Learning Monocular Regression of 3D People in Crowds via Scene-Aware Blending and De-Occlusion.
IEEE Trans. Multim.(2024)
摘要
In this study, we address the challenge of estimating 3D body pose, shape, and depth relationships from single RGB images in crowded scenes. The difficulty lies in the limited availability of in-the-wild training samples, which feature densely populated scenes. To mitigate this issue, we introduce a synthesis-based approach that fuses multiple human samples into a single composite scene. Our innovative scene-aware blending technique maintains human-scene consistency by positioning individuals within plausible locations and adjusting their scales to conform to 3D settings. Furthermore, our method enables flexible per-subject occlusion management during the blending process, bolstering the robustness of 3D human body representations through a novel de-occlusion training scheme. We present a one-stage model, CBD, designed to learn monocular regression of 3D people in crowds by leveraging blending and de-occlusion techniques. Our quantitative and qualitative evaluations on four benchmark datasets reveal that CBD surpasses existing state-of-the-art approaches in terms of 3D human pose and mesh regression accuracy, thereby establishing it as a promising solution for monocular 3D human mesh recovery in densely populated scenes.
更多查看译文
关键词
Human in Occlusion,3D Human Mesh Recovery,Image Blending,De-occlusion
AI 理解论文
溯源树
样例
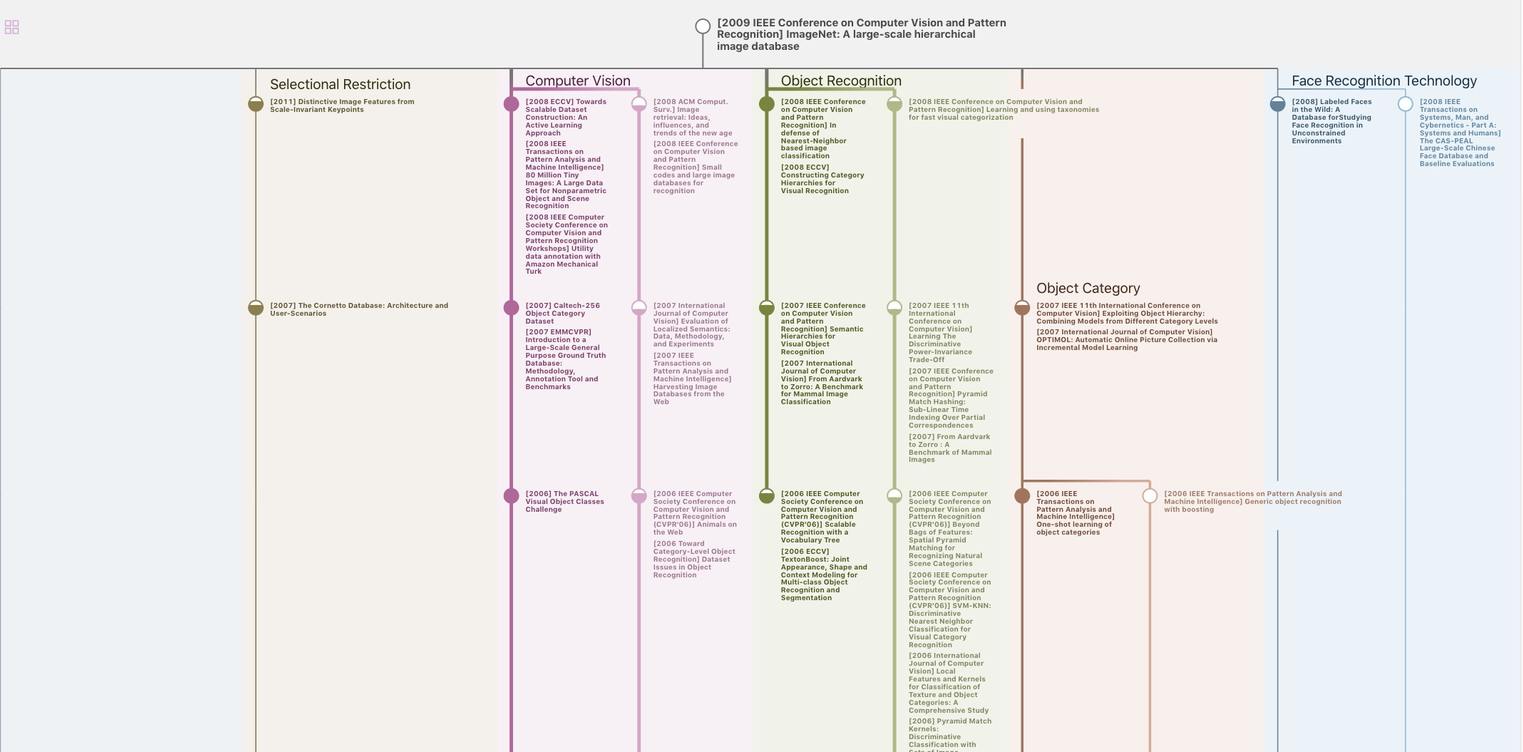
生成溯源树,研究论文发展脉络
Chat Paper
正在生成论文摘要