P1244: an automated quantification algorithm for evaluating total metabolic tumor volume in patients with fdg-avid lymphomas using a deep learning model
HemaSphere(2023)
摘要
Topic: 20. Lymphoma Biology & Translational Research Background: Total metabolic tumor volume (TMTV) holds promise as a method for quantifying tumor burden in patients with F-18 fluorodeoxyglucose (FDG)-avid lymphomas. The TMTV metric is associated with survival outcomes in patients with FDG-avid lymphomas and, with further validation, has potential as a prognostic biomarker to inform clinical decisions. We have developed a deep learning model for the automatic detection of lesions and quantification of TMTV from FDG-positron emission tomography/computed tomography (FDG-PET/CT) scans (Jemaa et al., 2020 & 2022). Aims: This study aims to further evaluate the model and identify clinical and technical factors that may influence the performance of lesion detection and TMTV quantification in patients with diffuse large B-cell lymphoma (DLBCL) and follicular lymphoma (FL). Methods: The test data set was compiled using baseline and post-treatment FDG-PET/CT scans from the phase 3 GOYA (NCT01287741) and GALLIUM (NCT01332968) clinical trials, which included 166 patients with DLBCL and 201 patients with FL, respectively. The model was trained using an independent data set from GOYA (n = 836). FDG-PET/CT images were assessed manually (mTMTV) and by the algorithm (aTMTV). Pearson’s correlation coefficient (r) was used to determine the overall performance of aTMTV versus mTMTV. Bias was assessed using the slope and intercept from a weighted Deming regression. Lesion detection performance was evaluated based on sensitivity (the proportion of mTMTV-detected lesions identified by aTMTV) and positive predictive value (PPV; the proportion of aTMTV-detected lesions identified by mTMTV). Performance was compared among patient populations with different demographics and clinical characteristics, and across images from different PET/CT scanner manufacturers. Results: