Variation-learning high-resolution network for capsulorhexis recognition of cataract surgery
Appl. Soft Comput.(2023)
摘要
In cataract surgery, capsulorhexis is the first, one of the most critical steps. However, recognizing the margin of rhexis during capsulorhexis is challenging and has rarely been studied due to its intraoperative invisibility, variable shape, and lack of a proprietary labeled dataset. Capsulorhexis margin recognition is essential for robot-assisted surgery, surgical assessment, and intraoperative reference. To solve the problem, this paper proposes a creative dynamic margin recognition structure called THRC, which includes a novel segmentation network, THRNet, and a compensation block. First, the dilated HRNet is designed to expand the receptive field while maintaining high-resolution representation for better detail preservation. Second, cascaded transformer blocks are proposed. The channel transformer block (CTB) is developed to include fixed and dynamic weights while generating global context dependency for nonrigid tissue variation pattern learning. The layer transformer block (LTB) with redistributed transformer variables is then developed to fuse multi-resolution features effectively for better multi-scale semantic segmentation. Moreover, the compensation block compensates for inter-frame movement by key-point registration and recovers a more realistic margin by a smoothing process. Finally, to evaluate the proposed structure, we establish the capsulorhexis dataset CapsSeg. The THRNet achieves 78.24% mean intersection over union (mIoU) and 86.59% mean Dice (mDice), making it equivalent in performance to state-of-the-art networks, but with a lower computational cost. The feasibility of the compensation block is also visualized.(c) 2023 Elsevier B.V. All rights reserved.
更多查看译文
关键词
High-resolution representations,Surgical scene segmentation,Capsulorhexis margin recognition,Transformer
AI 理解论文
溯源树
样例
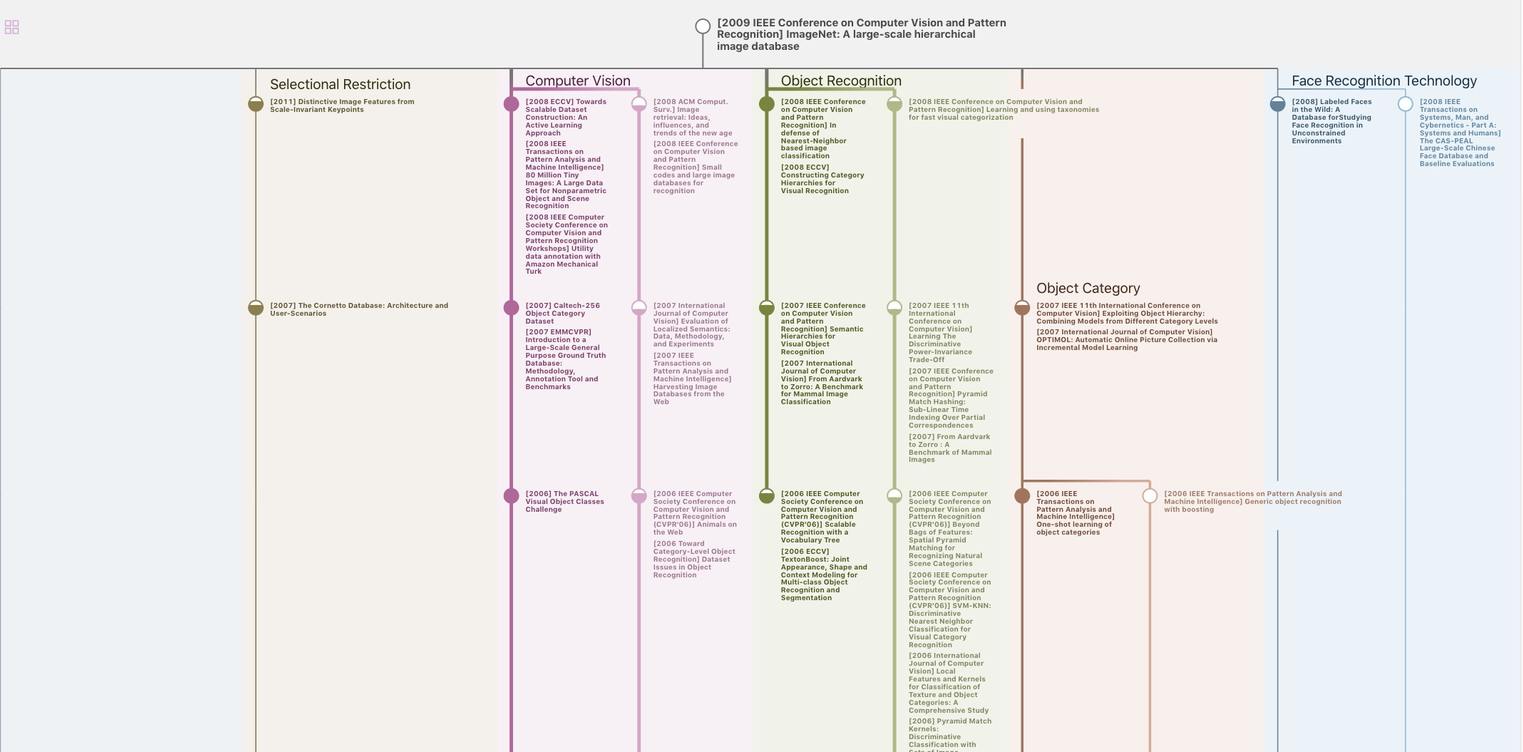
生成溯源树,研究论文发展脉络
Chat Paper
正在生成论文摘要