AE-Net: Novel Autoencoder-Based Deep Features for SQL Injection Attack Detection
IEEE ACCESS(2023)
摘要
Structured Query Language (SQL) injection attacks represent a critical threat to database-driven applications and systems, exploiting vulnerabilities in input fields to inject malicious SQL code into database queries. This unauthorized access enables attackers to manipulate, retrieve, or even delete sensitive data. The unauthorized access through SQL injection attacks underscores the critical importance of robust Artificial Intelligence (AI) based security measures to safeguard against SQL injection attacks. This study's primary objective is the automated and timely detection of SQL injection attacks through AI without human intervention. Utilizing a preprocessed database of 46,392 SQL queries, we introduce a novel optimized approach, the Autoencoder network (AE-Net), for automatic feature engineering. The proposed AE-Net extracts new high-level deep features from SQL textual data, subsequently input into machine learning models for performance evaluations. Extensive experimental evaluation reveals that the extreme gradient boosting classifier outperforms existing studies with an impressive k-fold accuracy score of 0.99 for SQL injection detection. Each applied learning approach's performance is further enhanced through hyperparameter tuning and validated via k-fold cross-validation. Additionally, statistical t-test analysis is applied to assess performance variations. Our innovative research has the potential to revolutionize the timely detection of SQL injection attacks, benefiting security specialists and organizations.
更多查看译文
关键词
Autoencoder optimization,deep learning,feature engineering,machine learning
AI 理解论文
溯源树
样例
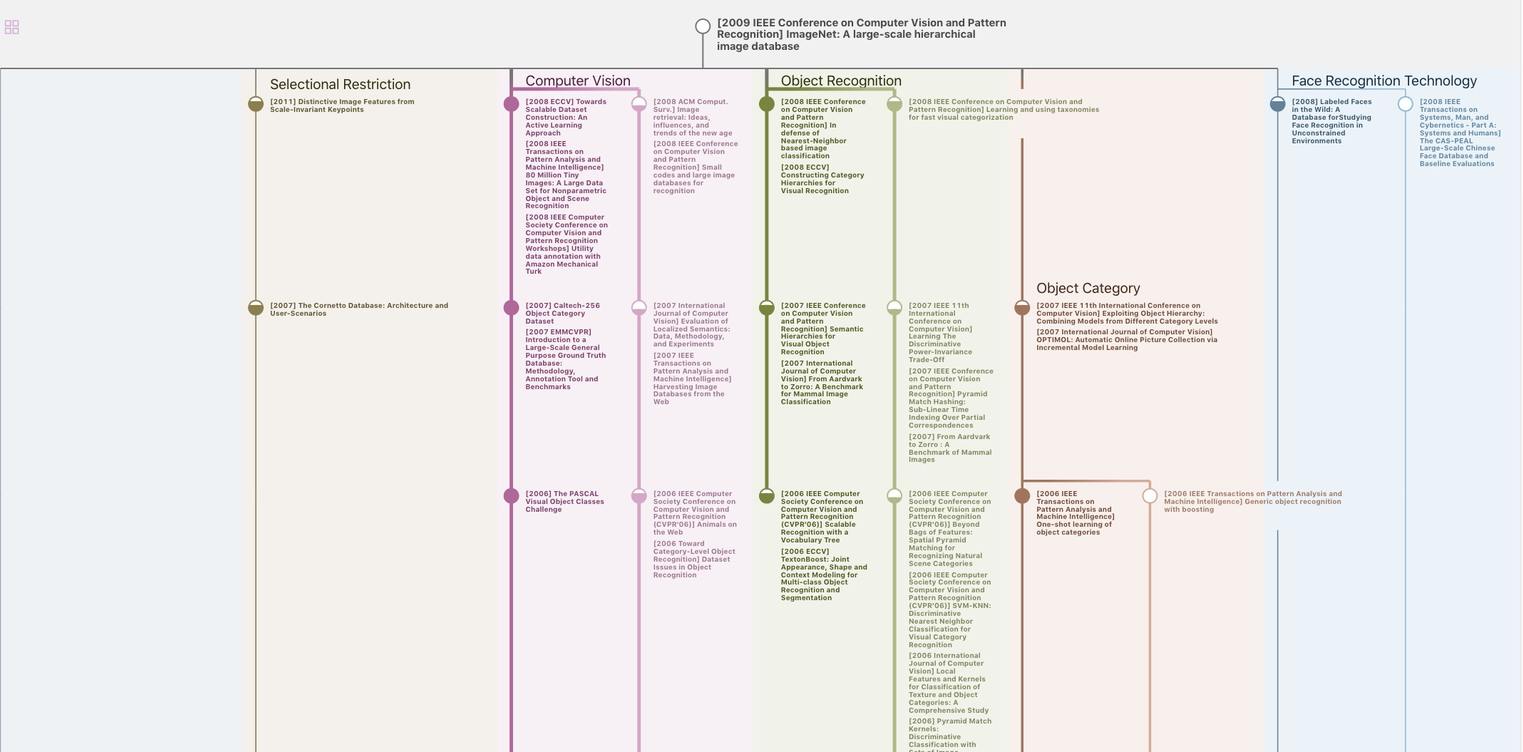
生成溯源树,研究论文发展脉络
Chat Paper
正在生成论文摘要