DeepFake detection against adversarial examples based on D-VAEGAN
IET IMAGE PROCESSING(2024)
摘要
Recent years, the development of DeepFake has raise a lot of security problems. Therefore, detection of DeepFake is critical. However, the existing DeepFake detection methods are often vulnerable to adversarial attacks, i.e. adding carefully crafted imperceptible perturbations into forged images is possible to evade detection. In this paper, a DeepFake detection method based on image denoising is proposed by combining variational autoencoder (VAE) and generative adversarial network (GAN), namely D-VAEGAN. Firstly, an encoder is designed to extract the features of the image in a low-dimensional latent space. Then, a decoder reconstructs the original clean image using the features in this low-dimensional latent space. Secondly, an auxiliary discriminative network is introduced to further improve the performance of the model, which improves the quality of the reconstructed images. Furthermore, feature similarity loss is added as a penalty term to the reconstruction optimization function to improve the adversarial robustness. Experimental results on the FaceForensics++ dataset show that the proposed approach significantly outperforms the five adversarial training-based defence methods. The approach achieves 96% in accuracy, which is on average about 50% higher than other comparison methods. The existing DeepFake detection methods are often vulnerable to adversarial attacks, so we propose a DeepFake detection method based on image denoising by combining variational autoencoder (VAE) and generative adversarial network (GAN), namely D-VAEGAN.image
更多查看译文
关键词
computer vision,image denoising
AI 理解论文
溯源树
样例
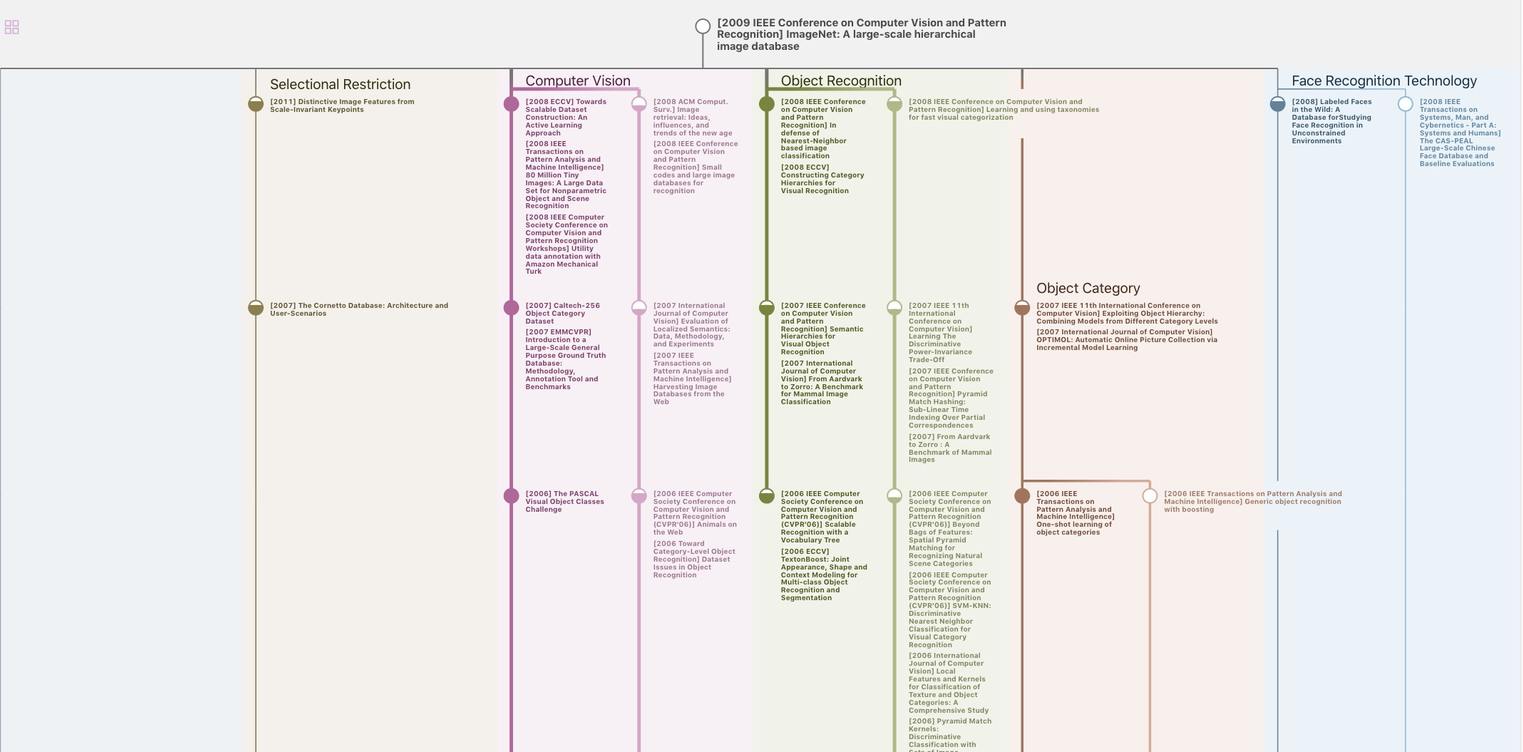
生成溯源树,研究论文发展脉络
Chat Paper
正在生成论文摘要