Hierarchical Spatial-Temporal Adaptive Graph Fusion for Monocular 3D Human Pose Estimation
IEEE SIGNAL PROCESSING LETTERS(2024)
摘要
Single-view 3D human pose estimation (HPE) based on Graph Convolutional Networks currently suffers from problems such as insufficient feature representation and depth ambiguity. To address these issues, this letter proposes a hierarchical spatial-temporal adaptive graph fusion framework to improve monocular 3D HPE performance. Firstly, to enhance the spatial semantic feature representation of human nodes, a progressive adaptive graph feature capture strategy is developed, which adaptively constructs global-to-local attention graph features of all human joints in a coarse-to-fine manner. A spatial-temporal attention fusion module is then constructed to model long-term sequential dependencies and mitigate depth ambiguity. The temporal attention factors of related frames are captured and utilized to intermediately supervise the joint depth. The spatial semantic information among all joints in the same frame and temporal contextual knowledge of the joints across relevant frames are fused to build spatial-temporal correlations and optimize the final features. Extensive experiments on two popular benchmarks show that our method outperforms several state-of-the-art approaches and improves 3D HPE performance.
更多查看译文
关键词
3D human pose estimation,attention mechanism,graph convolutional network,spatial-temporal fusion
AI 理解论文
溯源树
样例
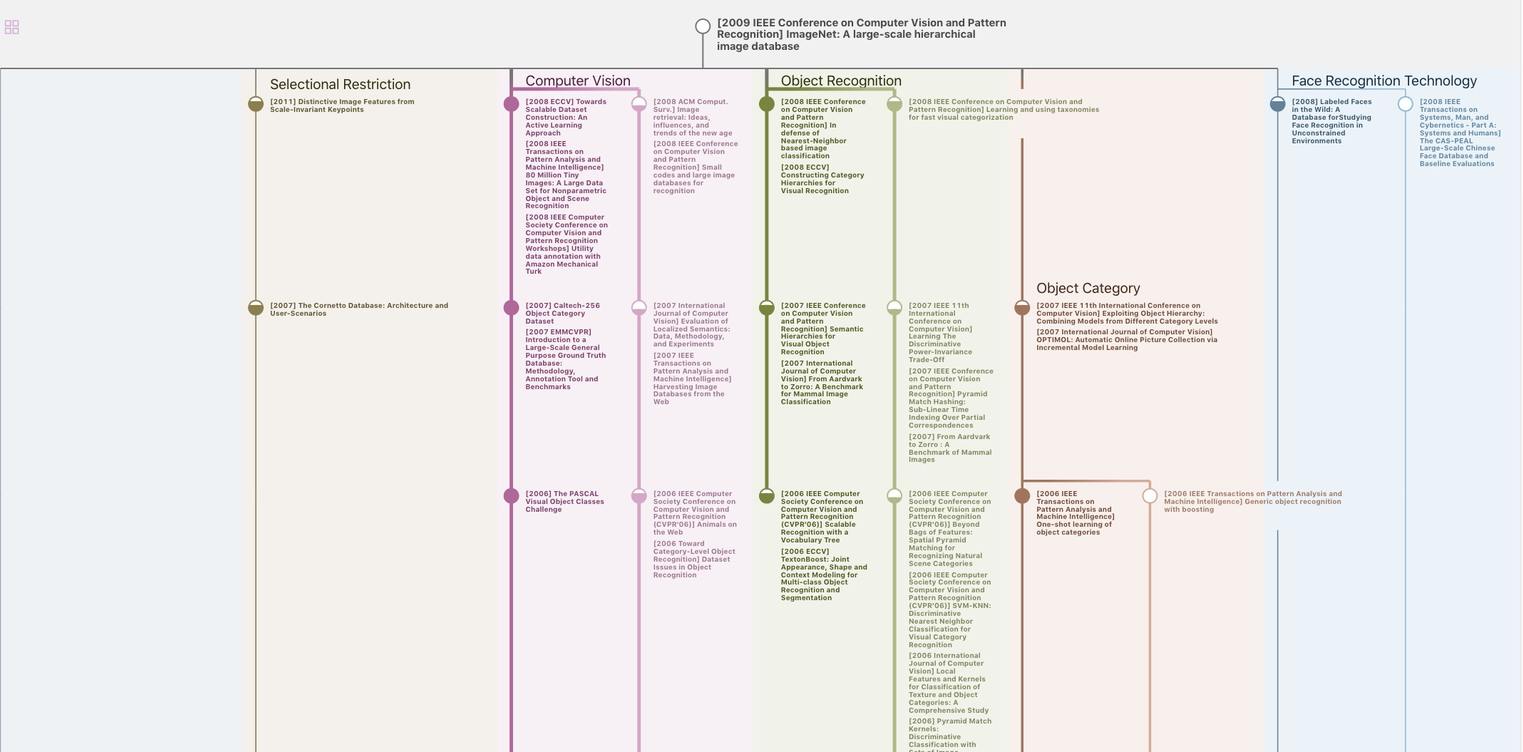
生成溯源树,研究论文发展脉络
Chat Paper
正在生成论文摘要