A hybrid of RainNet and genetic algorithm in nowcasting prediction
EARTH SCIENCE INFORMATICS(2023)
摘要
Quantitative precipitation nowcasting QPN is a powerful tool with many applications, such as rainfall prediction, urban sewage control, etc. Recently, researchers have focused on using machine learning and deep learning models for nowcasting raindrops. A few famous frameworks for nowcasting raindrops in a short leading time are Rainymotion, Segnet, Unet and RainNet. Rainymotion was developed using machine learning models and extracting areas of interest. Segnet is a deep, fully convolutional neural network architecture for semantic pixel-wise segmentation with a core trainable segmentation engine consisting of an encoder network, a corresponding decoder network followed by a pixel-wise classification layer while the U-net is a radar data-based model for precipitation nowcasting. The U-Net architecture is based on convolutional neural networks CNNs. The three models, RainyMotion, U-Net, and Segnet, are used to compare the experiment results. In this manuscript, we combined the genetic algorithm GA with the RainNet model to improve the nowcasting performance of the model RainNet. The GA is used to tune hyperparameters such as filter size, activation function, and the number of epochs for the model. To enhance the performance of the baseline models for nowcasting, an objective function is defined to decrease the mean absolute error MAE below 0.3 for the next 5 minutes. The proposed algorithm was evaluated on radar images taken by the German Weather Service DWD, a German Meteorological Service based in Offenbach am Main, a city in Hesse, Germany, which monitors weather and meteorological conditions over Germany. The result from our experiments obtained indicated that the RainNet+GA model is more accurate in predicting short-term raindrops but requires an expensive implementation of a powerful computer to run on Graphical processor Unit GPU. Our hybrid model RainNet +GA did not overcome the computational power of using GPU. However, the experimental results with a loss of 0.021 showed a much smaller mean absolute error value for the next 5 minutes compared to other models and the original RainNet structure. With the use of GA, it has been proven that using novel developed activation functions such as Scaled Exponential Linear Units’ SELU’ and ‘Swish’ is more desirable than the rectified linear activation function ReLU.
更多查看译文
关键词
rainnet,genetic algorithm,prediction
AI 理解论文
溯源树
样例
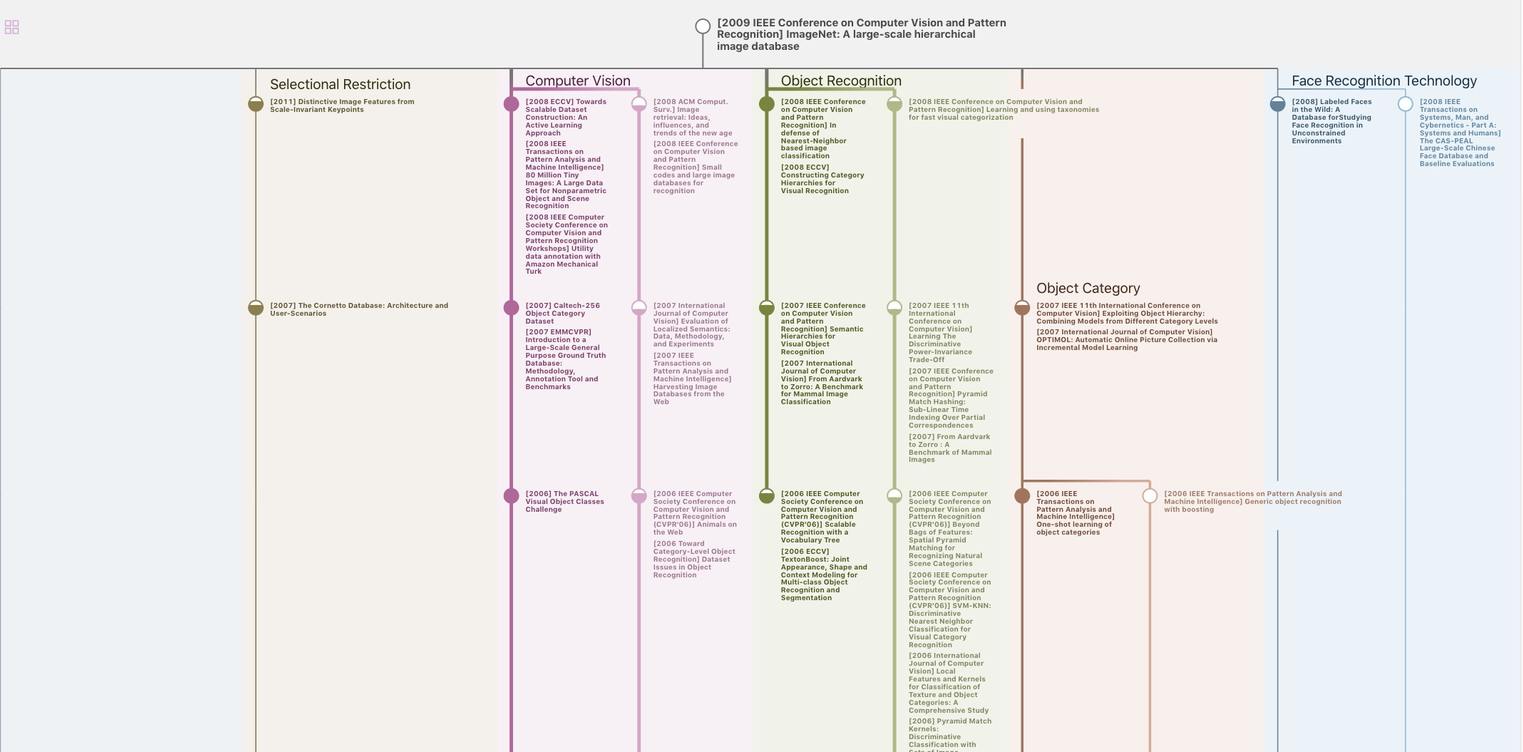
生成溯源树,研究论文发展脉络
Chat Paper
正在生成论文摘要