DDI-SSL: Drug-Drug Interaction Prediction Based on Substructure Signature Learning
APPLIED SCIENCES-BASEL(2023)
摘要
Drug-drug interactions (DDIs) are entities composed of different chemical substructures (functional groups). In existing methods that predict drug-drug interactions based on the usage of substructures, each node is perceived as the epicenter of a sub-pattern, and adjacent nodes eventually become centers of similar substructures, resulting in redundancy. Furthermore, the significant differences in structure and properties among compounds can lead to unrelated pairings, making it difficult to integrate information. This heterogeneity negatively affects the prediction results. In response to these challenges, we propose a drug-drug interaction prediction method based on substructure signature learning (DDI-SSL). This method extracts useful information from local subgraphs surrounding drugs and effectively utilizes substructures to assist in predicting drug side effects. Additionally, a deep clustering algorithm is used to aggregate similar substructures, allowing any individual subgraph to be reconstructed using this set of global signatures. Furthermore, we developed a layer-independent collaborative attention mechanism to model the mutual influence between drugs, generating signal strength scores for each class of drugs to mitigate noise caused by heterogeneity. Finally, we evaluated DDI-SSL on a comprehensive dataset and demonstrated improved performance in DDI prediction compared to state-of-the-art methods.
更多查看译文
关键词
drug-drug interactions, substructure graph convolution operator, substructure signatures, substructure extraction, collaborative attention mechanism
AI 理解论文
溯源树
样例
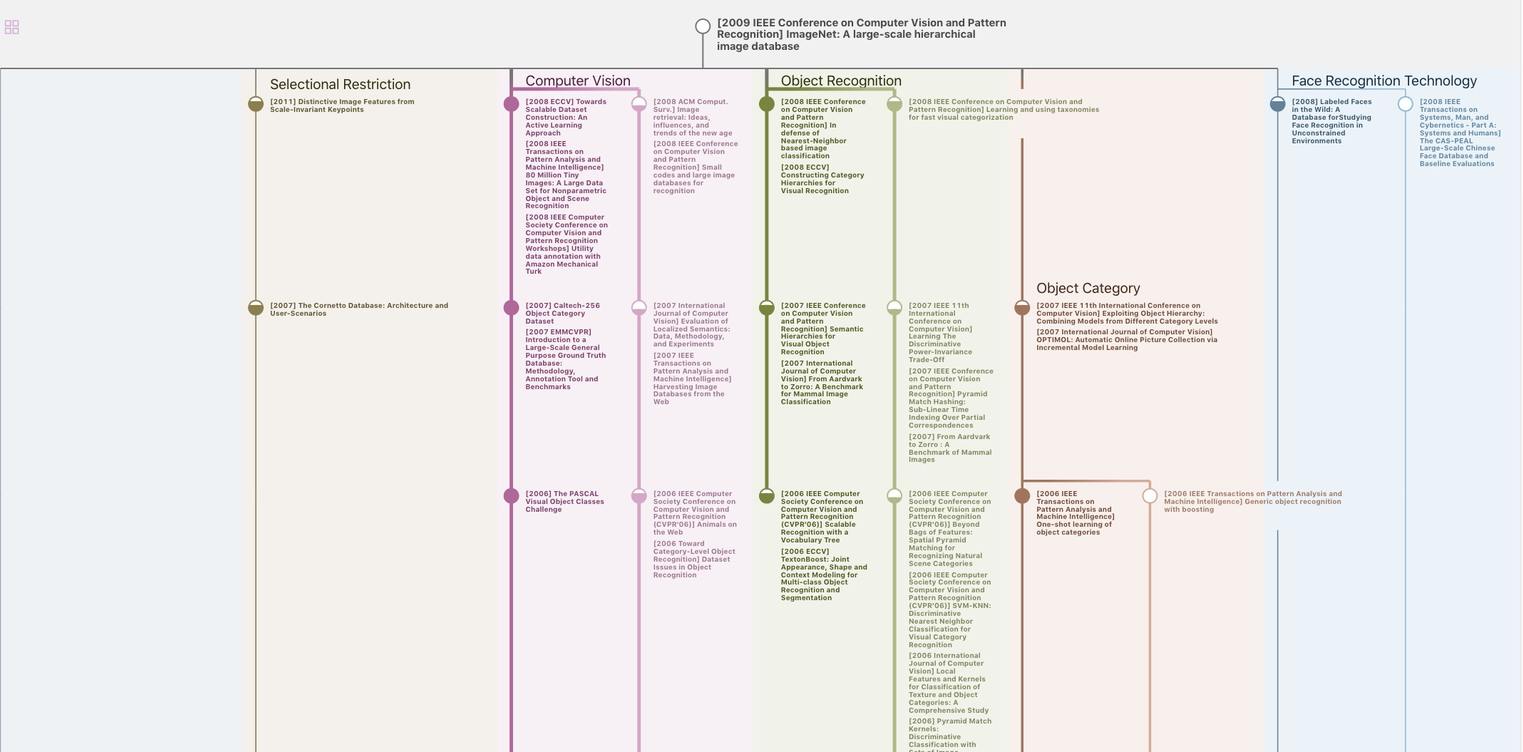
生成溯源树,研究论文发展脉络
Chat Paper
正在生成论文摘要