Unsupervised Video Domain Adaptation with Masked Pre-Training and Collaborative Self-Training
CVPR 2024(2024)
Abstract
In this work, we tackle the problem of unsupervised domain adaptation (UDA)
for video action recognition. Our approach, which we call UNITE, uses an image
teacher model to adapt a video student model to the target domain. UNITE first
employs self-supervised pre-training to promote discriminative feature learning
on target domain videos using a teacher-guided masked distillation objective.
We then perform self-training on masked target data, using the video student
model and image teacher model together to generate improved pseudolabels for
unlabeled target videos. Our self-training process successfully leverages the
strengths of both models to achieve strong transfer performance across domains.
We evaluate our approach on multiple video domain adaptation benchmarks and
observe significant improvements upon previously reported results.
MoreTranslated text
AI Read Science
Must-Reading Tree
Example
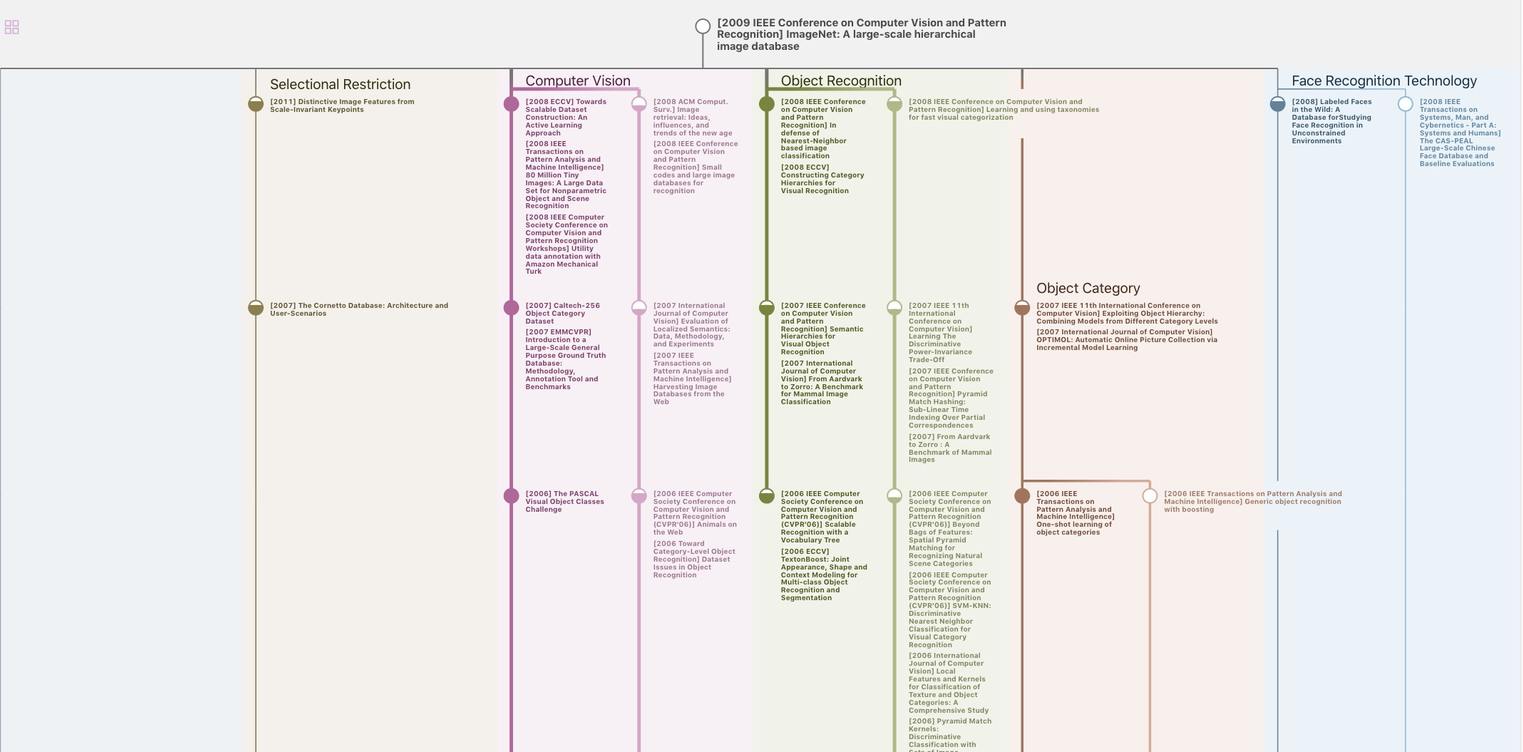
Generate MRT to find the research sequence of this paper
Chat Paper
Summary is being generated by the instructions you defined