Performance Evaluation of Depth Completion Neural Networks for Various RGB-D Camera Technologies in Indoor Scenarios.
AI*IA(2023)
摘要
RGB-D cameras have become essential in robotics for accurate perception and object recognition, enabling robots to navigate environments, avoid obstacles, and manipulate objects precisely. Such cameras, besides RGB information, allow the capture of an additional image that encodes the distance of each point in the scene from the camera. Popular depth acquisition techniques include active stereoscopic, which triangulates two camera views, and Time-of-Flight (T-o-F), based on infrared laser patterns. Despite different technologies, none of them is yet able to provide accurate depth information on the entire image due to various factors such as sunlight, reflective surfaces or high distances from the camera. This leads to noisy or incomplete depth images. Neural network-based solutions have been researched for depth completion, aiming to create dense depth maps using RGB images and sparse depth. This paper presents a comparison of the data provided by different depth-sensing technologies, highlighting their pros and cons in two main benchmark setups. After an analysis of the sensors’ accuracy under different conditions, several state-of-the-art neural networks have been evaluated in an indoor scenario to assess if it is possible to improve the quality of the raw depth images provided by each sensor.
更多查看译文
关键词
depth completion neural networks
AI 理解论文
溯源树
样例
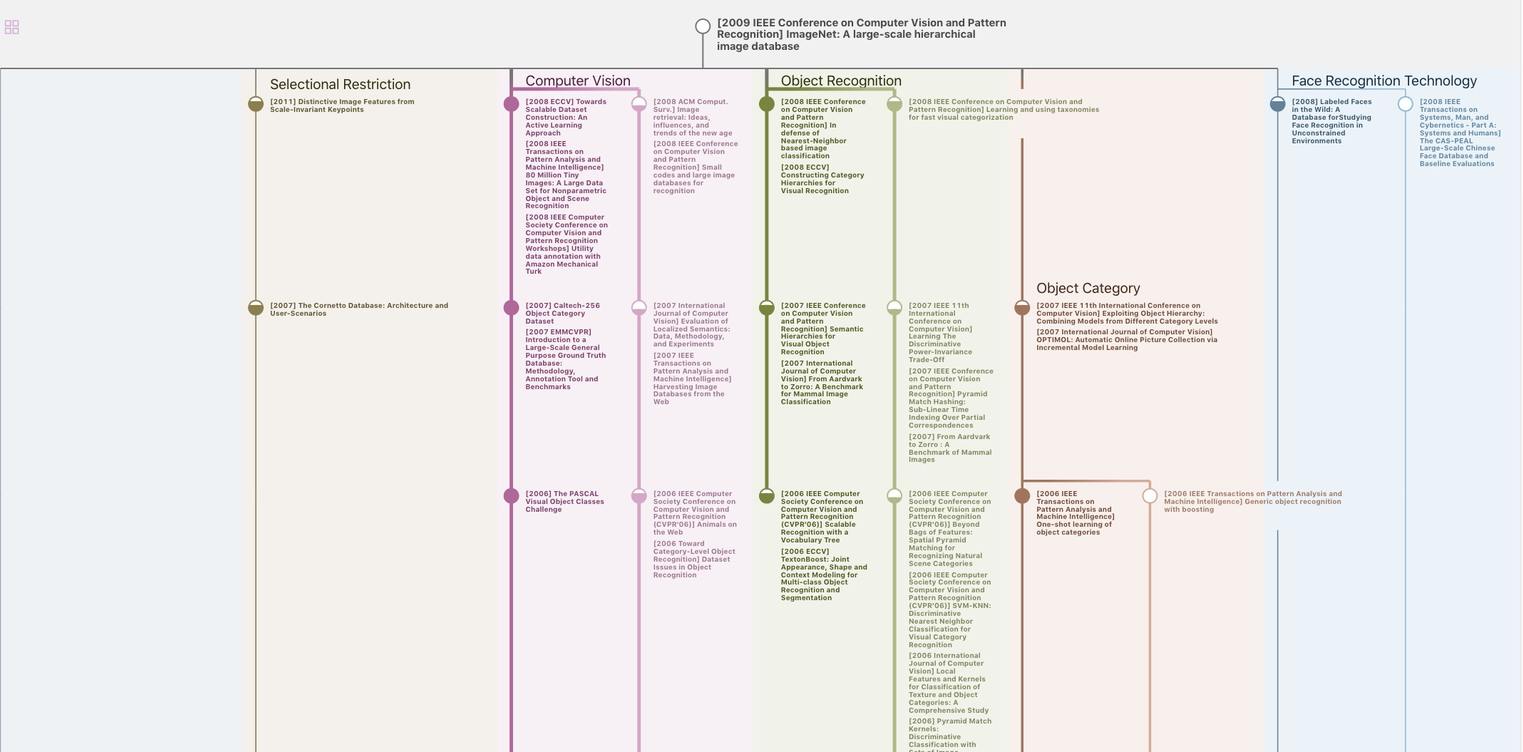
生成溯源树,研究论文发展脉络
Chat Paper
正在生成论文摘要