A flexible-predefined-time convergence and noise-suppression ZNN for solving time-variant Sylvester equation and its application to robotic arm
CHAOS SOLITONS & FRACTALS(2024)
摘要
Although the zeroing neural network (ZNN) has strong competitiveness in solving the time -varying Sylvester equation (TVSE), its convergence and robustness as two key indicators still need to be further improved. To address these problems, we propose a flexible -predefined -time convergence and noise -suppression ZNN (FPCNS-ZNN). Different from most existing ZNN variants, the proposed FPCNS-ZNN provides the following three new improvements: (1) In FPCNS-ZNN, a new specially designed time -variant -gain (SD-TVG) is proposed to better improve the convergence speed at each stage of iterative computation. (2) In FPCNS-ZNN, a new flexible nonlinear activation function (FN -AF) is proposed to flexibly control the convergence speed and predefine convergence time with certain robustness. (3) SD-TVG and FN -AF are dexterously combined to construct the new FPCNS-ZNN, which can obtain faster convergence speed and stronger robustness. Through detailed theoretical analysis and mathematical derivation, the proposed FPCNS-ZNN model has been confirmed to have promising stability, flexible -predefined -time, and strong robustness. It should be noted that the predefined -time upper bound of the proposed FPCNS-ZNN is accurately calculated by dexterously using the Beta function. Computer simulations and comparative experiments indicate that the proposed FPCNS-ZNN has faster convergence speed and stronger robustness compared with three state-of-the-art ZNN variants. Finally, the proposed FPCNS-ZNN is used to solve the motion planning problem of the robotic arm, and the experiment results illustrate the utility of the model.
更多查看译文
关键词
Time-variant Sylvester equation,Zeroing neural network,Convergence,Robustness
AI 理解论文
溯源树
样例
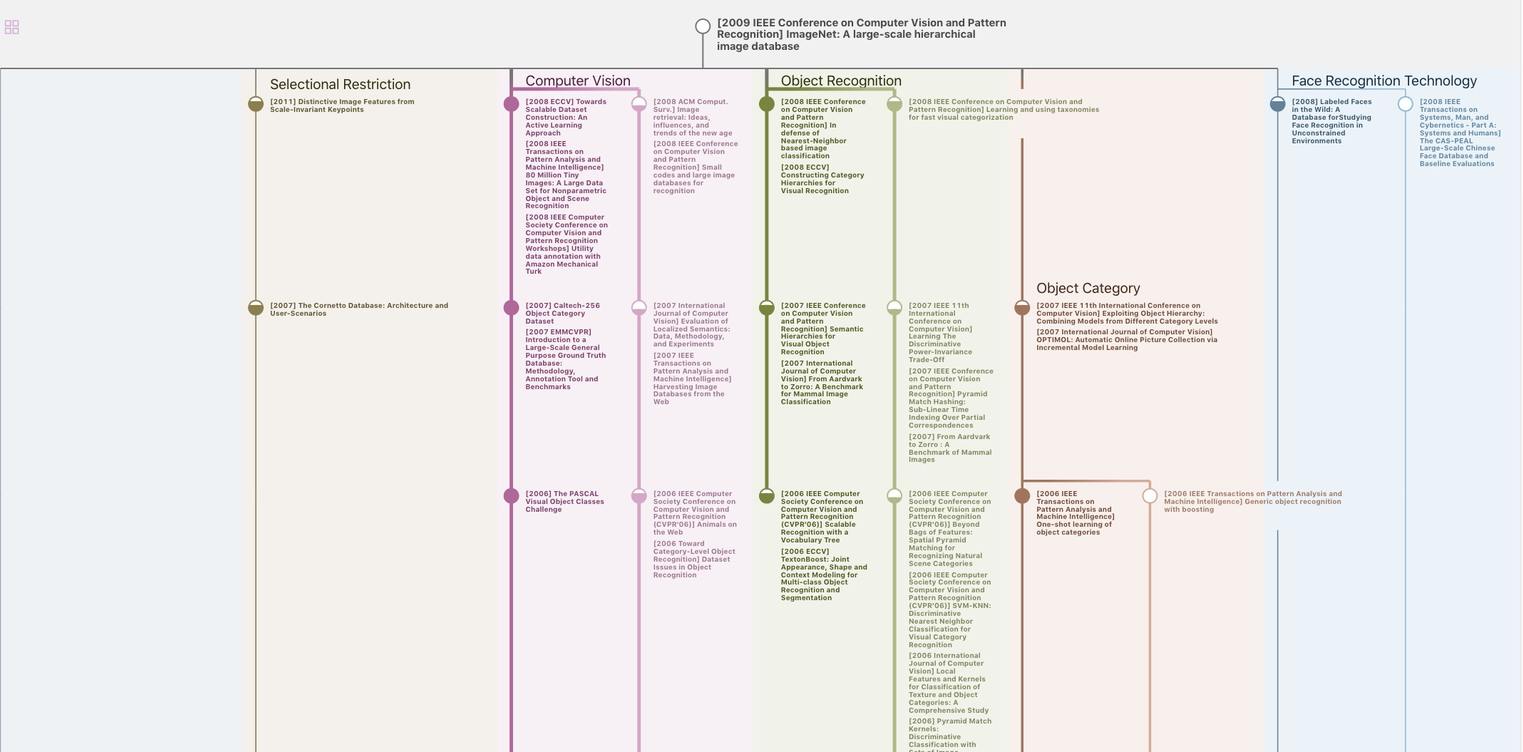
生成溯源树,研究论文发展脉络
Chat Paper
正在生成论文摘要