Towards a Unified Understanding of Uncertainty Quantification in Traffic Flow Forecasting
IEEE Transactions on Knowledge and Data Engineering(2023)
摘要
Uncertainty is an essential consideration for time series forecasting tasks. In this work, we focus on quantifying the uncertainty of traffic forecasting from a unified perspective. We develop a novel traffic forecasting framework, namely Deep Spatio-Temporal Uncertainty Quantification (DeepSTUQ), which can estimate both aleatoric and epistemic uncertainty. Specifically, we first leverage a spatio-temporal model to model the complex spatio-temporal correlations of traffic data. Subsequently, two independent sub-neural networks maximizing the heterogeneous log-likelihood are developed to estimate aleatoric uncertainty. To estimate epistemic uncertainty, we combine the merits of variational inference and deep ensembling by integrating the Monte Carlo dropout and the Adaptive Weight Averaging re-training methods, respectively. Furthermore, to relax the Gaussianity assumption, mitigate overfitting, and improve horizon-wise uncertainty quantification performance, we define a new calibration method called Multi-horizon Conformal Calibration (MHCC). Finally, we provide a theoretical analysis of the proposed unified approach based on the PAC-Bayes theory. Extensive experiments are conducted on four public datasets, and the empirical results suggest that the proposed method outperforms state-of-the-art methods in terms of both point prediction and uncertainty quantification.
更多查看译文
关键词
Deep ensembling,model calibration,PAC- bayes,traffic forecasting,uncertainty quantification,variational inference
AI 理解论文
溯源树
样例
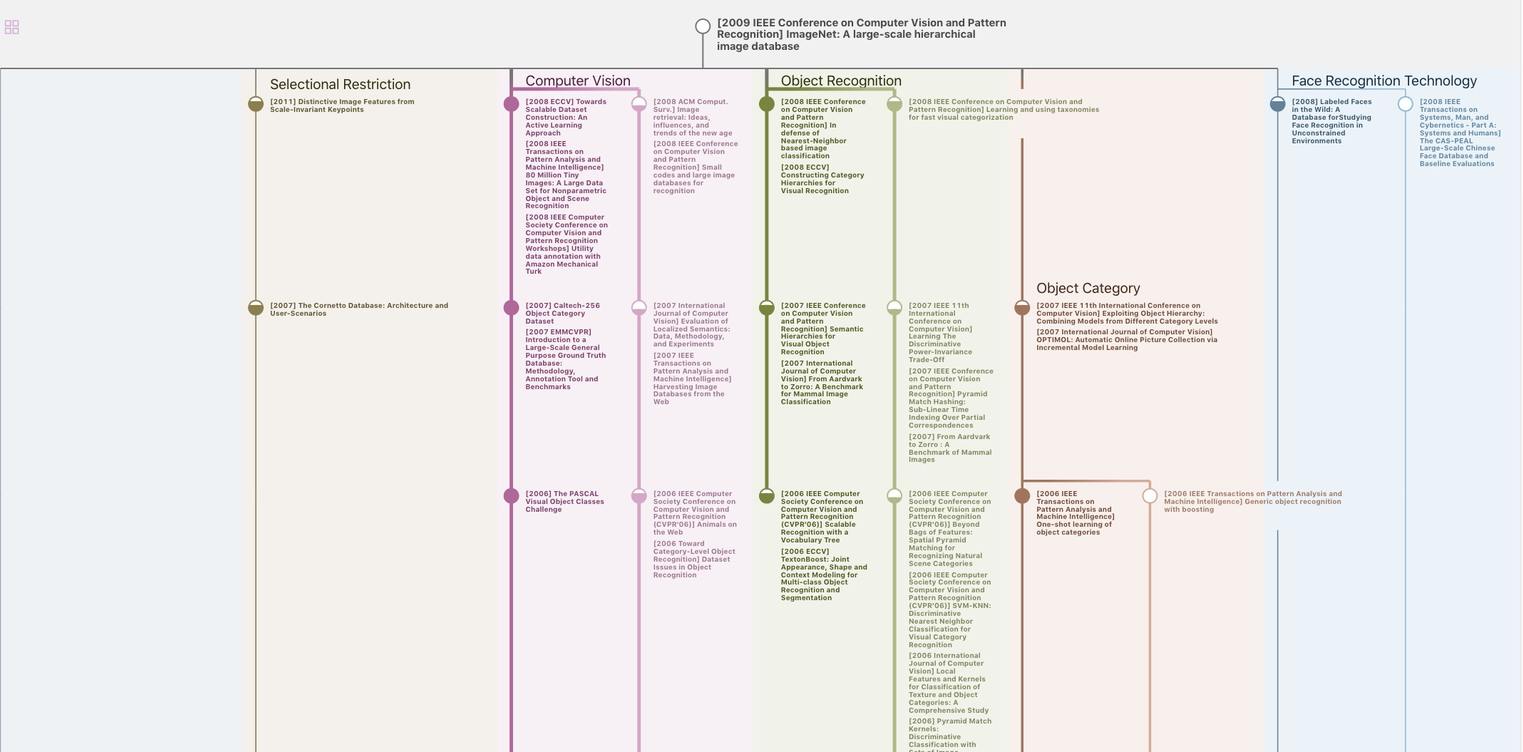
生成溯源树,研究论文发展脉络
Chat Paper
正在生成论文摘要