A novel framework for motor bearing fault diagnosis based on multi-transformation domain and multi-source data*
KNOWLEDGE-BASED SYSTEMS(2024)
摘要
Through the application of deep learning and multi-sensor data, fault features can be automatically extracted and valuable information can be integrated to tackle intricate challenges in motor bearing fault diagnosis. Most existing fusion models focus primarily on the original time series signal with information extraction largely restricted to the time domain (without extensions into multiple transformation domains). Also, most fusion models, the sensor fusion level is kept relatively simple which could lead to the oversight correlations and complementarities among the information. To enhance the recognition capability of diagnostic network features, in this paper, we propose a novel framework for motor bearing fault diagnosis from perspectives of multi-transformation domain and multi-source data fusion. Within this framework, feature extraction and fusion from various source data are achieved in the time domain, frequency domain, and frequency domain. Distinct independent networks are set up within these domains: one network is designated for overseeing feature fusion, while the others are dedicated to extracting features from individual sensors. To support the extraction of pivotal features across multiple fusion layers in various transformation domains, several fusion nodes are inserted between the layers of the multiple feature extraction networks and the feature summarization network. Furthermore, a channel attention mechanism is introduced as a fusion strategy serves to pinpoint the significance of different features, thus enhancing the efficiency of feature extraction. Experimental evaluation reveals the efficacy of the proposed model and highlights its noteworthy performance attributes such as scalability and universality.
更多查看译文
关键词
Fault diagnosis,Rolling bearing,Multi-transformation,Multi-source data,Deep learning,Attentional mechanisms
AI 理解论文
溯源树
样例
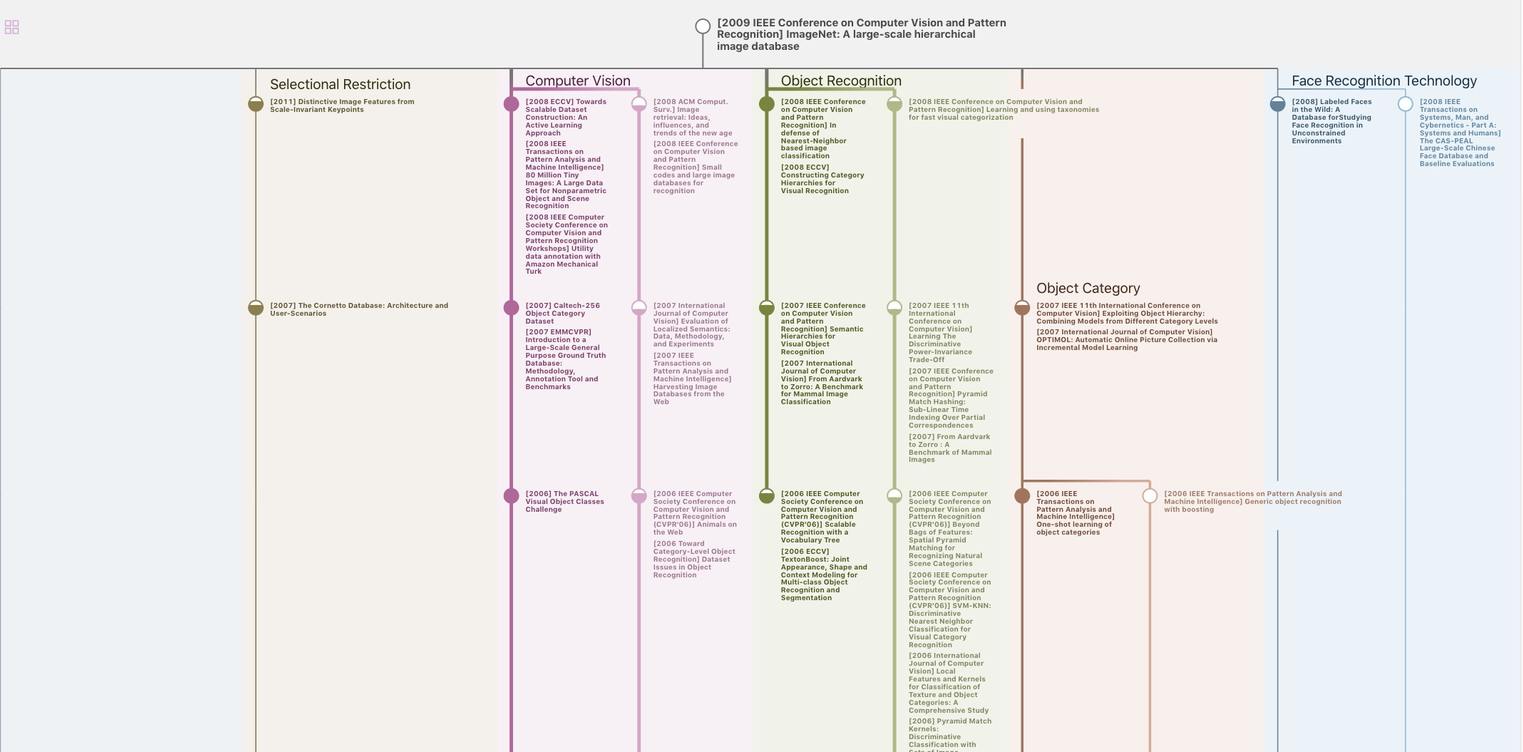
生成溯源树,研究论文发展脉络
Chat Paper
正在生成论文摘要