Asymmetric TeacherStudent Feature Pyramid Matching for Industrial Anomaly Detection
IEEE Transactions on Instrumentation and Measurement(2024)
摘要
Visual anomaly detection plays an important role in industrial product quality inspection, but the scarcity of anomaly samples makes the training of supervised models extremely challenging. Many knowledge distillation-based unsupervised detection methods have been proposed recently; however, these anomaly detection methods mostly use similar or identical symmetric structures to build teacher-student (T-S) models, which hinders the different expression of anomalies between T-S networks. To address this issue, this article proposes an asymmetric T-S feature pyramid matching network (ATSN). This network consists of a teacher network, a student network, and a lightweight feature fusion module (FFM). The teacher network is constructed with ResNet18, and the student network comprises a U-shaped network consisting of an encoder and a decoder. In the knowledge transfer process, the encoder acts as an intermediate transmitter of information, and the decoder reconstructs the information transmitted by the encoder into multiscale features. The U-shaped design can effectively improve the accuracy of knowledge transfer, thus increasing the differences in anomalous representation via T-S feature pyramid matching. Additionally, an FFM is used to fuse the correct information from the multiscale feature representation in the encoder and the decoder, thereby compensating for the loss of key information in the decoder and further increasing the difference in anomalous representation. Experiments on the MVTec AD dataset show that our proposed method surpasses the current state-of-the-art methods.
更多查看译文
关键词
Anomaly detection,asymmetric,convolutional neural network,knowledge distillation,multiscale feature fusion
AI 理解论文
溯源树
样例
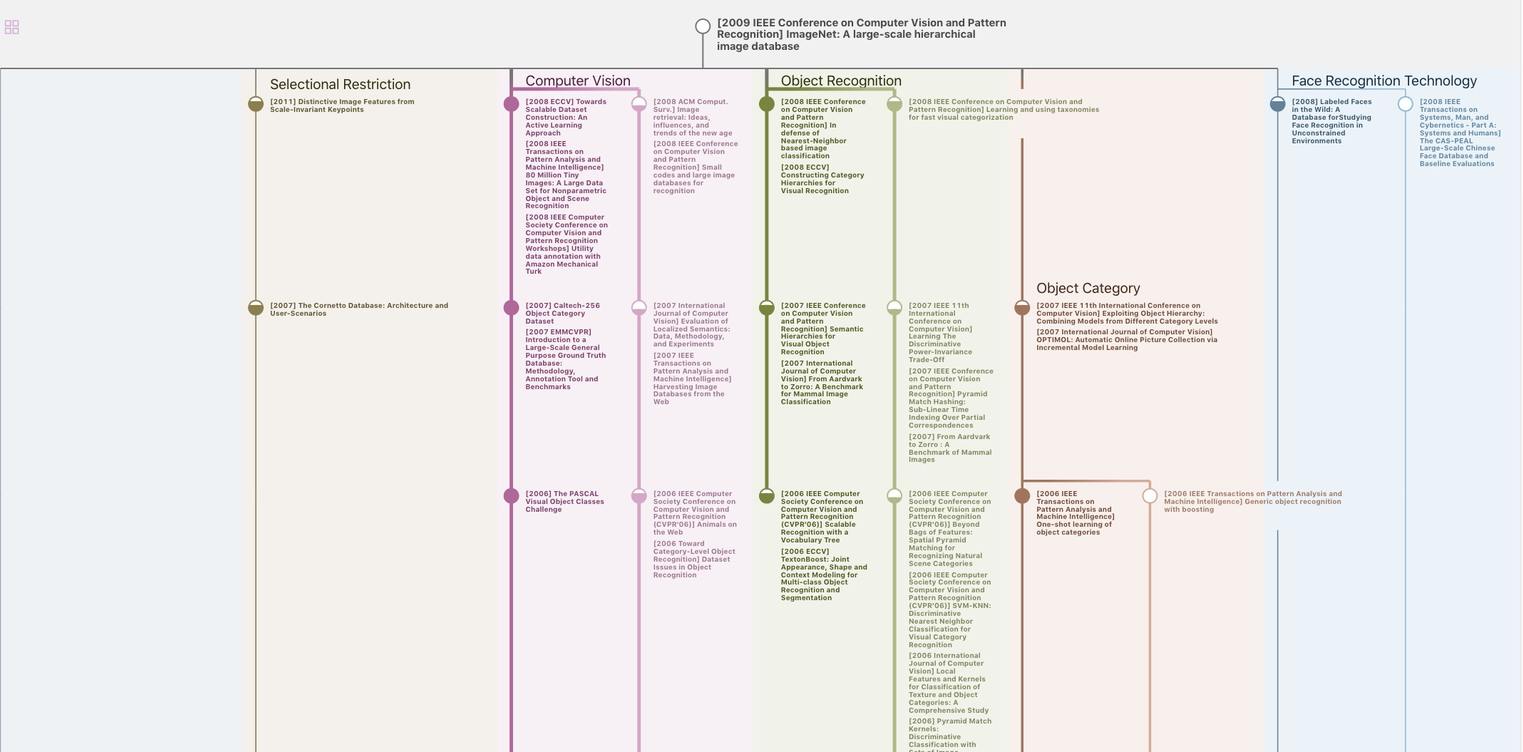
生成溯源树,研究论文发展脉络
Chat Paper
正在生成论文摘要