Deep Q-Network-Enabled Platoon Merging Approach for Autonomous Vehicles
TRANSPORTATION RESEARCH RECORD(2023)
摘要
Platoon merging control of autonomous vehicles driving in a platoon formation can improve traffic efficiency. However, current platoon merging control approaches primarily rely on rules, making it challenging to achieve optimal control. In this study, we propose a platoon merging control approach based on a deep Q-network (DQN). First, we specify the state and action of the vehicle and establish a set of reward functions to ensure safe driving. Then, the DQN algorithm is used to train a neural network suitable for merging the controls of connected and automated vehicles (CAVs) to continuously approach the state-value function. Finally, we compare the proposed approach with a rule-based (RB) vehicle merging approach using a MATLAB simulation. In particular, CAVs are driven simultaneously using the two approaches in a random environment. The simulation results show that the proposed DQN-based vehicle merging approach requires less merging travel time and fewer vehicle lane change times than the RB approach. Additionally, merging can result in improved capacity in medium and high traffic densities compared with no-merging: the higher the CAV penetration rate, the larger the improvement. We verify the effectiveness of the proposed approach for different initial conditions. We suggest that the proposed method is a safe and robust method for CAV platoon merging, and that it can be applied to increase the capacity of freeways and roads.
更多查看译文
关键词
operations, traffic flow theory and characteristics, automated/autonomous vehicles, vehicle-highway automation, platooning
AI 理解论文
溯源树
样例
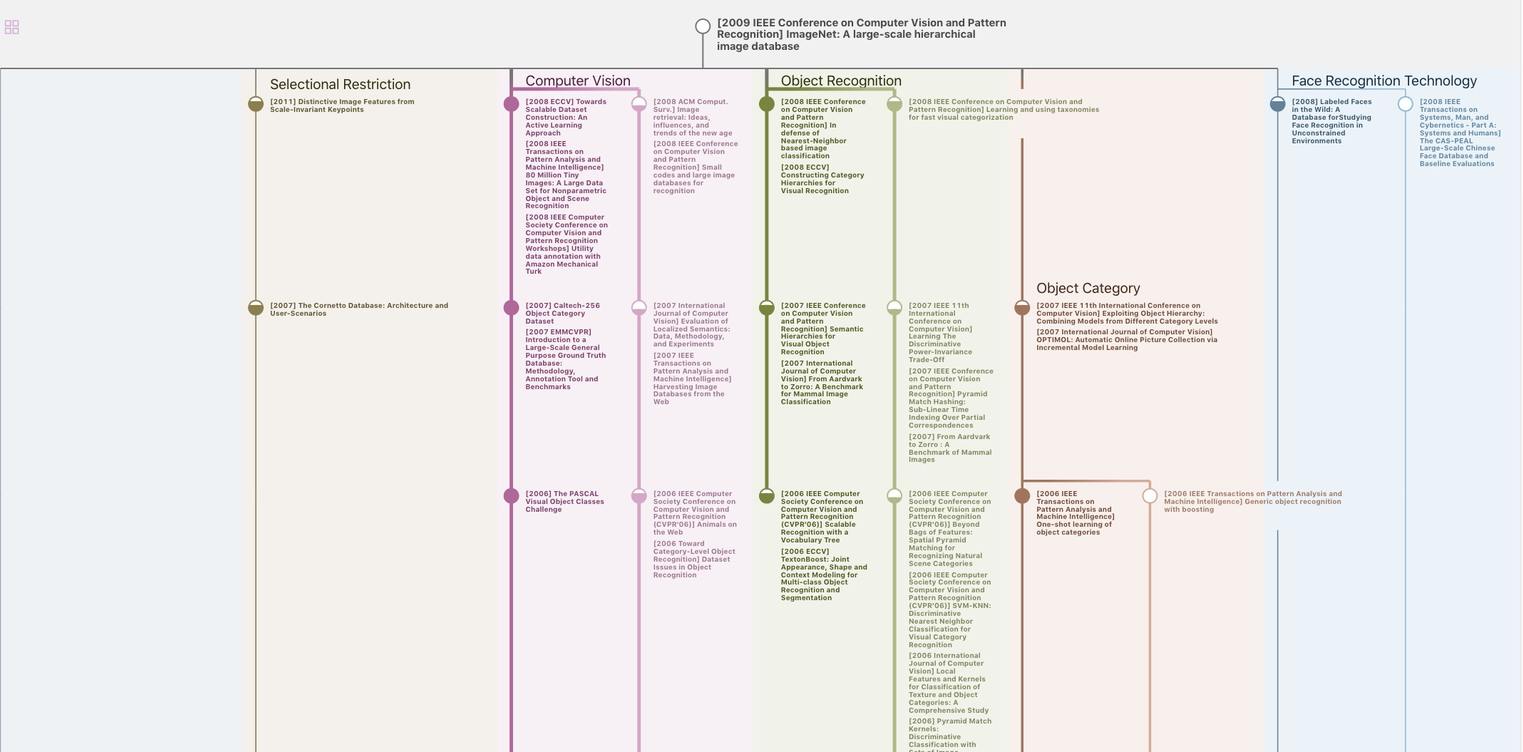
生成溯源树,研究论文发展脉络
Chat Paper
正在生成论文摘要