Designing an AI purchasing requisition bundling generator
COMPUTERS IN INDUSTRY(2024)
摘要
Following the design science methodology, a recommender system has been created with the research objective of finding a novel approach to the bundling problem in order to generate data-driven insights identifying cost potentials across an organization. In this study, a concept that has been implemented in business-to-business marketing at IBM is taken over to procurement in the automotive industry to provide decision support. Thereby, this work builds on information processing theory to utilize artificial intelligence technologies, i.e., natural language processing and supervised learning to augment the skills of buyers, whereby design principles were derived for information technology providers and procurement organizations in private and public settings worldwide. As a key finding, overall, Mini Batch K-means was the most performative model among the selected clustering algorithms. Furthermore, through actively making use of purchasing requisition data typically available in enterprise resource planning systems, the bundling generator artifact can lead to significant improvements in strategic planning and commodity management resulting in better utilization of buyer's capacities, tender designs, and thus procurement's value contribution in the transformation toward industry 4.0. The empirical study contributes to the literature on bundling and spend analysis, which has predominantly relied on historical data. By incorporating requisition data, which poses inherent challenges of precision and information-richness, this work expands this traditional approach with a forward-looking perspective.
更多查看译文
关键词
Bundling problem,Automotive case study,Design science methodology,Purchasing and supply management,Artificial intelligence,Industry 4.0
AI 理解论文
溯源树
样例
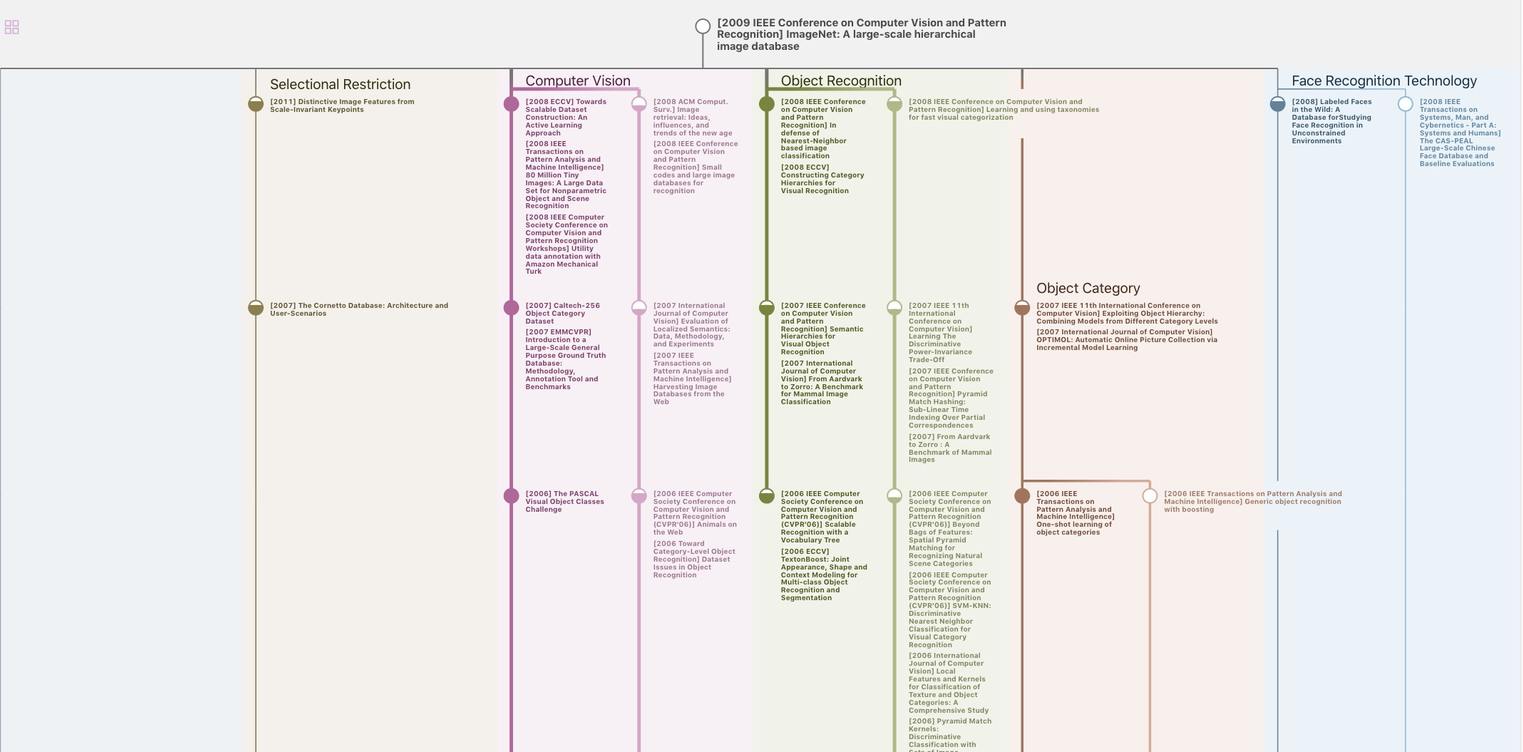
生成溯源树,研究论文发展脉络
Chat Paper
正在生成论文摘要