Capturing the Spread of Information in Heterogeneous V2X Through Scalable Computation
IEEE-ACM TRANSACTIONS ON NETWORKING(2023)
摘要
Emerging V2X technology enables vehicles to exchange messages with each other (V2V) and with signaling infrastructure (I2V) on the roadways. Information propagation in transportation networks is highly influenced by both vehicle mobility and wireless communication. As for vehicle mobility, realistic traffic flow changes with time, exhibiting sharp time-triggered transitions, due to external factors such as traffic lights. Thus, mobility process is temporally heterogeneous and not smooth, which fundamentally alters the dynamics of V2X (V2V and I2V together) message propagation in a complex manner. As for wireless communication, communication heterogeneity is an integral component of V2X systems -different types of vehicles may have different communication capabilities, and V2V and I2V communications coexist. We propose a mathematical framework, based on a continuous-time Markov chain (CTMC), for characterizing the spatio-temporal spread of V2X information (1) when the traffic flow exhibits sharp time-triggered transitions and (2) when there exists communication heterogeneity comprising of different V2V commutation capabilities, different wireless communication conditions, and both V2V and I2V. We prove that the state evolutions under the CTMC model converge to a set of differential equations in the asymptotic limit of a large number of vehicles, enabling computations that gracefully scale with increase in network size and the number of vehicles. Our framework can accommodate arbitrary traffic synchronization patterns corresponding for example to incorporate the presence of an arbitrary number of traffic signals. Furthermore, numerical computations using this mathematical framework answer several questions that influence the practice of V2X network design and security.
更多查看译文
关键词
V2X communication,vehicular mobility,information propagation,traffic signals,transportation network
AI 理解论文
溯源树
样例
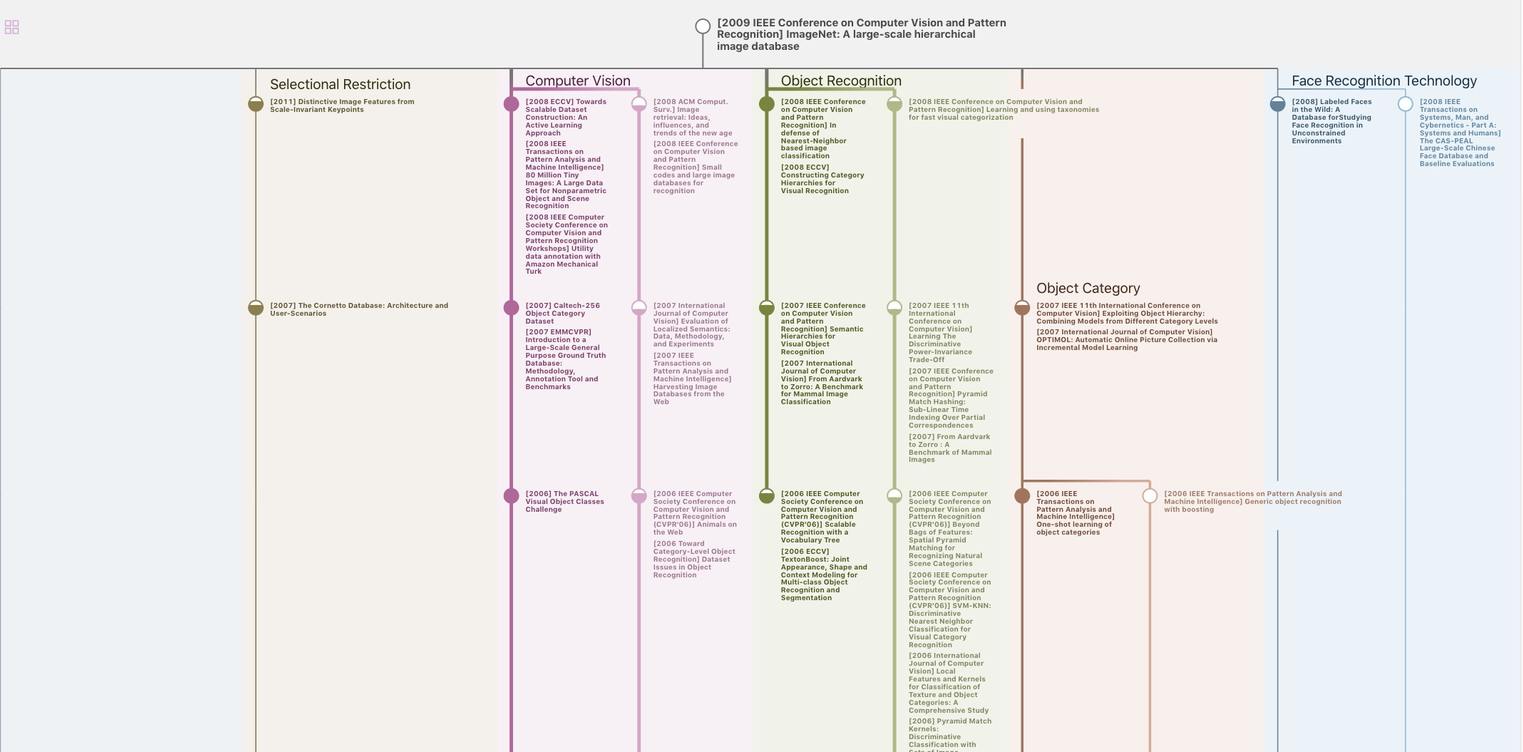
生成溯源树,研究论文发展脉络
Chat Paper
正在生成论文摘要