Optimization of Force-field Potential parameters using conditional variational autoencoder
Science and Technology of Advanced Materials: Methods(2023)
摘要
Owing to their high-speed, force-field (FF) calculations for inorganic solid-state materials using the parametric potential have been widely employed as an effective tool for high-throughput calculations such as exhaustive property evaluation of material databases and/or calculations for models containing tens of thousands of atoms or more, including amorphous and grain boundary models. However, the accuracy of such calculations strongly depends on the choice of the FF parameters. Today, compounds containing three or more elements are targeted in the research and development of functional materials, in which case the number of parameters often exceeds 10 dimensions. This significantly increases the search space, making it difficult to determine the parameters rationally. To resolve this issue, we previously reported an FF parameter determination approach based on meta-heuristics (APL Materials, 8, 081111 (2020)). In this study, we further investigated a methodology to efficiently determine the FF parameters using a conditional variational autoencoder (CVAE), a type of deep learning method, which can reduce the dimension of the input parameters and distribute them in a probabilistic manner in the latent space. As a demonstration, we applied the method to the optimization of 11 FF parameters for an argyrodite-type Li7PS6 material, which has attracted considerable attention as a solid electrolyte for all-solid-state batteries. The results confirmed that the proposed approach can generate valid FFs that are highly consistent with the results of first-principles calculations, even when using a limited set of meta-heuristics-generated FF parameters.
更多查看译文
关键词
potential parameters,optimization,force-field
AI 理解论文
溯源树
样例
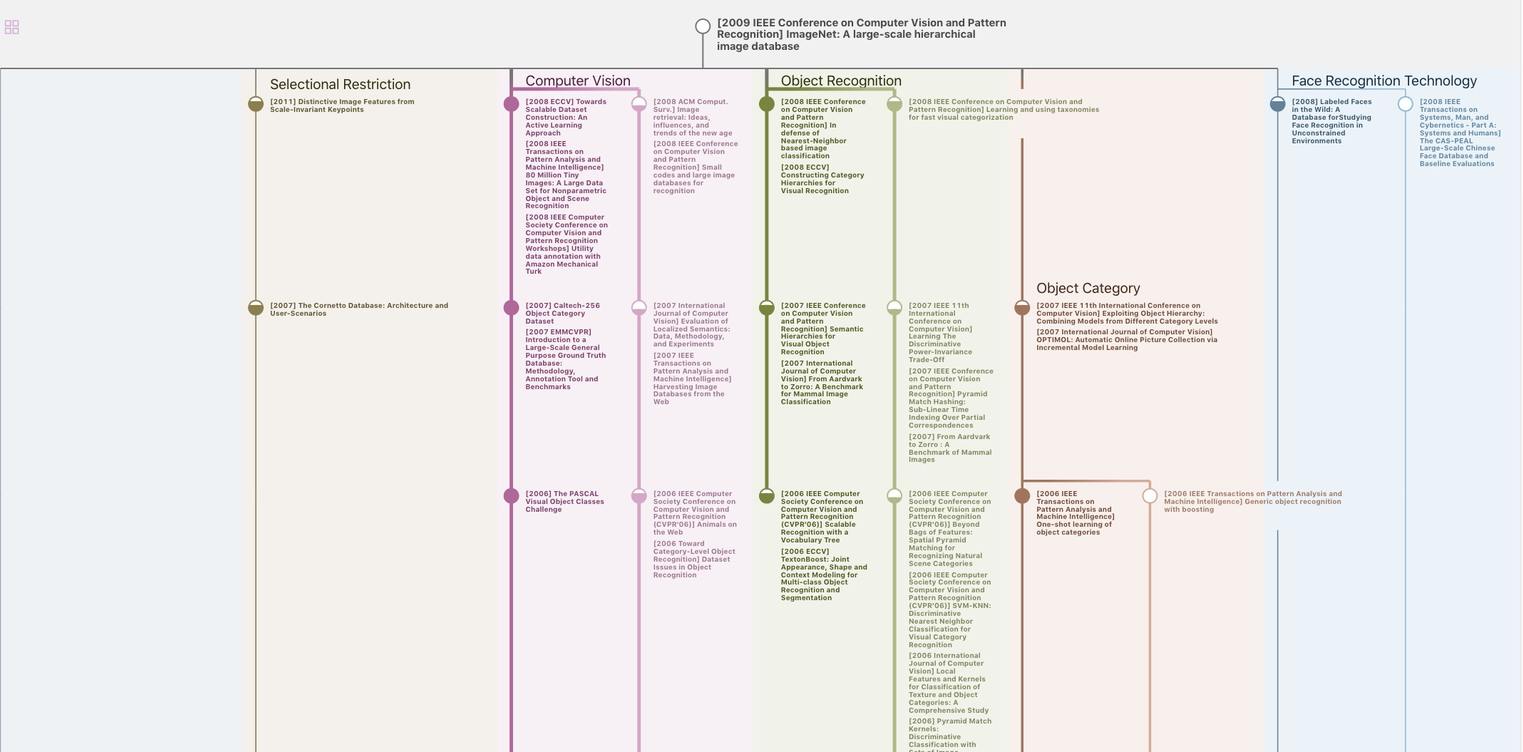
生成溯源树,研究论文发展脉络
Chat Paper
正在生成论文摘要