A real-time seismic damage prediction framework based on machine learning for earthquake early warning
EARTHQUAKE ENGINEERING & STRUCTURAL DYNAMICS(2024)
摘要
For implementing earthquake early warning (EEW), real-time parameters of ground motion (GM) which can be obtained at the beginning of earthquake wave arrival (i.e., P-wave arrival) are usually used to estimate the intensity measure (IM) of the following GM for supporting alarm decision. However, prior studies have proved that the estimated IM is hard to relate structural damage effectively, thus affecting the decision-making behavior and resulting in more economic losses and casualties. Although the real-time parameters may be adopted to directly predict structural damage, it is difficult to construct the accurate mathematical model between the parameters and structural damage index. To solve the above problems, a machine learning-based real-time damage prediction framework that can directly predict the structural maximum inter-story drift ratio (MIDR) after P-wave arrival is proposed for use in EEW. Within the framework of the proposed method, the database for training the prediction model come from the accurate nonlinear time-history analyses of 32 typical building types under the real GM records, where the real-time characteristic parameters of P-wave of the records are taken as the inputs. To achieve accurate and efficient prediction, a total of 32 alternative characteristic parameters of P-wave are selected and a parameter optimization approach is developed to remove the redundant and useless parameters for various type of building structure. Moreover, an interpretable approach is adopted to explain the effects of different input parameters on the prediction results and demonstrate the reliability of the proposed method. Finally, the framework is thoroughly assessed and investigated through two seismic damage cases and an occurred seismic event, which has an accuracy rate above 96.4% on average for risk-consistent early-warning trigger classification. These results show that the proposed method have the ability of continuously stable and excellent prediction power when using it for EEW.
更多查看译文
关键词
damage prediction,earthquake early warning,information theory,interpretability of the trained model,machine learning,the optimal parameter combination
AI 理解论文
溯源树
样例
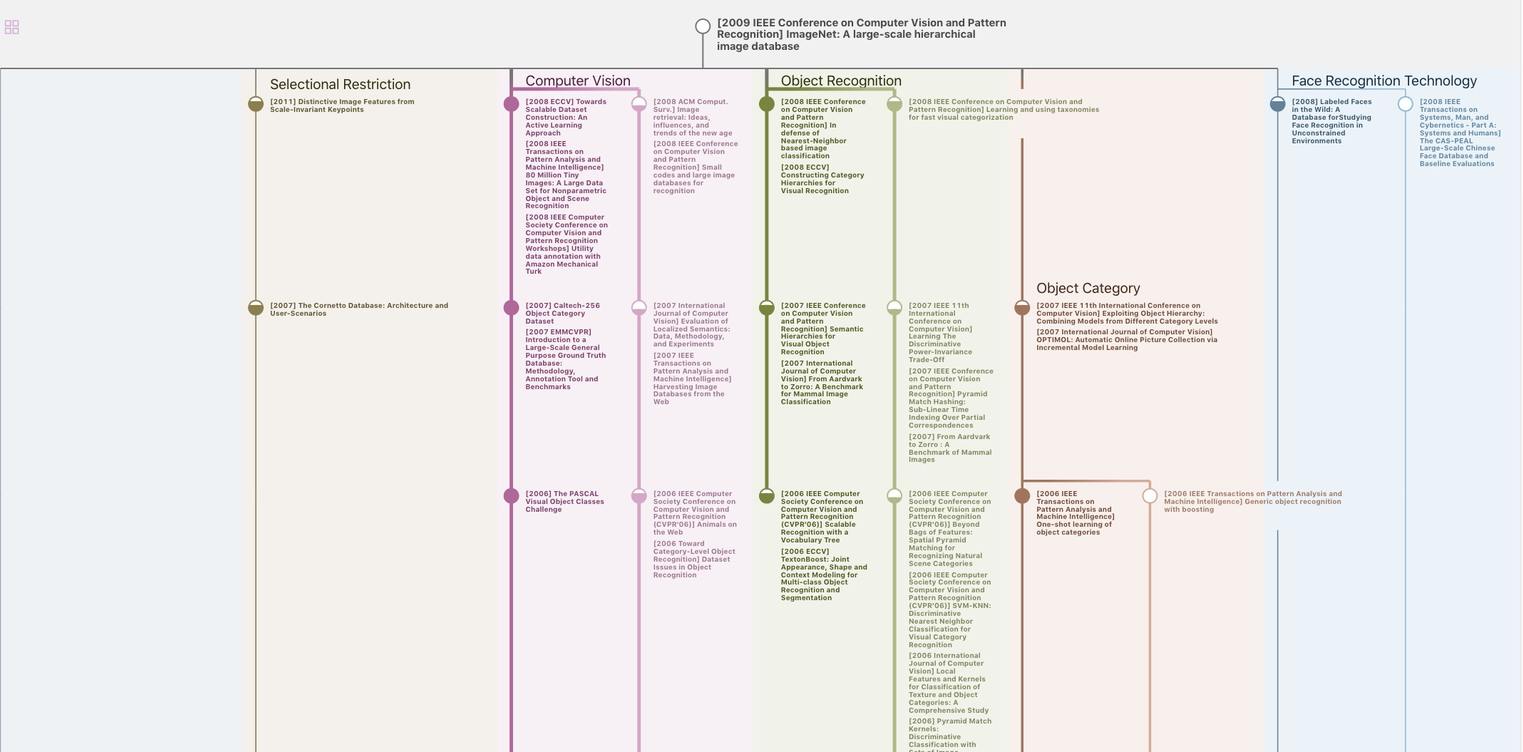
生成溯源树,研究论文发展脉络
Chat Paper
正在生成论文摘要