Driving school for self-driving labs
DIGITAL DISCOVERY(2023)
摘要
Self-driving labs (SDLs) have emerged as a strategy for accelerating materials and chemical research. While such systems autonomously select and perform physical experiments, this does not mean that the human experimenter has no role to play. Instead, the experimenter must monitor progress of the SDL and make adjustments to ensure that the SDL progresses towards the chosen goal. Unfortunately, researchers rarely receive training specific to the unique challenges inherent to operating SDLs. Here, we provide a heuristic framework for SDL operators. In analogy with how a human might operate a car or other complex system, this framework defines the knobs and the gauges, or the SDL settings that can be modified and the information that an experimenter can consider to change these settings. These lessons are discussed in the context of a common optimization strategy (Bayesian optimization using Gaussian process regression) but can be generalized to other models. Crucially, these adjustments constitute fine tunings that can occur at any point during an SDL campaign, allowing the experimenter to participate in this closed-loop process without being in the loop. As the framework introduced here is material-system agnostic, it can form a resource for researchers developing or using SDLs in any discipline. Self-driving labs benefit from occasional and asynchronous human interventions. We present a heuristic framework for how self-driving lab operators can interpret progress and make changes during a campaign.
更多查看译文
关键词
school,self-driving
AI 理解论文
溯源树
样例
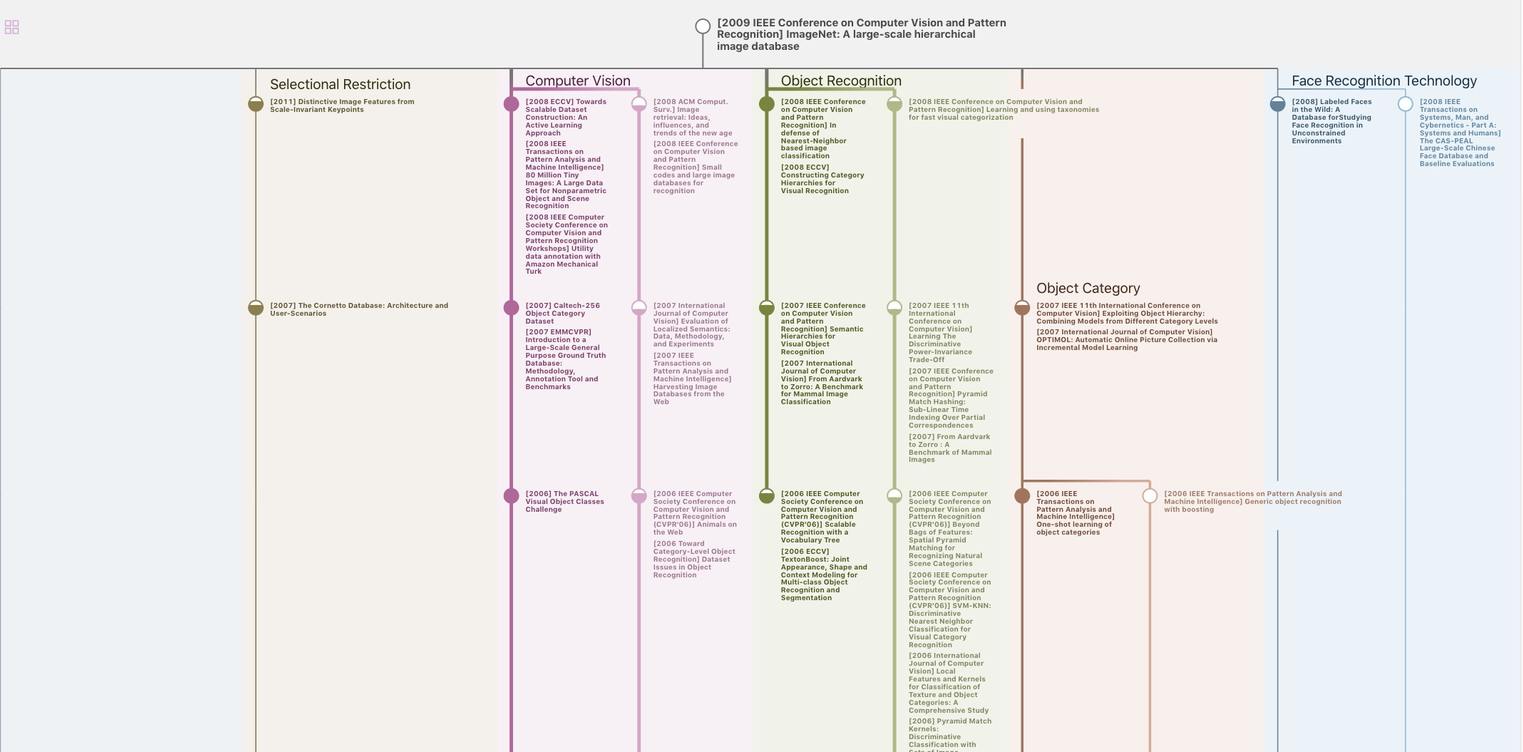
生成溯源树,研究论文发展脉络
Chat Paper
正在生成论文摘要