Multistage damage detection method based on the defect mode for periodically stiffened panels
JOURNAL OF SOUND AND VIBRATION(2024)
摘要
Periodically stiffened panels are extensively used in engineering structures, but methods for their nondestructive testing and evaluation remain limited. The limitations arise due to the intricate vibration modes and Lamb wave signals reflected by the stiffeners and damage. To handle these complexities, the multistage method proposed in this research has solved an inverse problem utilizing the defect modes to detect damage in periodically stiffened panels. The proper selection of vibration frequency related to the local damage mode is important as local vibrations at damage induce additional bandgaps in stiffened panels. Therefore, a damage-related frequency range was selected by comparing the transmission coefficients of healthy and damaged periodically stiffened panels, constituting the first stage. Subsequently, a vibration-based approach was implemented in the second stage to obtain the defect modes using forced vibrations around the selected frequency range and then the damage location was estimated using a baseline-free damage index. To address the difficulty associated with damage size estimation in vibration based methods, a Lamb-wave-based technique was employed in the third stage to accurately quantify the size of damage in the localized regions. To depress the dispersive characteristics of the Lamb waves, the complicated signals reflected by stiffeners and damage were processed using continuous wavelet transform based on Gabor wavelet in time domain. The method was tested on an aluminum periodically stiffened panel with debonding and stiffness reduction damage. The results obtained using numerical simulations and experiments validate the accuracy of the proposed method which exhibits a significant potential for various industrial applications.
更多查看译文
关键词
Nondestructive testing,Periodically stiffened panel,Defect mode,Operational deformation shape,Lamb waves
AI 理解论文
溯源树
样例
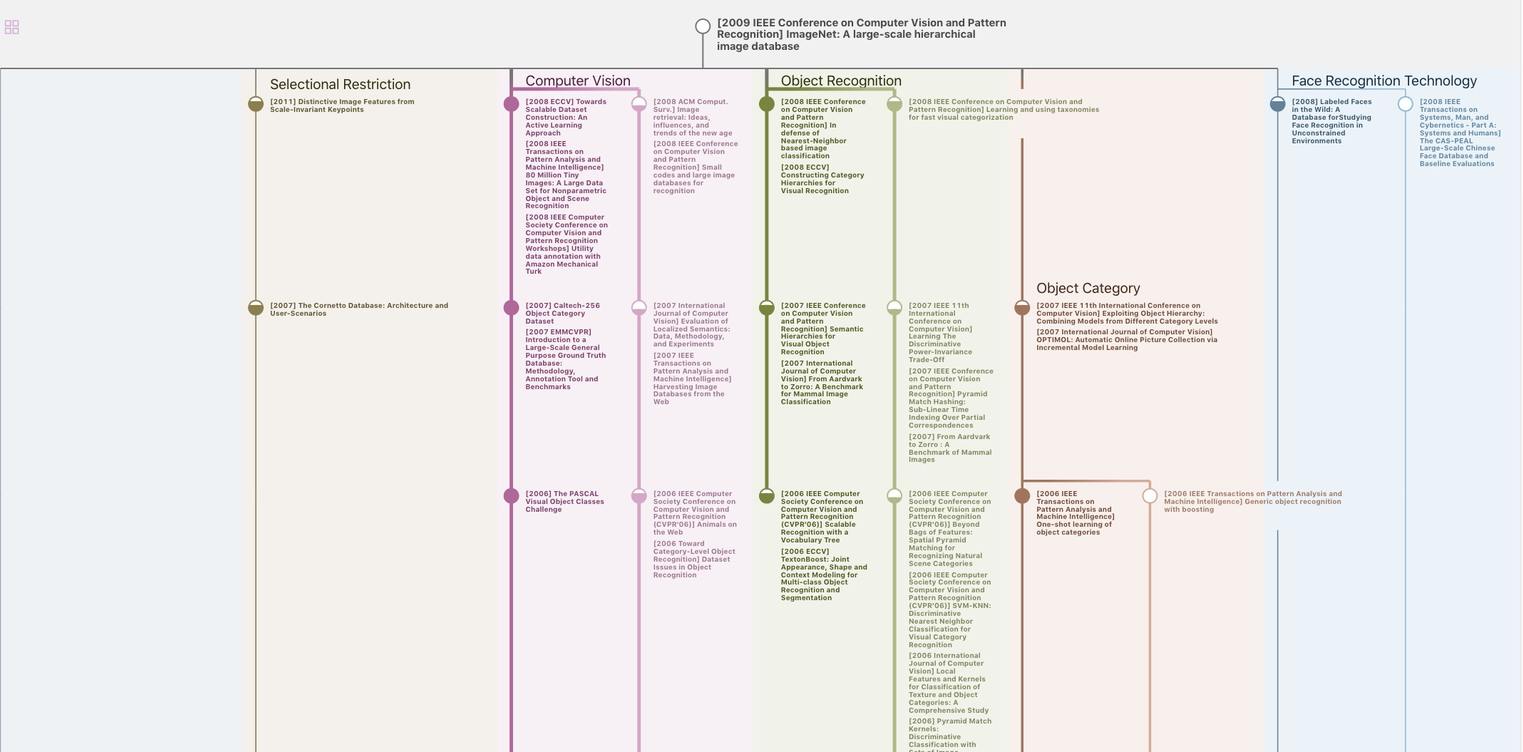
生成溯源树,研究论文发展脉络
Chat Paper
正在生成论文摘要