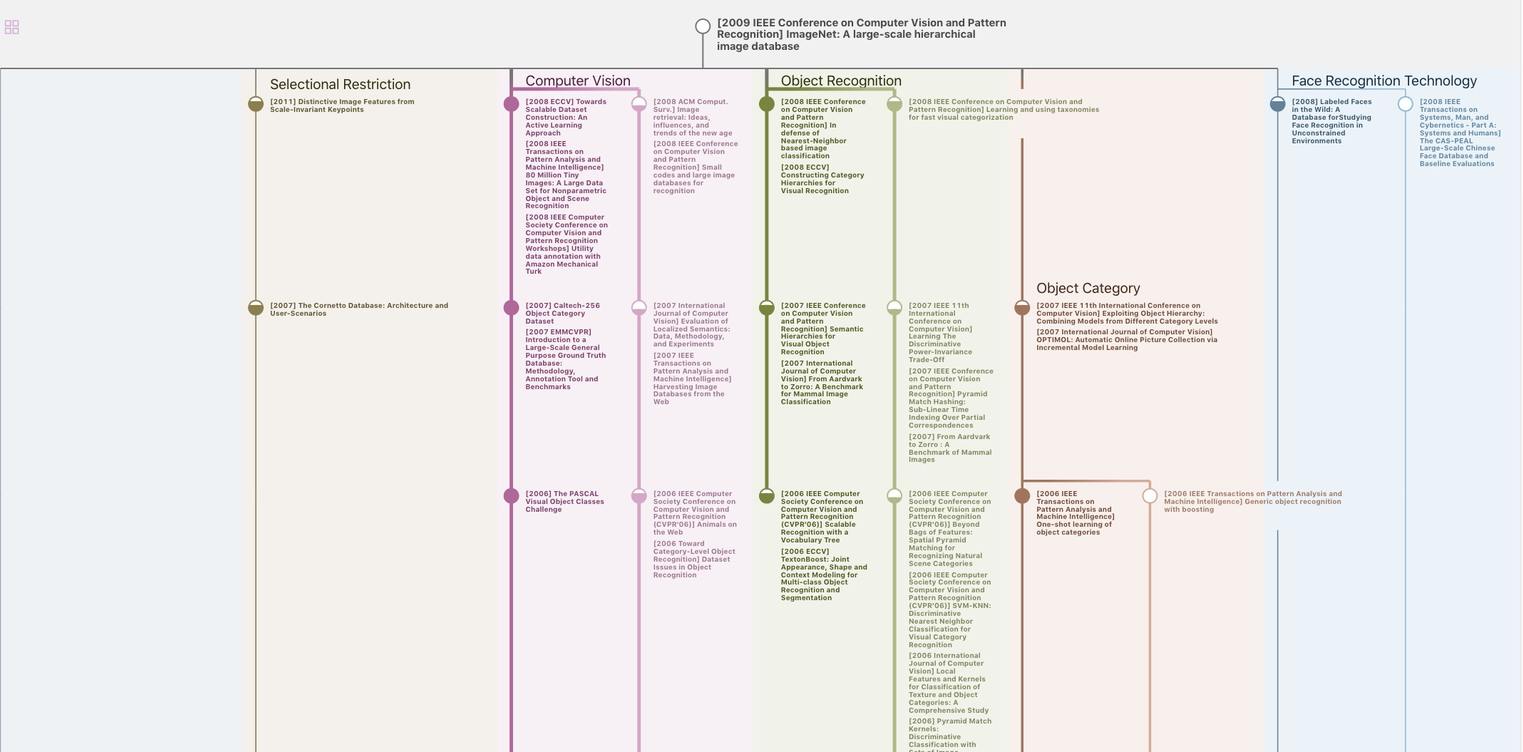
生成溯源树,研究论文发展脉络
Chat Paper
正在生成论文摘要