Progressive network based on detail scaling and texture extraction: A more general framework for image deraining
NEUROCOMPUTING(2024)
摘要
Many feature extraction components have been proposed for image deraining tasks, aiming to improve feature learning. However, few models have addressed the integration of multi-scale features from derain images. The fusion of multiple features at different scales in one model has the potential to significantly enhance the authenticity and detail of rainy images restoration. This study introduces a migratable multi-scale feature blending model, which is a progressive learning model based on detail dilation and texture extraction. First, the degraded image is sent to the detail dilation module, which is designed to increase the detailed outline and obtain the coarse image features. Second, the extracted feature maps are sent to the multi-scale feature extraction (MFE) module and the multi-scale hybrid strategy (MHS) module for improved texture restoration. Third, the simple convolution modules are replaced by an optimized transformer model to more efficiently extract contextual features and multi-scale information in images. Finally, a progressive learning strategy is employed to incrementally restore the degraded images. Empirical results show that our proposed module for progressive restoration achieves near state-of-the-art performance in several rain removal tasks. In particular, our model exhibits better rain removal realism compared to state-of-the-art models. The source code is available at https://github.com/JackAILab/DTPNet.
更多查看译文
关键词
Image deraining,Transformer,Multi-scale feature blending,Progressive learning
AI 理解论文
溯源树
样例
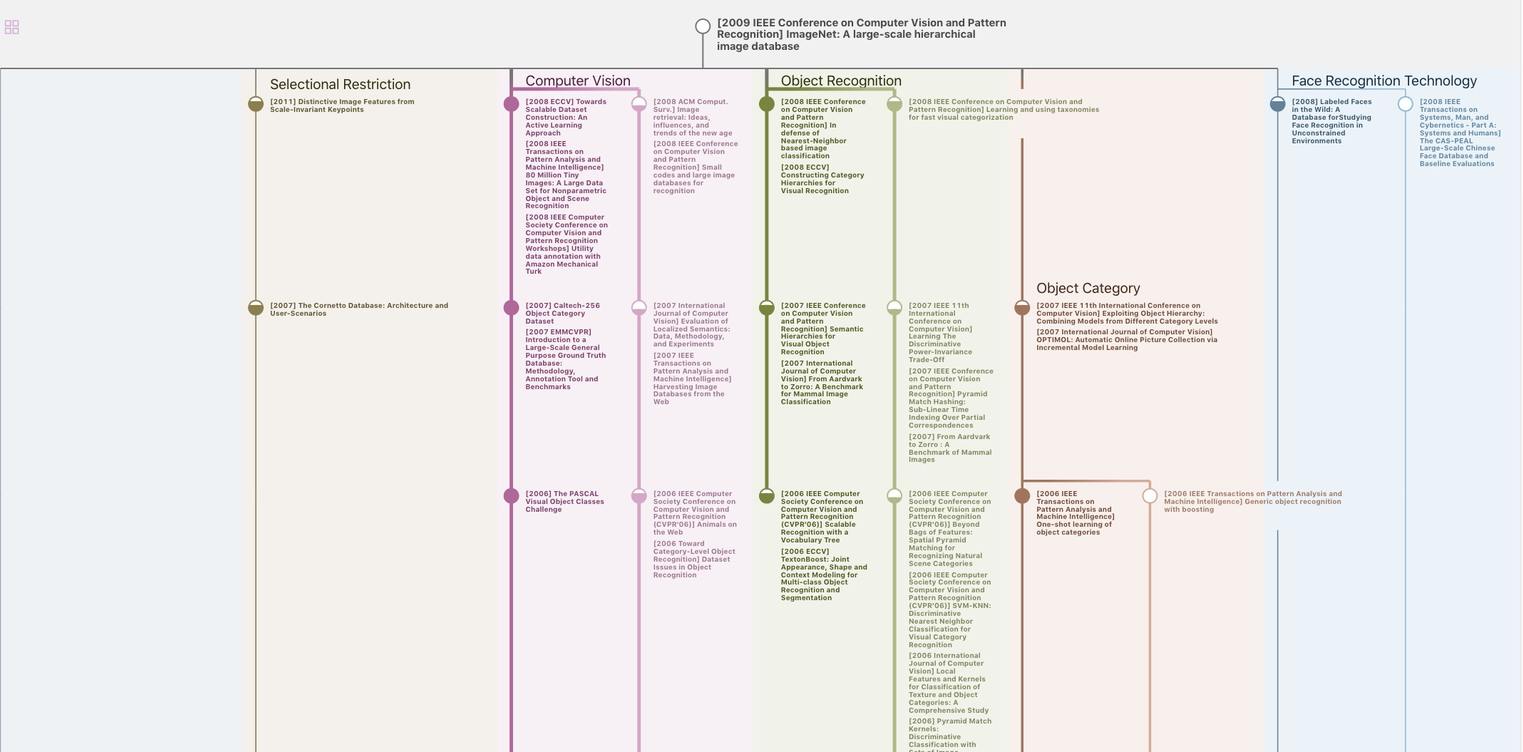
生成溯源树,研究论文发展脉络
Chat Paper
正在生成论文摘要