YOLOPX: Anchor-free multi-task learning network for panoptic driving perception
PATTERN RECOGNITION(2024)
摘要
Panoptic driving perception encompasses traffic object detection, drivable area segmentation, and lane detection. Existing methods typically utilize anchor-based multi-task learning networks to complete this task. While these methods yield promising results, they suffer from the inherent limitations of anchor-based detectors. In this paper, we propose YOLOPX, a simple and efficient anchor-free multi-task learning network for panoptic driving perception. To the best of our knowledge, this is the first work to employ the anchor-free detection head in panoptic driving perception. This anchor-free manner simplifies training by avoiding anchor-related heuristic tuning, and enhances the adaptability and scalability of our multi-task learning network. In addition, YOLOPX incorporates a novel lane detection head that combines multi-scale high-resolution features and long-distance contextual dependencies to improve segmentation performance. Beyond structure optimization, we propose optimization improvements to enhance network training, enabling our multi-task learning network to achieve optimal performance through simple end-to-end training. Experimental results on the challenging BDD100K dataset demonstrate the state-of-the-art (SOTA) performance of YOLOPX: it achieves 93.7% recall and 83.3% mAP50 on traffic object detection, 93.2% mIoU on drivable area segmentation, and 88.6% accuracy and 27.2% IoU on lane detection. Moreover, YOLOPX has faster inference speed compared to the lightweight network YOLOP. Consequently, YOLOPX is a powerful solution for panoptic driving perception problems. The code is available at https://github.com/jiaoZ7688/YOLOPX.
更多查看译文
关键词
Multi-task learning,Panoptic driving perception,Autonomous driving,Anchor-free
AI 理解论文
溯源树
样例
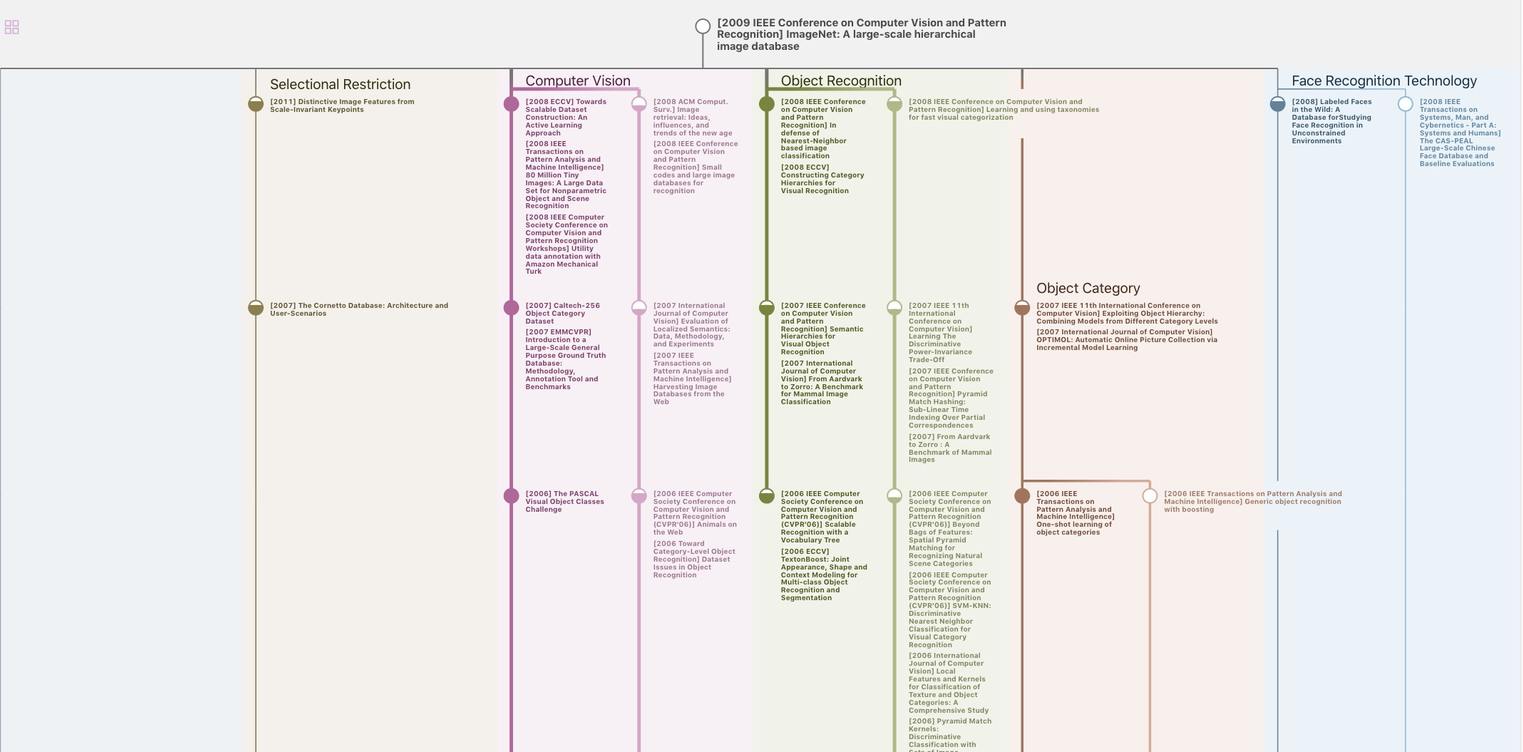
生成溯源树,研究论文发展脉络
Chat Paper
正在生成论文摘要