Rapid health estimation of in-service battery packs based on limited labels and domain adaptation
JOURNAL OF ENERGY CHEMISTRY(2024)
摘要
For large-scale in-service electric vehicles (EVs) that undergo potential maintenance, second-hand trans-actions, and retirement, it is crucial to rapidly evaluate the health status of their battery packs. However, existing methods often rely on lengthy battery charging/discharging data or extensive training samples, which hinders their implementation in practical scenarios. To address this issue, a rapid health estima-tion method based on short-time charging data and limited labels for in-service battery packs is proposed in this paper. First, a digital twin of battery pack is established to emulate its dynamic behavior across various aging levels and inconsistency degrees. Then, increment capacity sequences (4Q) within a short voltage span are extracted from charging process to indicate battery health. Furthermore, data-driven models based on deep convolutional neural network (DCNN) are constructed to estimate battery state of health (SOH), where the synthetic data is employed to pre-train the models, and transfer learning strategies by using fine-tuning and domain adaptation are utilized to enhance the model adaptability. Finally, field data of 10 EVs exhibiting different SOHs are used to verify the proposed methods. By using the 4Q with 100 mV voltage change, the SOH of battery packs can be accurately estimated with an error around 3.2%.(c) 2023 Science Press and Dalian Institute of Chemical Physics, Chinese Academy of Sciences. Published by ELSEVIER B.V. and Science Press. All rights reserved.
更多查看译文
关键词
Lithium -ion battery,Electric vehicles,Health estimation,Feature extraction,Convolutional neural network,Domain adapatation
AI 理解论文
溯源树
样例
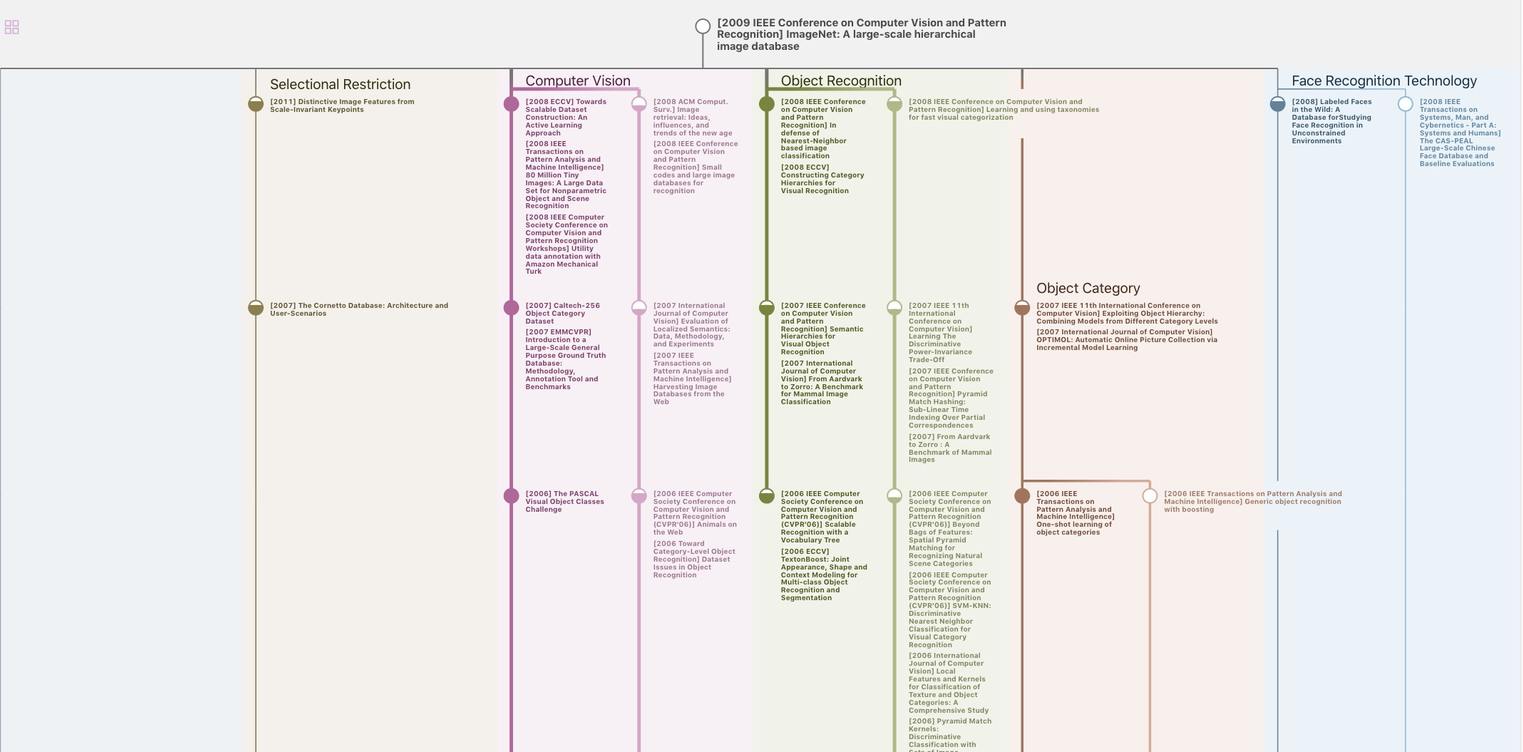
生成溯源树,研究论文发展脉络
Chat Paper
正在生成论文摘要