Time series classification of dynamical systems using deterministic learning
Nonlinear Dynamics(2023)
摘要
This paper studies the classification of large-scale time series data constructed by nonlinear dynamical systems via deterministic learning. Firstly, a large-scale time series dataset including five classes of dynamical patterns is constructed based on the benchmark Lorenz system. Secondly, the performance of the dynamical pattern recognition method based on deterministic learning is evaluated for the time series classification task in the large-scale time series dataset. Last but not least, based on the concept of representative selection and the recent research on the deterministic learning theory, a novel dynamical pattern recognition-based time series classification framework is modified, including: (1) the selection of a representative training subset with the largest Lyapunov exponent as an example; (2) inherent dynamics modeling via deterministic learning in the representative subset; (3) dynamics comparing between representative training patterns and a given test pattern through generating recognition errors; (4) and label assigning based on the minimal recognition error principle. The significance of this paper is that it improves the application of existing dynamical pattern recognition methods to large-scale datasets by incorporating representative selection strategies. The proposed method can achieve comparable classification accuracy on a small representative subset of chaotic trajectories, as compared to the original large-scale training set. Numerical simulations are provided to demonstrate the effectiveness of the proposed method. The simulation results show that the proposed method outperforms even the baseline deep learning methods on the representative subset in the dynamical system classification tasks.
更多查看译文
关键词
time series classification,dynamical systems,learning
AI 理解论文
溯源树
样例
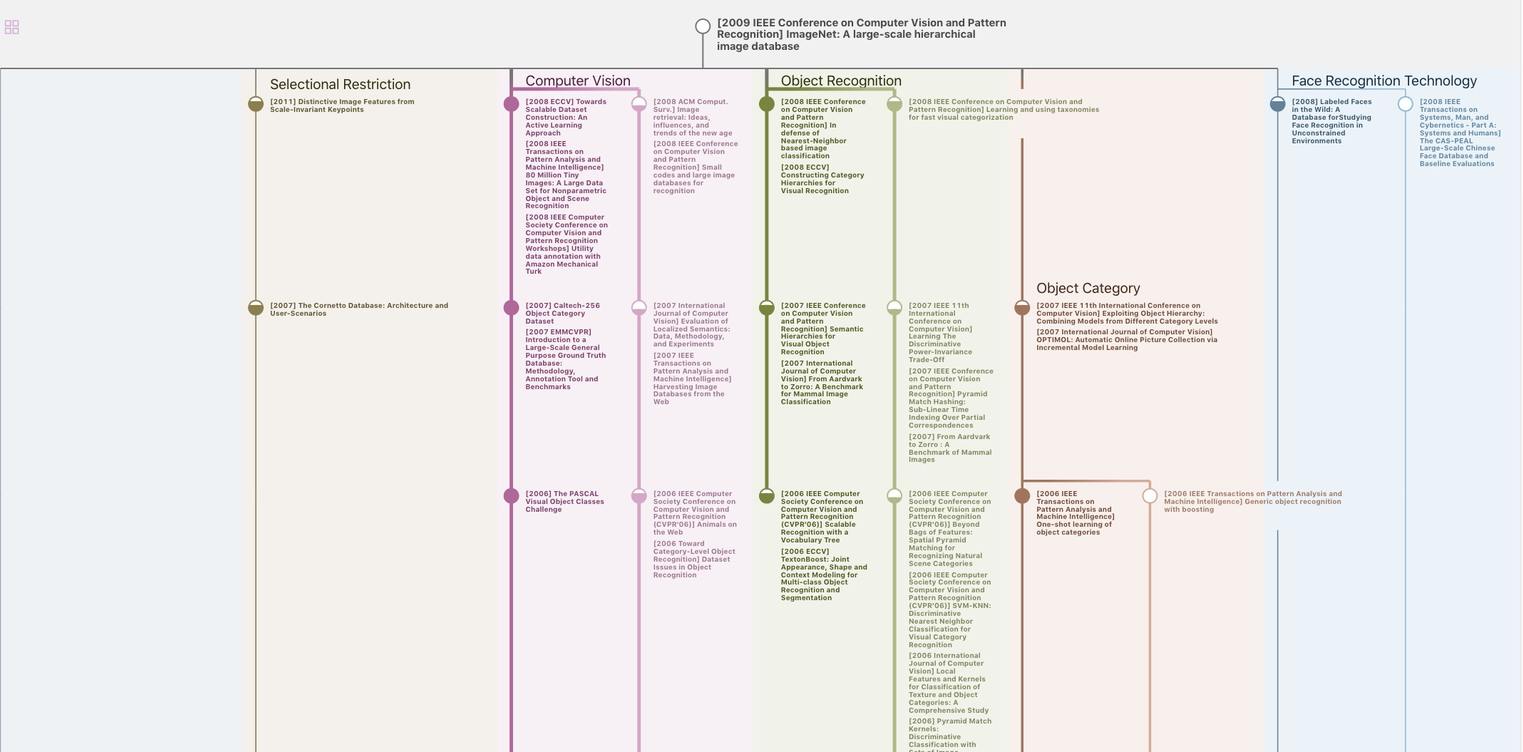
生成溯源树,研究论文发展脉络
Chat Paper
正在生成论文摘要