PoShapley-BCFL: A Fair and Robust Decentralized Federated Learning Based on Blockchain and the Proof of Shapley-Value
NEURAL INFORMATION PROCESSING, ICONIP 2023, PT II(2024)
摘要
Recently, blockchain-based Federated learning (BCFL) has emerged as a promising technology for promoting data sharing in the Internet of Things (IoT) without relying on a central authority, while ensuring data privacy, security, and traceability. However, it remains challenging to design an decentralized and appropriate incentive scheme that should promise a fair and efficient contribution evaluation for participants while defending against low-quality data attacks. Although Shapley-Value (SV) methods have been widely adopted in FL due to their ability to quantify individuals' contributions, they rely on a central server for calculation and incur high computational costs, making it impractical for decentralized and large-scale BCFL scenarios. In this paper, we designed and evaluated PoShapley-BCFL, a new blockchainbased FL approach to accommodate both contribution evaluation and defense against inferior data attacks. Specifically, we proposed PoShapley, a Shapley-value-enabled blockchain consensus protocol tailored to support a fair and efficient contribution assessment in PoShapley-BCFL. It mimics the Proof-of-Work mechanism that allows all participants to compute contributions in parallel based on an improved lightweight SV approach. Following using the PoShapley protocol, we further designed a fair-robust aggregation rule to improve the robustness of PoShapley-BCFL when facing inferior data attacks. Extensive experimental results validate the accuracy and efficiency of PoShapley in terms of distance and time cost, and also demonstrate the robustness of our designed PoShapley-BCFL.
更多查看译文
关键词
Blockchain,Proof of Shapley,Federated learning,Contribution Evaluation
AI 理解论文
溯源树
样例
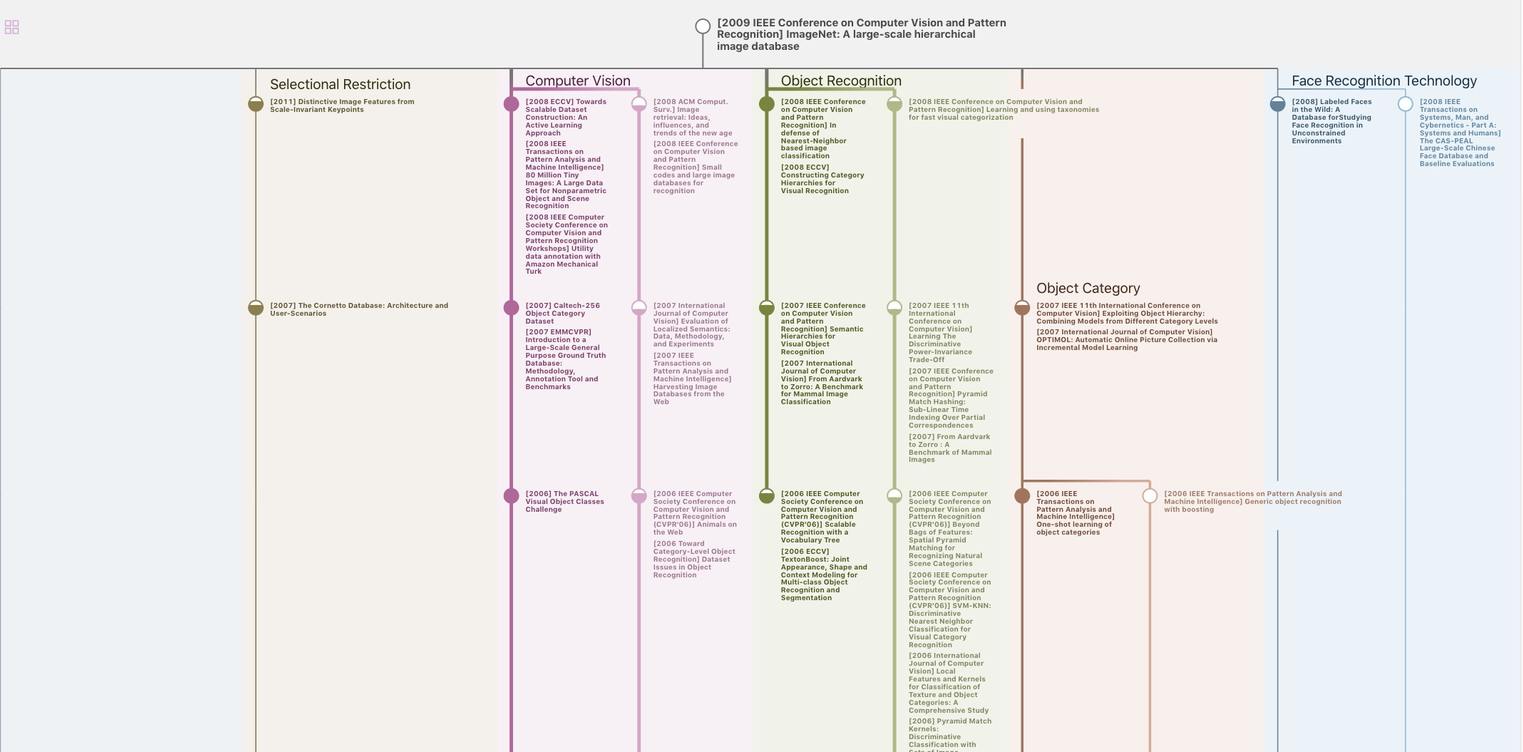
生成溯源树,研究论文发展脉络
Chat Paper
正在生成论文摘要