Hyperbolic embedding of discrete evolution graphs for intelligent tutoring systems
EXPERT SYSTEMS WITH APPLICATIONS(2024)
摘要
Intelligent tutoring systems (ITS) have received much attention recently as online learning has taken off and is replacing offline instruction in many cases. It analyses user behavior and customizes personalized learning strategies for users through artificial intelligence technology. ITS encompasses a variety of entities and multiple relations, making it suitable to be represented as a graph. This perfectly aligns with the utilization of graph embedding (GE) for downstream ITS tasks. Existing GE methods cannot effectively model ITS data because the user evolution in ITS is discrete in time. The patterns of variation in user states are similar to each other but not correlated at the temporal level. Because of the hierarchical structure caused by the discrete evolution, encoding ITS data in a hyperbolic space is more sensible. We define a discrete evolution graph (DEG) to characterize ITS and propose a method called DEGE to embed it. The static nodes in a DEG are projected randomly and then transformed into hyperbolic space. Next, employ hyperbolic evolution networks to generate the embedding of dynamic nodes. The aggregated features of each node are then delivered by hyperbolic aggregation networks and are concatenated to generate the final higher-order features. To validate the superiority, design a multi-objective loss function with preserving pairwise proximity and preserving link types to train the model on several real datasets. The experimental results demonstrate that our method outperforms other baselines on both question annotation and performance prediction in ITS.
更多查看译文
关键词
Intelligent tutoring systems,Graph embedding,Dynamic graph,Hyperbolic embedding
AI 理解论文
溯源树
样例
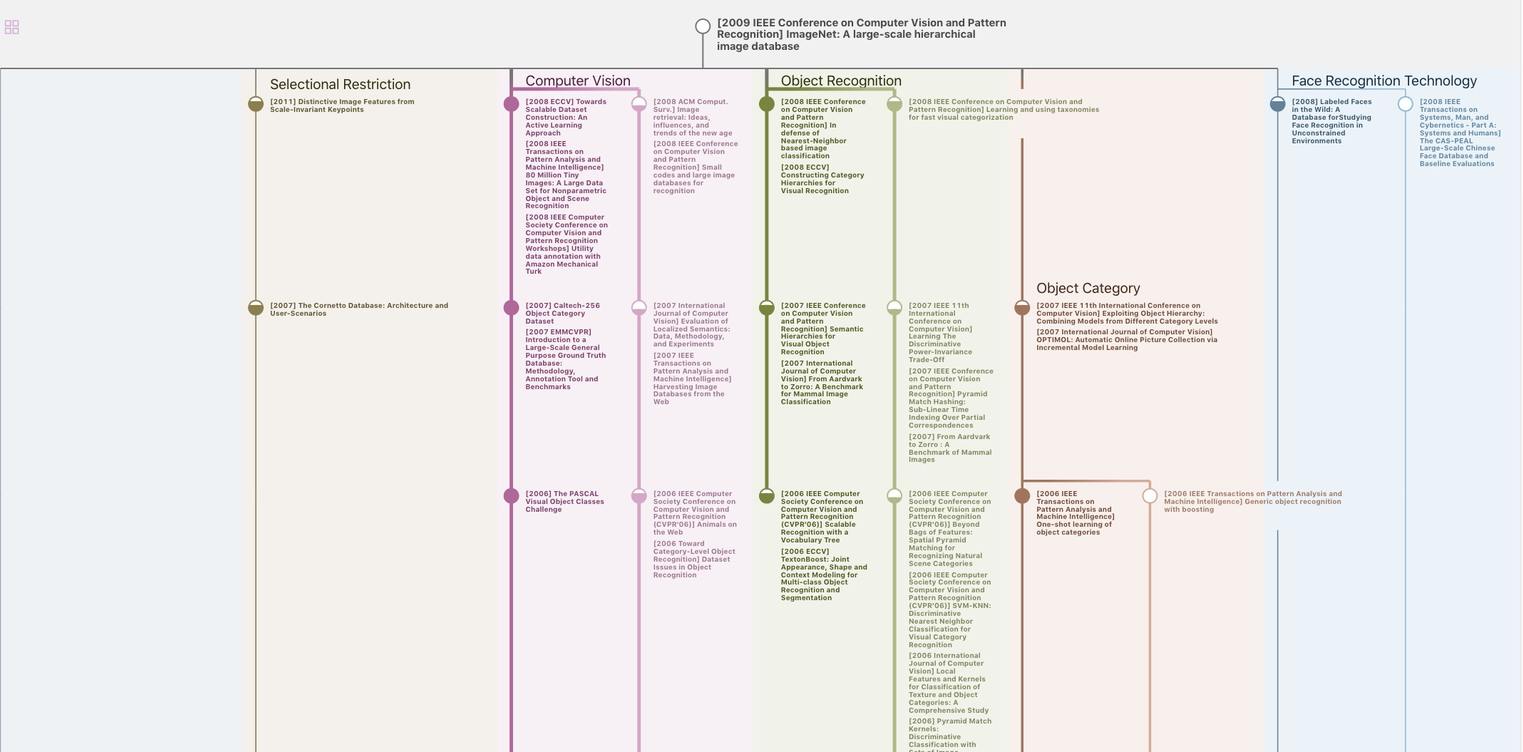
生成溯源树,研究论文发展脉络
Chat Paper
正在生成论文摘要