Novel technique for the preparation and analysis of powder-based polished sections by automated optical mineralogy: Part 2-Use of deep learning approach for transparent mineral detection
MINERALS ENGINEERING(2024)
摘要
Automated reflected light optical microscopy represents an alternative and affordable technique compared to automated scanning electron microscopy for quantitative mineralogical analysis. Polished sections are the commonly used sample analysis format, and it is essential to obtain unbiased mineralogical quantification results from those. However, automated optical microscopy does not allow the detection of transparent minerals during reflected light analysis, as the reflectivity of the resin and these minerals is very similar.This work aims to propose an innovative way to automatically detect all particles and minerals, including transparent minerals, in polished sections with acrylic resin using reflected light optical imaging and a deep learning algorithm. To do so, several ore powders and standard mineral mixes were mounted into acrylic resin polished sections at two different particle sizes: < 1 mm and P80 similar to 75 mu m. Optical images of these polished sections were acquired with an automated reflected light optical microscope to train and test the deep learning algorithm to detect mineral particles. The results suggest that the deep learning algorithm easily detected all mineral particles in the acrylic resin matrix, allowing all minerals particles (including transparent minerals) to be well-differentiated under reflected light optical microscopy, and providing an unbiased mineralogical quantification.
更多查看译文
关键词
Polished section preparation,Optical reflected light microscopy,Epoxy resins,Quantitative automated mineralogy,Deep learning
AI 理解论文
溯源树
样例
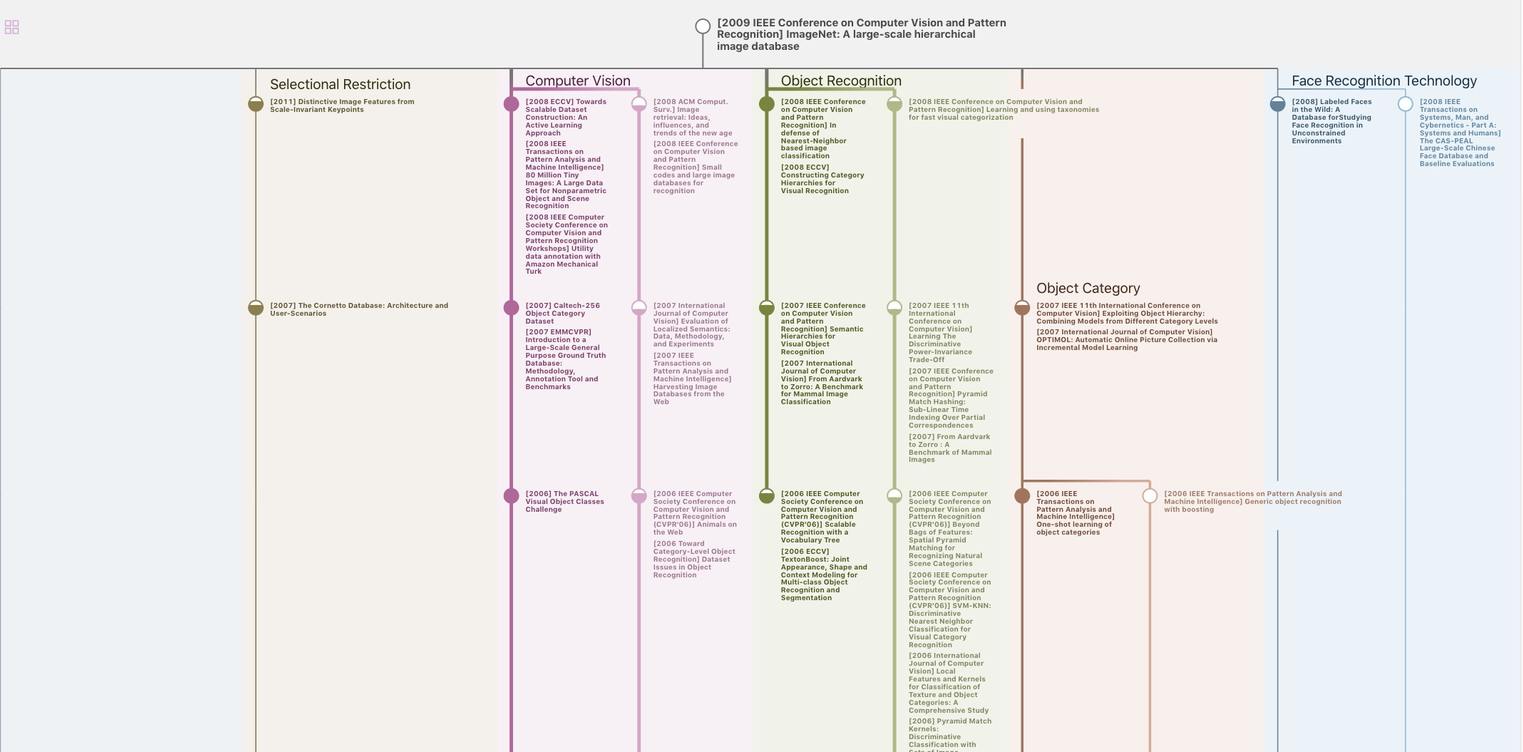
生成溯源树,研究论文发展脉络
Chat Paper
正在生成论文摘要