Optimizing Seasonal-To-Decadal Analog Forecasts With a Learned Spatially-Weighted Mask
Authorea (Authorea)(2023)
摘要
Seasonal-to-decadal climate prediction is crucial for decision-making in a number of industries, but forecasts on these timescales have limited skill. Here, we develop a data-driven method for selecting optimal analogs for seasonal-to-decadal analog forecasting. Using an interpretable neural network, we learn a spatially-weighted mask that quantifies how important each grid point is for determining whether two climate states will evolve similarly. We show that analogs selected using this weighted mask provide more skillful forecasts than analogs that are selected using traditional spatially-uniform methods. This method is tested on two prediction problems using the Max Planck Institute for Meteorology Grand Ensemble: multi-year prediction of North Atlantic sea surface temperatures, and seasonal prediction of El Nino Southern Oscillation. This work demonstrates a methodical approach to selecting analogs that may be useful for improving seasonal-to-decadal forecasts and understanding their sources of skill. Understanding how the climate will look in one to 10 years is useful for many industries, but this task is very difficult. One method for making forecasts on these timescales is called analog forecasting. In analog forecasting, a researcher finds past states in observations, or states in a climate model simulation, that look like the current state of the climate, and uses how those maps changed over time to predict how the climate will change over time. Some regions are more important for determining how a climate state will change over time, and we use a machine learning method called a neural network to identify these important regions. We find that if we only look at these important regions when determining if two climate states are similar or not, we can improve our analog forecasting skill. An interpretable neural network provides a spatially-weighted mask for selecting optimal analogsAnalogs selected with the weighted mask offer more skillful forecasts than traditional methods for selecting analogsThe learned mask highlights precursor regions for predicting large-scale climate anomalies in a perfect model framework
更多查看译文
关键词
interpretable machine learning,analog forecasting,seasonal-to-decadal climate prediction,El Nino Southern Oscillation,North Atlantic,sources of predictability
AI 理解论文
溯源树
样例
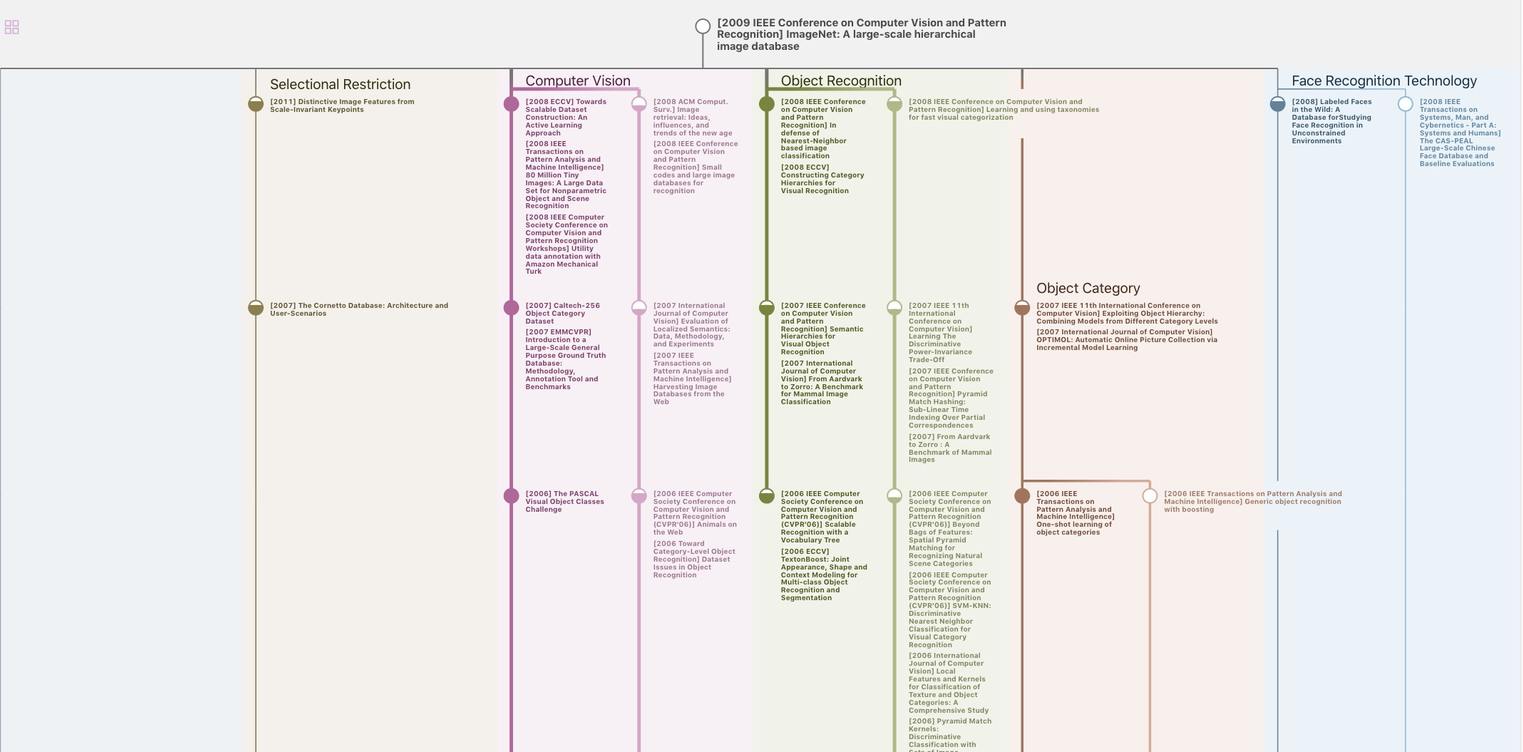
生成溯源树,研究论文发展脉络
Chat Paper
正在生成论文摘要