Research on Bidirectional LSTM Recurrent Neural Network in Speech Recognition
2023 IEEE 6th International Conference on Pattern Recognition and Artificial Intelligence (PRAI)(2023)
摘要
Speech recognition is a very useful artificial intelligence technology, which has been widely used in various fields and has great potential for development. With the continuous progress of technology, speech recognition will become an indispensable part of people’s daily life, but in the process of further development of speech recognition, there are still many problems that have not been solved. For example, speech signals are susceptible to a variety of interference factors, such as noise, speaker accent, which may lead to the degradation of speech recognition performance. In speech recognition, it is often necessary to process long time sequences of speech signals, such as continuous conversations of speakers, long sentences in speech recognition tasks. Traditional recurrent neural networks may suffer from gradient disappearance or gradient explosion, which makes it difficult for the model to capture long-term dependencies. In this paper, our primary focus is designing a bidirectional LSTM recurrent network structure [10]. We utilize the CTC algorithm to calculate the loss and employ the cluster search decoding algorithm for decoding the network’s output. Compared with the bidirectional LSTM recurrent neural network with 5 hidden layers, the pinyin text recognition can reach 85% correct rate, 5% less training times, and 6% less wrong pinyin words in individual sentences at 15%. Some of the recognition rates presented in this paper may appear suboptimal due to noise in individual speech signals and other factors. How to denoise has been the focus of speech recognition in neural networks.
更多查看译文
关键词
Neural Networks,Speech recognition,Mel frequency cepstrum coefficient,Deep Learning
AI 理解论文
溯源树
样例
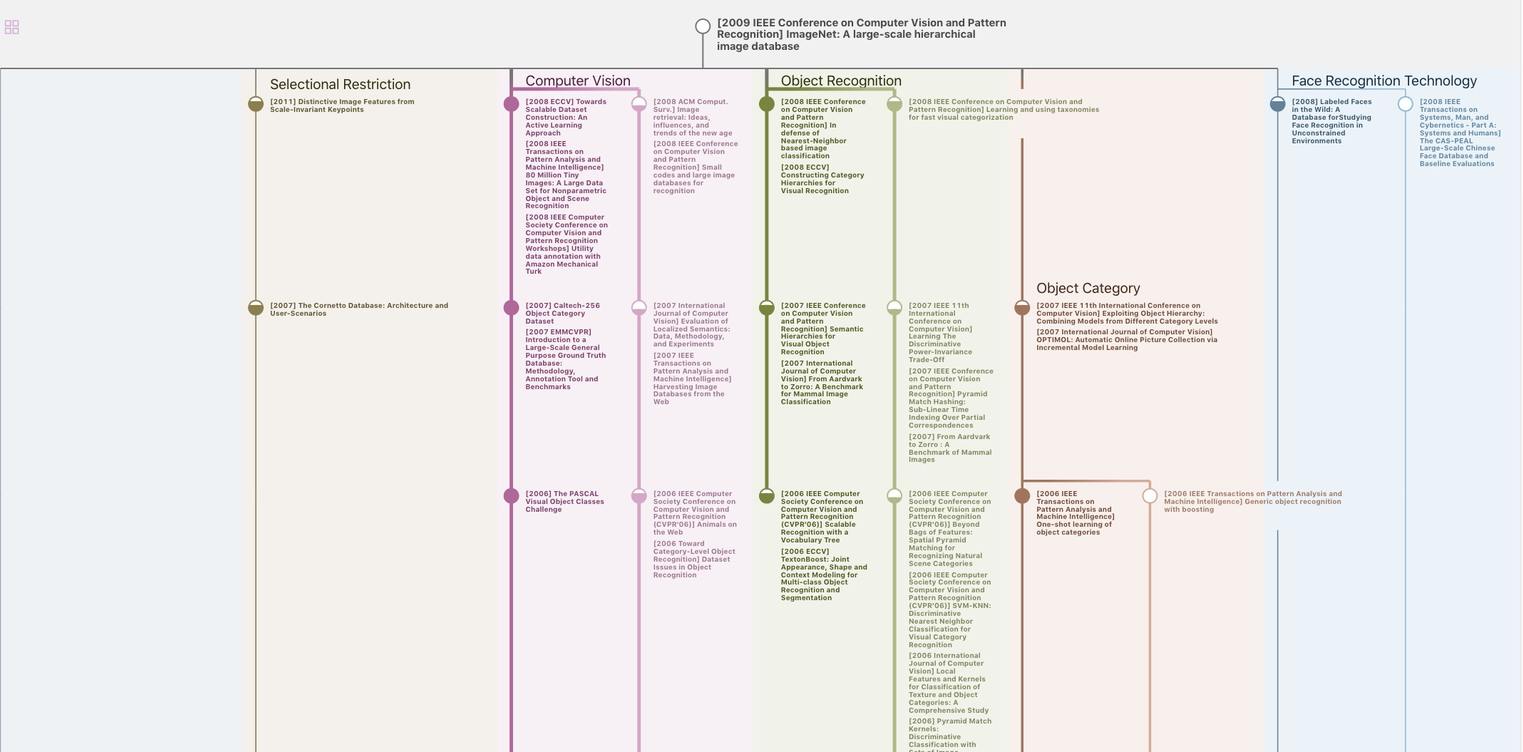
生成溯源树,研究论文发展脉络
Chat Paper
正在生成论文摘要