Human Activity Recognition based on Local Linear Embedding and Geodesic Flow Kernel on Grassmann manifolds
EXPERT SYSTEMS WITH APPLICATIONS(2024)
摘要
Human Activity Recognition (HAR) plays a crucial role in various applications(e.g., medical treatment, video surveillance and sports monitoring). Transfer learning is a promising solution to cross-domain identification problems in HAR. However, existing methods usually ignore the negative transfer caused by using the features of each source domain in equal proportions, as well as the distribution difference between the source and target domains. In this paper, an HAR method based on manifold learning is proposed. Firstly, the similarity between the domain and multiple source domains is calculated using the Multi-Kernel-Maximum Mean Difference (MK-MMD), and the source domain most similar to the target domain is selected as the optimal source domain in the transfer task. Secondly, Locally Linear Embedding (LLE) is leveraged to reduce the dimensionality of both optimal source domain and target domain data to remove redundant information, and the Geodesic Flow Kernel (GFK) is utilized to project low-dimensional data into the Grassmann manifold space and reduce the distribution difference between the two domains. Finally, the source domain action training model is applied to the target domain. Three public datasets (i.e., PAMAP2, OPPORTUNITY and UCI DSADS) are utilized to validate the effectiveness of the proposed approach. Experimental results are presented to demonstrate that the proposed HAR method can predict a large number of unlabeled samples in the target domain while preserving the original data structure.
更多查看译文
关键词
Human Activity Recognition,Transfer learning,Locally Linear Embedding,Geodesic Flow Kernel,Grassmann manifolds
AI 理解论文
溯源树
样例
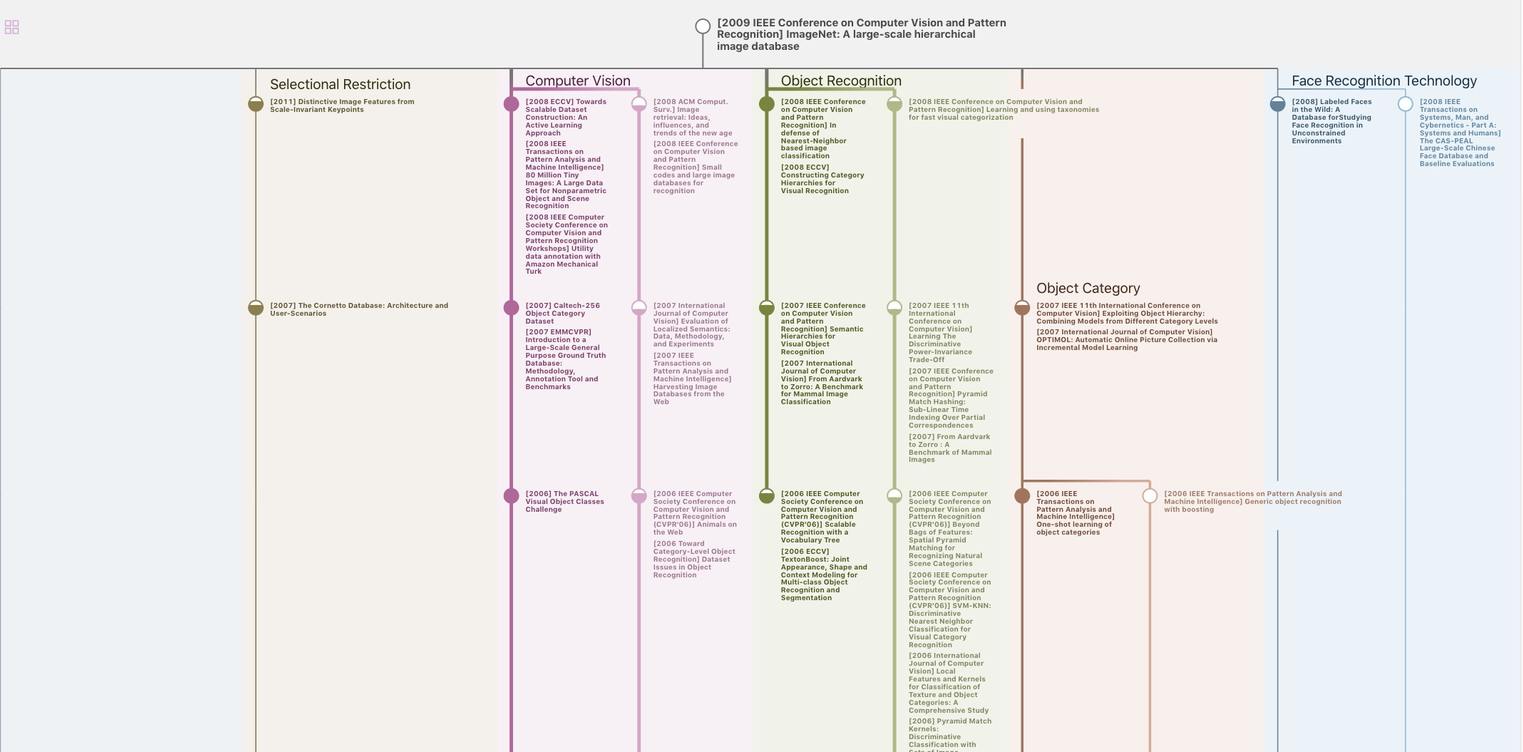
生成溯源树,研究论文发展脉络
Chat Paper
正在生成论文摘要