Federated Learning for Privacy-aware Cognitive Workload Estimation
MUM '23: Proceedings of the 22nd International Conference on Mobile and Ubiquitous Multimedia(2023)
摘要
Human physiological monitoring has become easily accessible by integrating wearable devices into our lives, providing valuable real-time data. Methods for Cognitive Workload (CW) estimation utilize such physiological data to quantify CW during task execution. These methods are crucial for various domains, including mobile healthcare, forecasting human errors, and human-machine interaction. However, accurately estimating CW continues to pose a challenge due to the absence of objective ground truth data, context dependency, and the privacy sensitivity of the data. This study tackled the complex task of estimating CW based on privacy-sensitive data (e.g., eye movement, pupil diameter, blink information, and other physiological signals) using Federated Learning (FL) methods to improve user privacy. We compared the outcomes of the FL models with the more conventional centralized approach on two publicly-available datasets COLET and ADABase, which include data from 75 participants overall. The results highlight the efficacy of FL in collaboratively training a global (person-independent) model. The FL models achieved performances on par with centralized state-of-the-art models while preserving data privacy. Recognizing the importance of privacy in user sensing, FL presents a promising approach that enables wearable sensing applications in privacy-sensitive domains.
更多查看译文
AI 理解论文
溯源树
样例
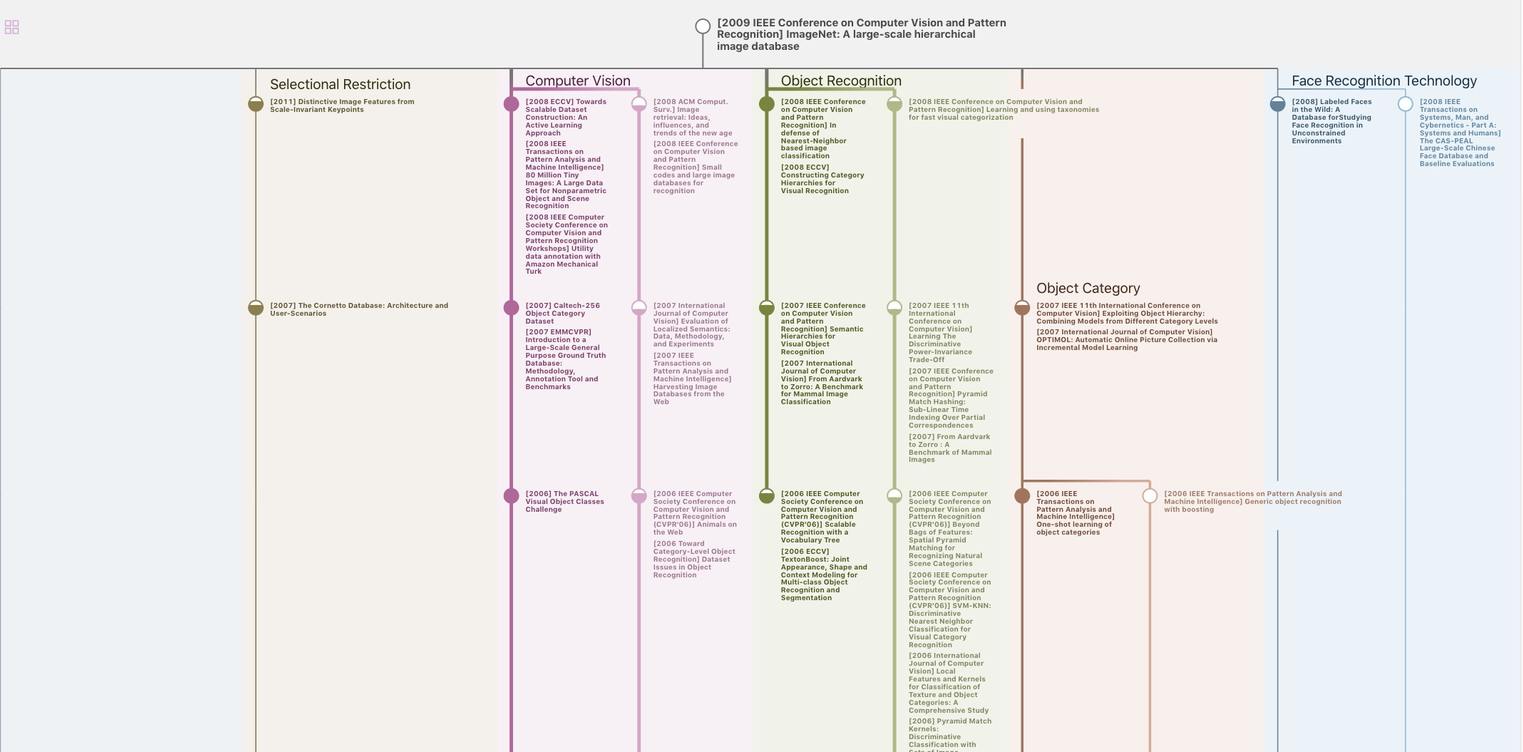
生成溯源树,研究论文发展脉络
Chat Paper
正在生成论文摘要