Machine Learning Applied to EIT: Image Reconstruction, Displaced Electrodes and Boundary Shape
IFAC PAPERSONLINE(2023)
摘要
Electrical Impedance Tomography (EIT) is a noninvasive, nonradioactive technique used to obtain an internal image of a body or object. This work addresses several EIT-related problems using Artificial Neural Networks (ANNs). The EIT method consists of performing measurements of electrical potentials in a set of electrodes while different patterns of electrical current are applied. This work proposes the use of a modified version of LeNet-5 to determine the conductivity distribution within the domain, resulting in an image of the interior of the boundary in which the electrodes are positioned. There are two sources of errors in the usage of EIT in medical images. The first one is the displacement of the electrodes, which are supposed to be equidistant; and the second one is the modification of the boundary shape. The human body is not rigid, and due to breathing the boundary shape changes, leading to the displacement of electrodes. A modified version of LeNet-5 is also used to determine the changes of the boundary shape, and classifiers, such as Support Vector Machine (SVM), Random Forest and K-nearest Neighbors (KNN), are used to determine the displaced electrodes. In this work, all methods are used apart. Then, the reconstruction algorithm does not yet consider these sources of errors. Copyright (c) 2023 The Authors. This is an open access article under the CC BY- NC-ND license (https://creativecommons.org/licenses/by-nc-nd/4.0/)
更多查看译文
关键词
EIT,CNN,SVM,random forest,KNN
AI 理解论文
溯源树
样例
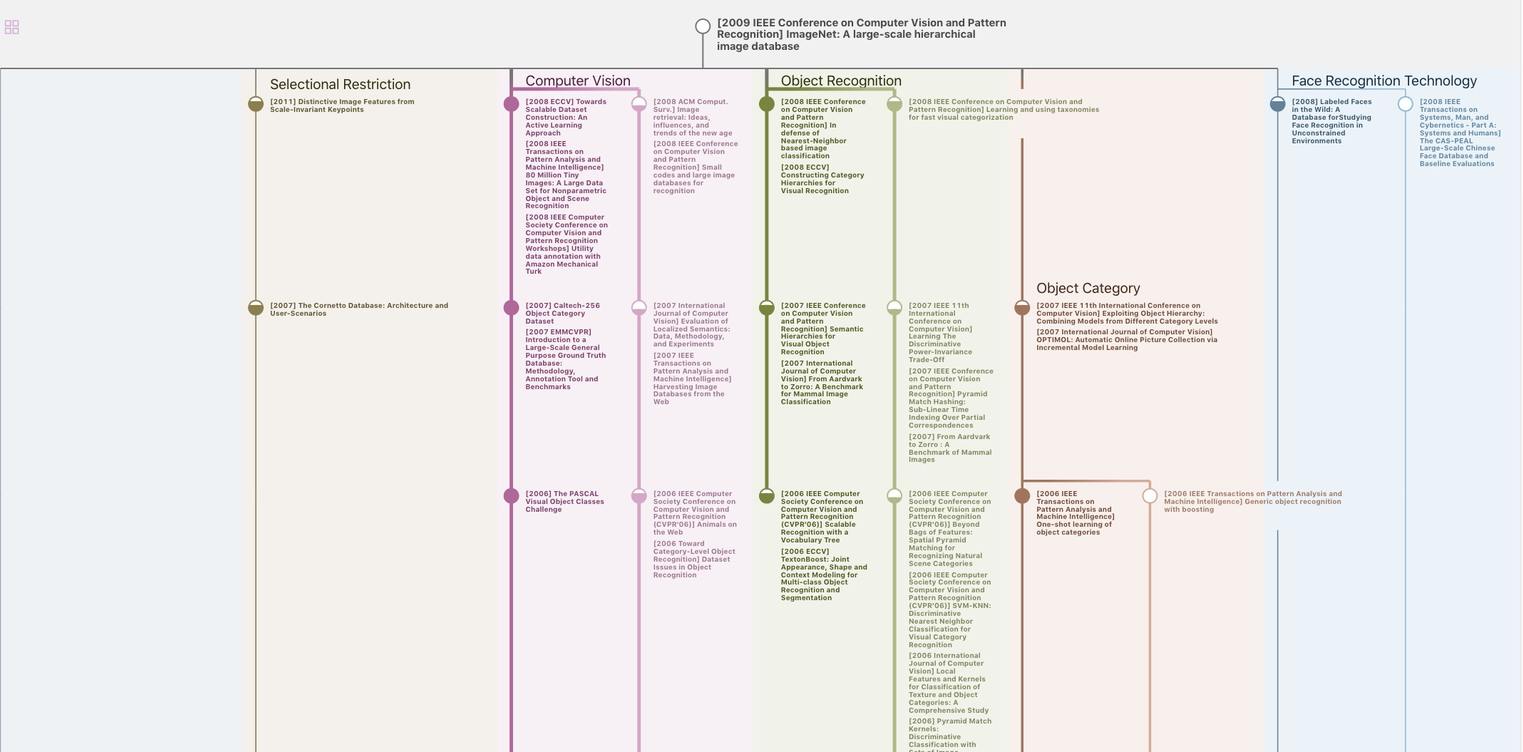
生成溯源树,研究论文发展脉络
Chat Paper
正在生成论文摘要