Mp-453083-7 prediction of post-infarction ventricular tachycardia from ct images using interpretable graph network
Heart Rhythm(2023)
摘要
Scar heterogeneity is the substrate of reentrant ventricular tachycardia (VT) after myocardial infarction (MI). In the context of ablation, LV wall thickness (WT) from CT was shown to correlate with the reentrant circuit. To build an AI classification model to predict the presence/absence of post-MI VT from WT distribution on CT, and to leverage on model interpretability to also localize arrhythmogenic sites. We included consecutive patients with history of MI who underwent CT between 2010 and 2020. Automated LV wall segmentation, reorientation and WT computation were applied to obtain 3D WT maps of the LV. An average mesh was used as a template to ensure point-to-point correspondence between input datasets. The population was divided into a training (3/4) and a testing set (1/4). On the training set, a graph convolutional network with index pooling (GCN-Pooling) model was trained to predict history of VT. For each prediction, integrate gradients were used to generate interpretability heat maps, indicating the regions most influential in the model decision. The generalisability of the model was assessed on the test population and compared to that of other clinical variables. In a subset of test cases with available high density electroanatomical maps of entire VT circuits, hot spots on interpretability maps were compared to actual sites of circuit re-entry. 600 patients were included (age 73±12 years, 83% males, LVEF 45%±13), including 167 (27.5%) with history of VT. When performing univariable analyses on the training set, VT was found to significantly relate to male gender, LVEF, scar size, and scar age, with best cut-off values of 43%, 65cm2, and 132 months for LVEF, scar size and age, respectively. When applied on the testing dataset, the GCN-Pooling model outperformed all of these risk markers, with accuracy/sensitivity/specificity values of 81%/78%/82%. In contrast, LVEF was weakly associated with VT (67%/70%/65%). A high-density activation map during VT was available in 10 patients from the testing set. The GCN-Pooling model accurately predicted the presence of VT in 9/10 patients (90%). Hot spots on interpretability maps co-localized with actual circuits in 7 of these 9 VTs (78%). The proposed GCN-pooling approach allows for a fully automated prediction of VT from CT images, while at the same time providing interpretability maps that spatially co-localize with VT circuits. This may find applications both in risk stratification and ablation guidance.
更多查看译文
关键词
prediction images,post-infarction
AI 理解论文
溯源树
样例
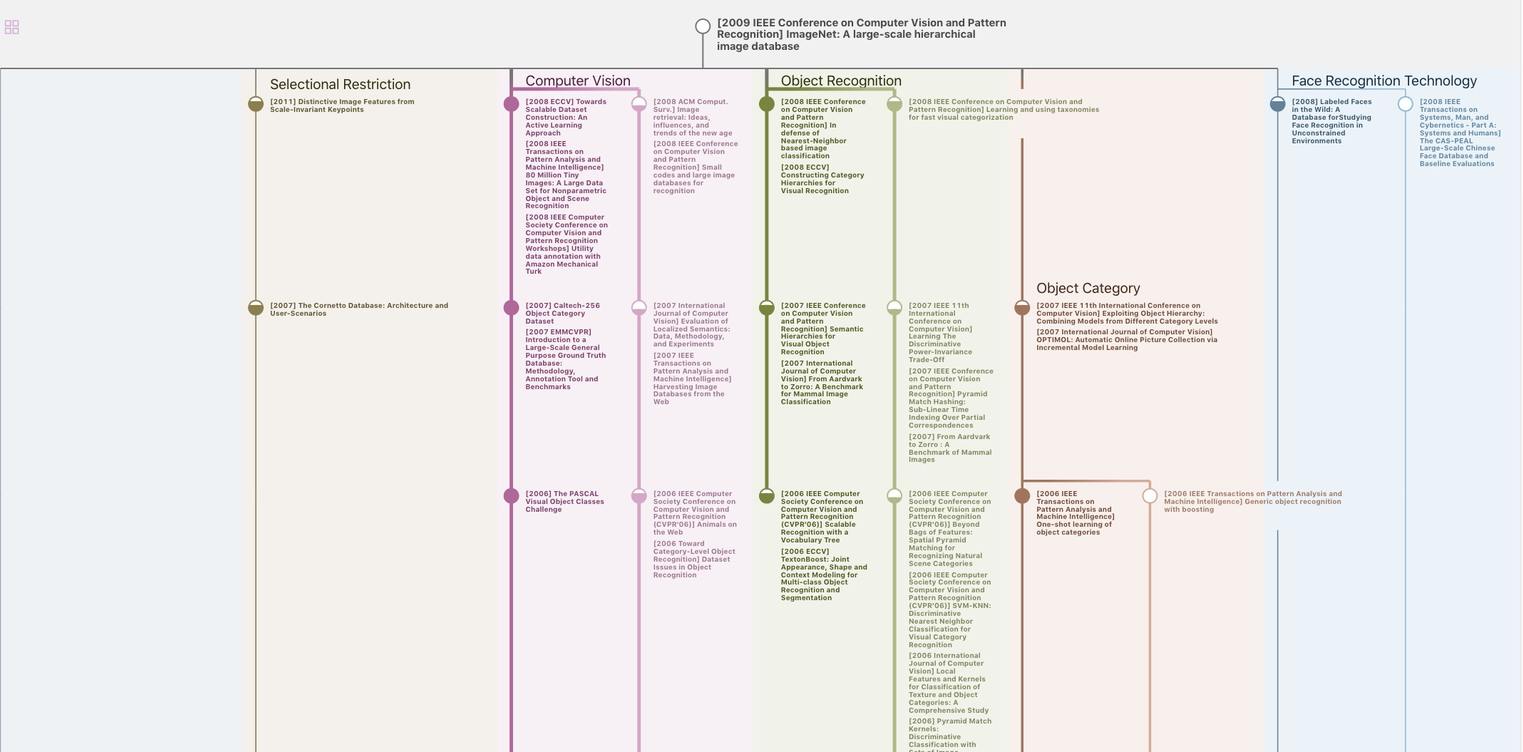
生成溯源树,研究论文发展脉络
Chat Paper
正在生成论文摘要