aTMTV quantification highly correlated with mTMTV in the combined data set (n = 367; r, 0.96 [95% confidence interval (CI): 0.95, 0.96]; Figure A) and in the subgroup analysis by lymphoma subtype (DLBCL: r, 0.98 [95% CI: 0.97, 0.98]; FL: r, 0.93 [95% CI: 0.91, 0.95]). No systematic bias was observed between aTMTV and mTMTV in the combined data set (slope, 1.06 [95% CI: 1.02, 1.09]; intercept, –0.27 [95% CI: –0.52, –0.03]; mean difference between methods, 0.10 [standard deviation (SD): 1.15]) or the DLBCL subgroup (slope, 1.01 [95% CI: 0.97, 1.05]; intercept, –0.26 [95% CI: –0.60, 0.03]; mean difference between methods, –0.17 [SD: 0.93]). Compared with mTMTV, the algorithm slightly overestimated TMTV in FL (slope, 1.14 [95% CI: 1.09, 1.21]; intercept, –0.4 [95% CI: –0.75, –0.06]; mean difference between methods, 0.4 [SD: 1.83]). Agreement between aTMTV and mTMTV was consistent among patients with different baseline demographics and clinical characteristics, and across scans from different PET/CT scanner manufacturers. Overall mean sensitivity and PPV for lesion detection were both > 0.8 (Figure B). Performance was lower for lesions ≤ 10 mL (mean sensitivity, 0.67; mean PPV, 0.72) than for lesions > 10 mL (mean sensitivity and PPV > 0.95). Summary/Conclusion: The aTMTV algorithm demonstrated good performance for the measurement of TMTV in patients with non-Hodgkin lymphoma, while showing good generalizability across lymphoma subtypes, patient subpopulations and PET/CT scanner manufacturers. Reduced algorithm performance for small lesions (≤ 10 mL) may be the result of higher variability among readers in the determination of small lesions; future work aims to refine and optimize performance of the algorithm for use as a prognostic tool in clinical practice.Keywords: Artificial intelligence, Lymphoma, Tumor, FDG-PET
更多查看译文
关键词
total metabolic tumor volume,tumor volume,deep learning,automated quantification algorithm,fdg-avid
AI 理解论文
溯源树
样例
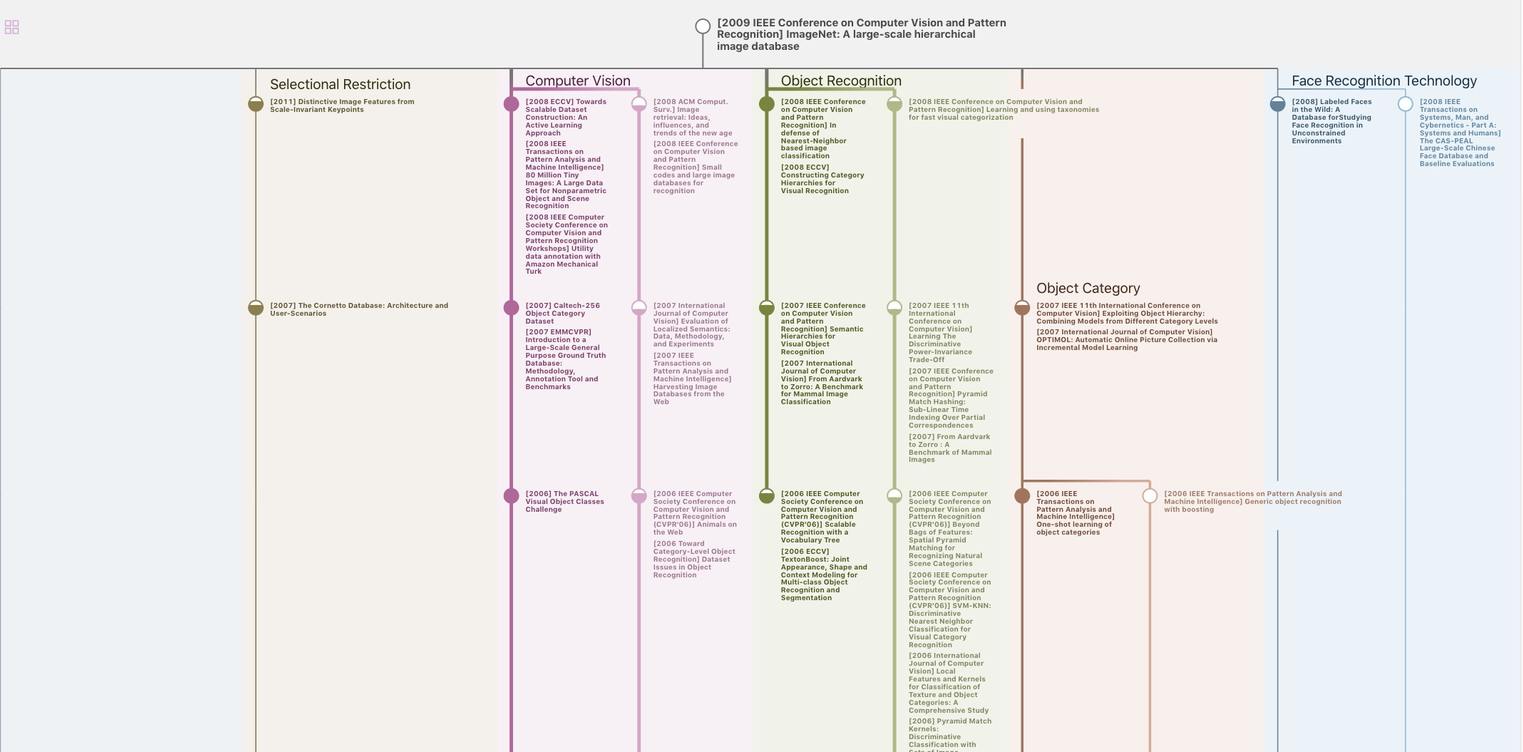
生成溯源树,研究论文发展脉络
Chat Paper
正在生成论文摘要