Stability in Reinforcement Learning Process Control for Additive Manufacturing
IFAC PAPERSONLINE(2023)
摘要
Reinforcement Learning (RL), as a machine learning paradigm, receives increasing attention in both academia and industry, in particular for process control. Its trial-and-error concept, along with its data-driven nature, make RL suitable for process control in complex tasks, where the control task and framework can be formulated flexibly. However, there are still challenges that need to be addressed in order for RL to be introduced into the control mainstream, in particular for critical processes. A major challenge in RL is that there is no guarantee for robust, stable RL process control. This is a key impediment to RL implementation on tasks for which stability is an important requirement. Additive Manufacturing (AM) is an example of such process control task, since the very high complexity of the manufacturing process makes it suitable for RL process control, while stable performance is a necessity. However, one has to firstly understand performance and stability as a key requirement. In this paper, we reflect on stability approaches in RL and we investigate the stability requirements for AM. Our AM case study provides intuition and encourages further research for stable RL that would unlock potential for adoption and implementation of RL in AM applications. Research in the proposed direction would also have the potential for impacts to other process control sectors, in critical applications, where appropriate utilisation of stable RL control could bring significant advantages. Copyright (c) 2023 The Authors.
更多查看译文
关键词
Reinforcement learning and deep learning in control,Robust control,Lyapunov methods,Additive manufacturing
AI 理解论文
溯源树
样例
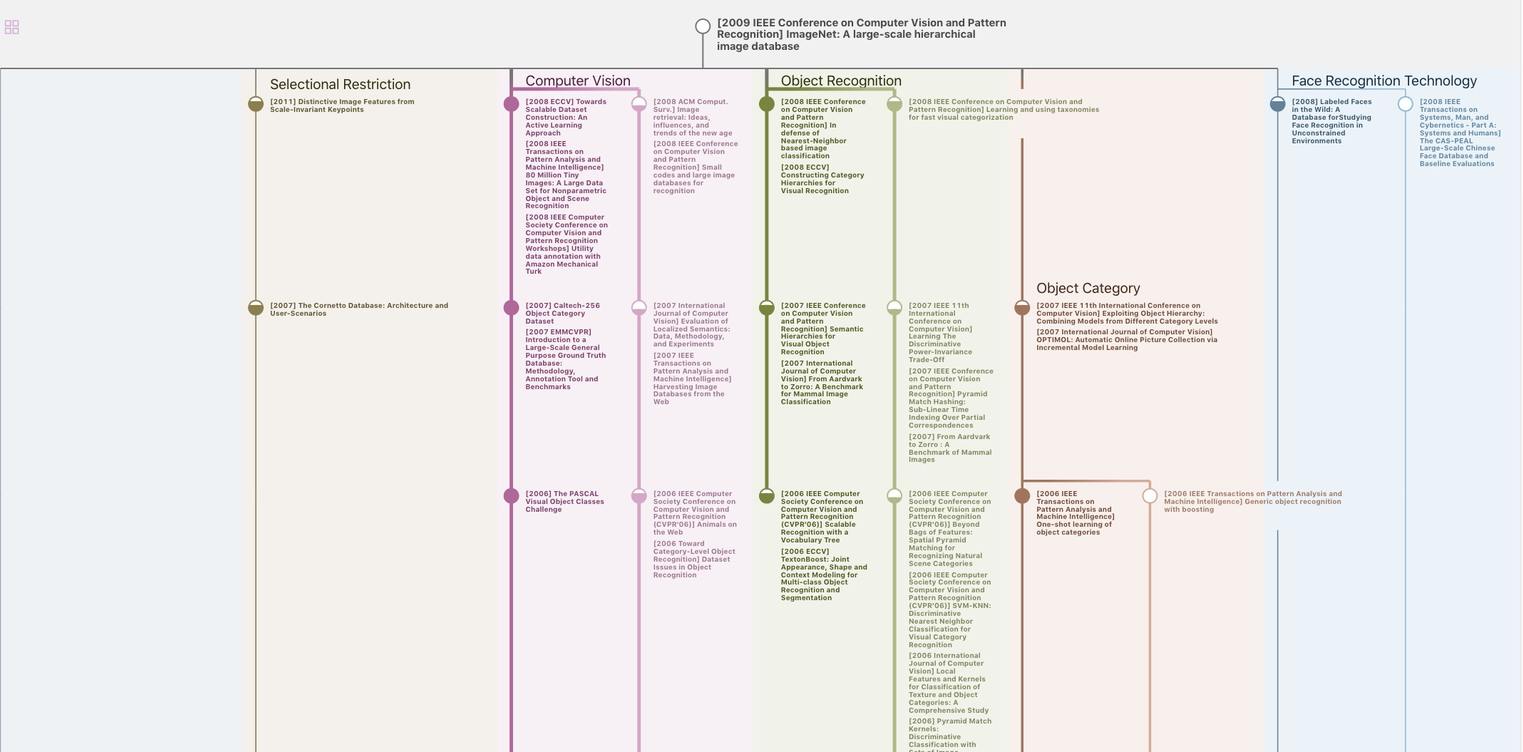
生成溯源树,研究论文发展脉络
Chat Paper
正在生成论文摘要