DTFL: A Digital Twin-Assisted Graph Neural Network Approach for Service Function Chains Failure Localization
IEEE TRANSACTIONS ON CLOUD COMPUTING(2023)
Abstract
Cloud computing enables Network Function Virtualization to dynamically provide and deploy network functions (NFs) to meet business-specific requirements. This approach streamlines NFs' lifecycle management and lowers the cost of Operation Administration and Maintenance. However, these advantages cause Service Function Chain (SFC) failure to grow in both scope and dimensionality, making it difficult to establish a model to locate the failure effectively. In this paper, we propose a complete analysis scheme DTFL (Digital Twin (DT) based for SFC failure localization (FL)) through the following two steps: one is classifying and locating failures, and the other is conducting root cause analysis. We propose transGNN based on the Graph Neural Network and improved graph search model to achieve the classification and location for SFC failures. On this basis, the FNSG-RCA algorithm (failure based graph model) is proposed to analyze failures. We build a prototype based on the cloud platform and experimental results show that this scheme can achieve an accuracy rate of over 98% in fine-grained classification of 49 failure types. In addition, DTFL delivers desirable performance in RCA, approximately 13% more accurate than SOTA, the state-of-the-art approach. DTFL improves both RCA accuracy and model deployment efficiency compared with the non-DT approaches.
MoreTranslated text
Key words
Service function chain,digital twin,graph neural network,transfer learning,failure localization
AI Read Science
Must-Reading Tree
Example
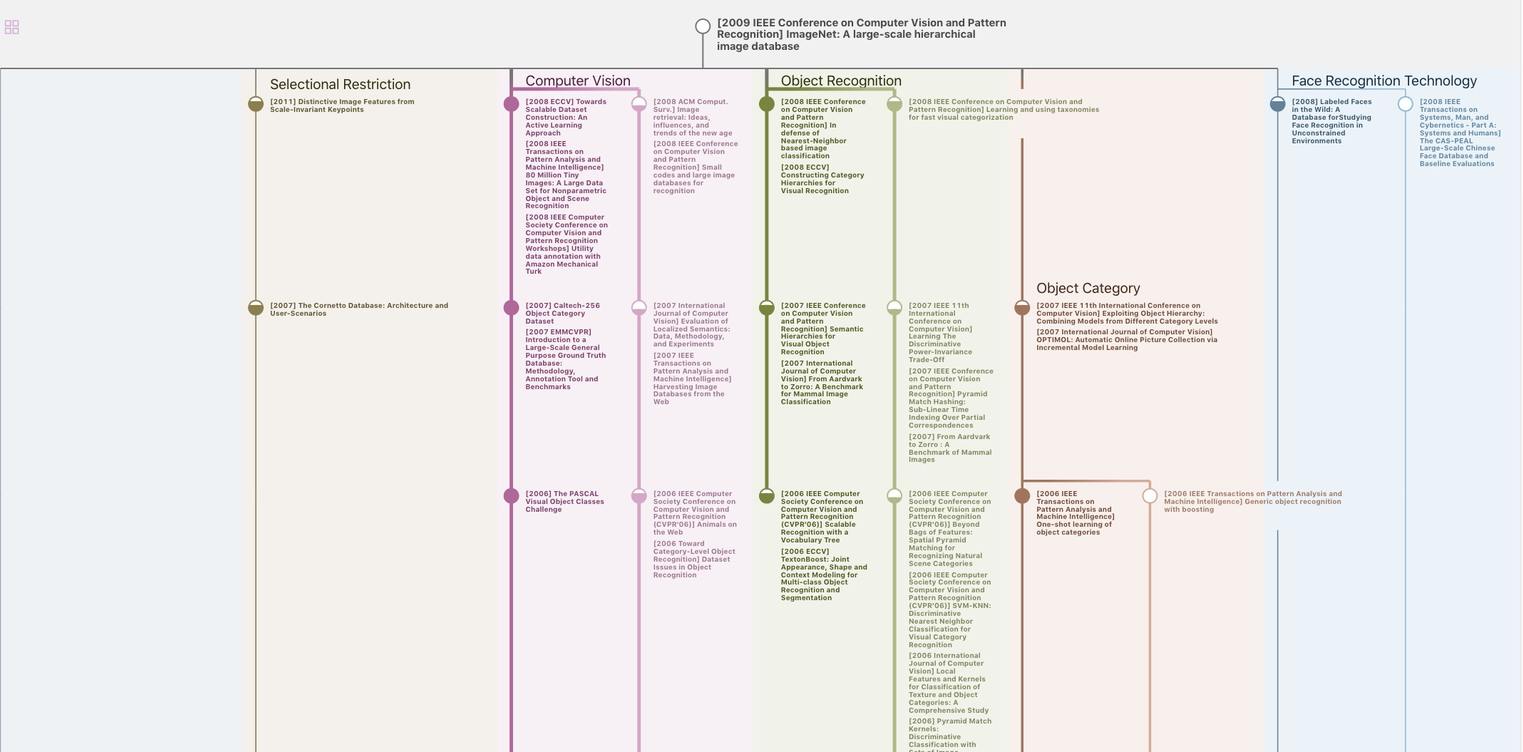
Generate MRT to find the research sequence of this paper
Chat Paper
Summary is being generated by the instructions you defined