A Kidney Tumor Segmentation Method Based on the Combination of U-Net and Transformer
2023 IEEE 6th International Conference on Pattern Recognition and Artificial Intelligence (PRAI)(2023)
摘要
According to the characteristics of kidney tumours in abdominal multi-organ CT images, analyzing the CNN network to deal with long-distance connection defects and the shortage of Transformer structure detail processing, we designed a multimodal residual continuous convolution CNN and an improved multiscale dual-path multi-head attention Transformer combined with an encoder, deconvolution up-sampling, and learnable attention gating mechanism of the decoder with a jump junction introduced into the multiscale convolutional fusion module to form an asymmetric U-Net model framework for kidney tumour segmentation. Experimental comparison results show that the proposed method achieves an average dice coefficient of 0.9352, an average cross-merge rate of 0.9894, and a class-average pixel accuracy of 0.98235 for kidney segmentation metrics, which are more accurate and detailed than the existing and newer network models for segmentation.
更多查看译文
关键词
Residual continuous convolution,multi-scale fusion block,patched dual-path,multi-head attention
AI 理解论文
溯源树
样例
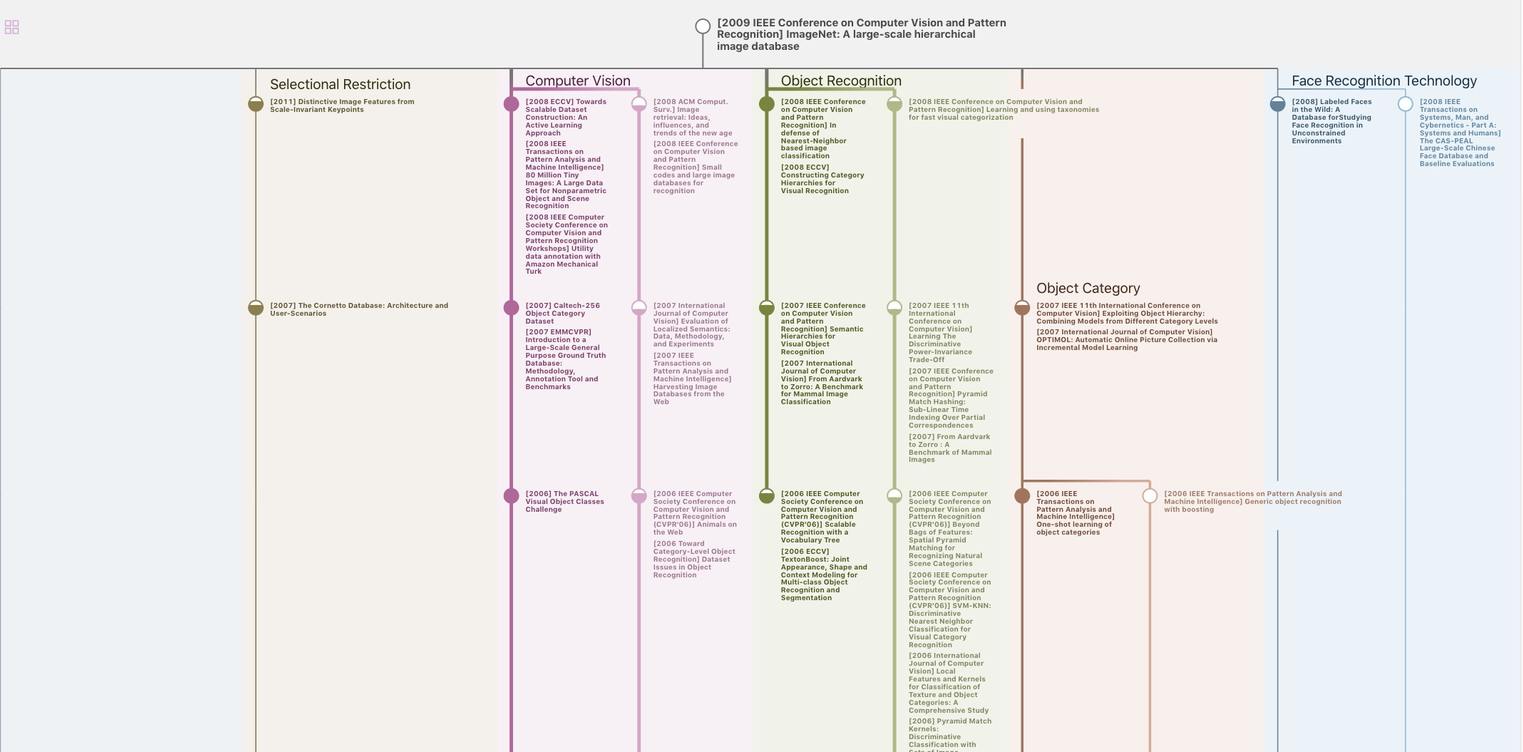
生成溯源树,研究论文发展脉络
Chat Paper
正在生成论文摘要