From Mechanism to Application: Decrypting Light-Regulated Denitrifying Microbiome Through Geometric Deep Learning
IMETA(2024)
关键词
denitrification,graph neural networks,meta-omics,microbiomes,optogenetics
AI 理解论文
溯源树
样例
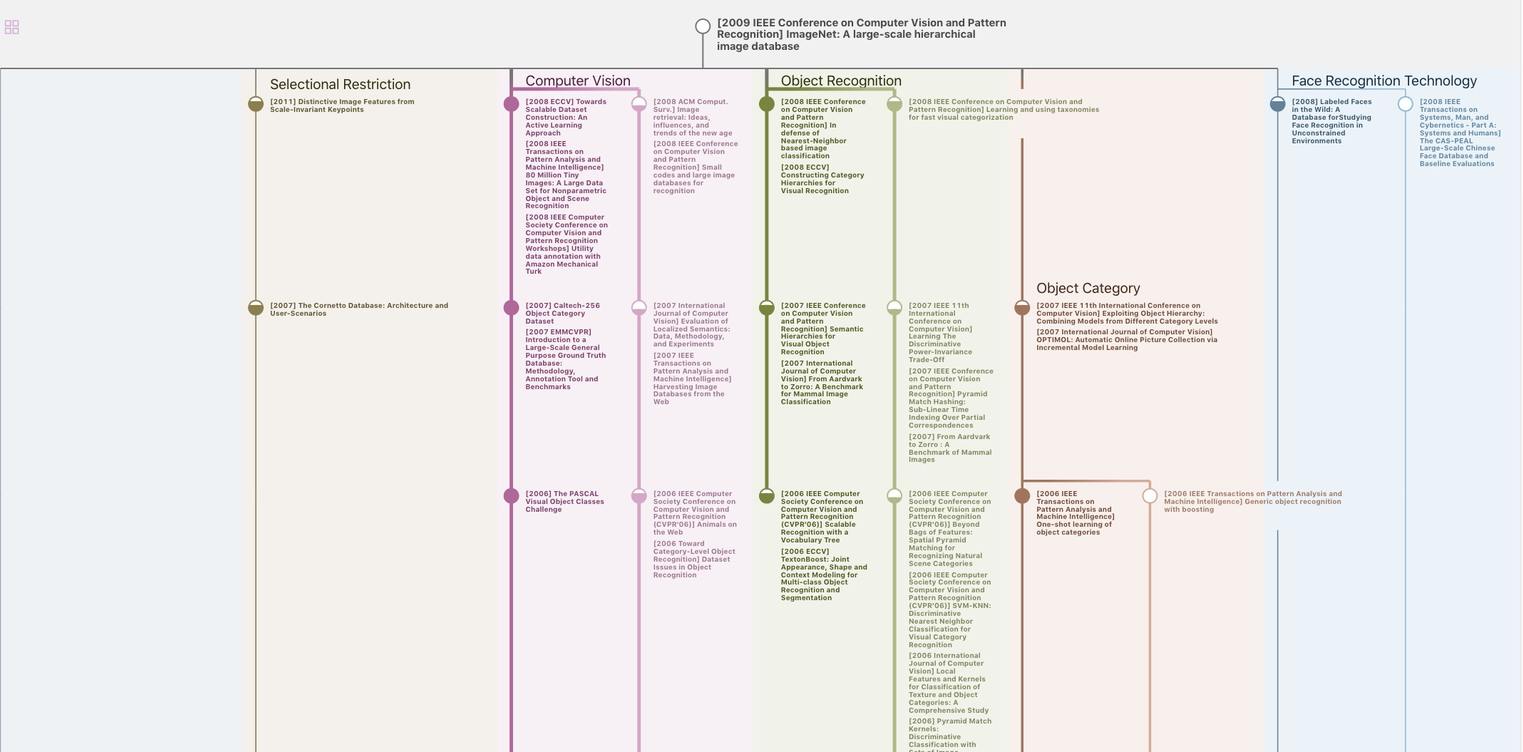
生成溯源树,研究论文发展脉络
Chat Paper
正在生成论文摘要